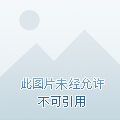
"We are confident that the effect of urban NGP rolled out within this year will be considerably better than that of FSD." On March 26, He Xiaopeng made it clear to electric vehicle observers in an interview at the 100 Forum that Xiaopeng Automobile will begin to transition to unmanned driving in 2026.
At this time, it has just been 16 months since He Xiaopeng called Tesla founder Musk that "(to) fight in China's autopilot field (Tesla) can't find the east".
He Xiaopeng speaks on social media in 2020
Of course, Tesla is not idle.
In April, Musk said that a beta version of the FSD that can achieve "full self-driving capability" has been installed on more than 100,000 Tesla models. The recently released Tesla 2021 Impact Report said that all cars in the United States are 8 times more likely to have accidents than Tesla cars that use Autopilot auto-assisted driving. Musk said the data will tend to be more than 10 times.
But in China, because there are too few open functions, expensive FSDs are far more important to Tesla owners than the use value of brand labels.
At the 2020 Xiaopeng Technology Day, in a comparative experiment of the Xiaopeng NPG/Tesla NoA navigation pilot driver assistance system, the Xiaopeng P7 performed stably, and the Tesla Model3 had a series of unprovoked, illegal lane changes and wrong driving out.
This performance is also consistent with repeated comparative test results in chinese media: Tesla, which began testing fully autonomous driving in North America, is still unsatisfactory on China's structured roads.
Xiaopeng, who started seven years later, can his current self-driving ability compete with Tesla's? He Xiaopeng said that he wanted to catch up with Tesla, was he bragging?
More importantly, the two major technical routes of pure visual and perceptual integration represented by Tesla and Xiaopeng, what is the difference under the goal of mass production of automatic driving, and what is the prospect?
01
In China, where is Tesla "worse" than Xiaopeng?
In essence, today's pure visual perception tesla models and the Xiaopeng models that rely on multi-sensor fusion are two "organisms" with very different operating modes, and the "habitat" is also very different.
1.1 | Pure vision VS multi-sensor fusion
Tesla FSD relies entirely on "seeing." Eight cameras around the body, at a rate of 36 frames per second each, with a resolution of 1280×960, 12-Bit RAW format images.
The raw image data is directly fed into a single pure visual neural network algorithm called "HydraNets", which performs image stitching, object classification, target tracking, online calibration of time series introduction, visual SLAM (positioning and map construction) and a series of others that allow the machine to understand "what I photographed", and finally form a "vector space" of road conditions space-time - a virtual mapping of the real physical world.
"The most difficult thing is to build an accurate vector space," Musk said, "and once you have an accurate vector space, the control problem is similar to a video game." ”
Vector Space, Tesla AI DAY August 2021
"Vector space" is a necessary condition for all L3 and above advanced driver assistance systems, the difference is how to obtain (perceive) real-world data.
Starting from P7, XPILOT Intelligent Driver Assistance System (hereinafter referred to as XPILOT) has formed a "Xiaopeng style" fusion perception system: front-looking tri-eye camera + fender side rearview camera + reflector front-view camera + rearview camera + five millimeter wave radar + four surround view cameras + twelve ultrasonic radar + high-precision map + high-precision positioning.
Starting with the P5, XPILOT introduced lidar.
Xiaopeng P5 will be applicable to urban NGP
Radar provides direct speed, depth, distance, and partial material information, with lidar directly virtually modeling 3D point clouds on real-world scenes, and cameras sensing multiple details such as pedestrians and traffic sign markings. After that, through the fusion algorithm mode, the raw data or perception results of different sensors are fused in 4D consistently, thereby establishing a vector space.
Both sets of options have their own advantages and disadvantages.
Vision solutions have great cost advantages. The cost of a single-eye camera is only between 150-600 yuan, and the cost of a more complex three-eye camera is usually within 1000 yuan.
Tesla's eight cameras cost less than $200 (1,400 yuan), plus self-developed autopilot chips, for a total cost of less than 10,000 yuan.
In addition to the camera, the cost of millimeter-wave radar is around $50, semi-solid-state lidar is generally in the hundreds of dollars, and the cost of high-precision maps.
In 2019, AutoNavi announced a standardized high-precision map cooperation price of 100 yuan / car / year. However, the Head Leopard Research Institute believes in the report that in addition to basic services, high-precision map dealers also charge auxiliary automatic driving service fees, and the industry price may be 700-800 yuan / car / year.
Cost is a decisive factor in the scale of mass production of a technology, but the reliability and achievability of the technology are even more important.
Distance/depth/speed detection is one of the disadvantages of the visual scheme, to build a vector space of 3D + time through 2D images, there is not only a delay problem caused by 2D "translation" to 3D, but also a very high requirement for image processing algorithms, the number /quality of scenes used in AI learning, and hardware computing power.
For example, after Tesla eliminated millimeter-wave radar last year, the FSD beta's auto-steering feature set a top speed of 75 mph (120 km/h) and a minimum of three vehicles. Over the next two months, Tesla raised the speed limit to 80 mph (128 km/h) and lowered the follow-up distance to two body lengths.
The multi-sensor solution has distance/depth/speed data provided directly by radar, as well as a priori information beyond the line of sight provided by HIGH-precision maps, and decimeter or even centimeter-level positioning capabilities provided by the high-precision positioning module.
"[Thus] helps the AI understand, decide, and plan next actions, providing an auxiliary and redundant source of information for perception based on other sensors." Wu Xinzhou, vice president of autonomous driving at Xiaopeng Automobile, told Electric Vehicle Observer.
Each level of autonomous driving corresponds to the number of pixels, data quantity and computing power requirements (estimated value) "CICC | AI Ten-Year Outlook》
Getting enough redundancy is the main reason why companies such as L4-level autonomous driving companies and Xiaopeng have chosen multi-sensor fusion instead of pure vision routes.
At present, because pure vision does not have the ability to directly measure speed and acceleration, ghost braking will be a long-term problem that is difficult to cure.
In the future, for the expected functional safety and functional safety required by high-end automatic driving systems, it is necessary to prevent the failure of a single system and narrow the expected redundancy of failure, "At present, it is difficult for pure vision systems to meet the safety requirements required for high-level automatic driving." An expert in the field of autonomous driving told The Electric Vehicle Observer.
1.2 Tesla in the United States and Xiaopeng in China
The difference in "habitat" further increases the landing performance of the two technical routes.
The complexity of China's traffic environment far exceeds that of the United States, and a large amount of auxiliary information outside the visual distance is required to provide to the decision-making system in order to successfully complete the passage, which also leads to relying only on real-time, pure visual perception systems, which is difficult to land in China.
The most complex overpass system in China and the United States: Atlanta, USA (left) and Chongqing, China
For example, even if the closed highway is a simple scene, China has more curves and greater curvature than the United States road, and even has two overlapping sections, and the route that can be "seen at a glance" is very short; China's highway has longer entry and exit ramps, and more frequently changing virtual lane lines, and even pedestrians who should not appear on the closed road.
Some companies have found in practice that due to the difference in the degree of traffic participants' traffic norms, the automatic driving system is nearly 10 times easier than in China at intersections.
Without the use of high-precision maps, the lack of prior information, and complete reliance on visual perception, neural networks account for more than 98% of FSDs, which require massive amounts of high-quality, differential data to evolve.
Therefore, under the "feeding" of North American data, the FSD beta version has achieved partial self-driving capabilities in unstructured road sections, but Tesla has not been able to smoothly run through the high-speed road sections in China – it currently lacks the ability to use Chinese scene data.
Due to national data security requirements, Tesla data in China can not "go abroad", which not only means that the data itself must be stored in Chinese servers, foreign IP can not be accessed through the network, even those who read data in China have strict nationality background restrictions.
This means that Tesla needs to "rebuild" the organization in China to adapt to the Chinese scene.
The first is the data and R&D center in China. "Responsible for data collection and model training, as well as a series of supporting organizations such as product managers, teams of more than 100 people." A big data engineer of the new car manufacturing force told the Electric Vehicle Observer.
You also need to rebuild the workflow. Because U.S. data cannot enter China, it can only pass model parameters from the United States, but not the data itself, "which will have a great impact on the training of the model, and it is necessary to re-build the pipeline for training in China (data pipeline: data collection, processing, desensitization, cleaning, labeling, classification and training process). The big data engineer said that this in turn means hundreds or even thousands of process teams.
"Electric Vehicle Observer" learned in the interview that in the second half of 2021, Tesla has begun to recruit relevant personnel for self-driving research and development in China, but the scale and use are still unknown.
And, like all multinational organizations, overseas affiliates are never just a matter of money and people.
"Even with all the R&D imports, the integration of Tesla's R&D teams in China and the United States is not necessarily so smooth." Zhu Chen, general manager of the Internet of Things business line of Thoughtworks, told The Electric Vehicle Observer that the most painful part of international R&D institutions is that the ideas of branches and headquarters are different. For example, China's R&D team makes some specific judgments according to China's national conditions, and whether it is willing to approve after submitting it to the headquarters. "Whose code is used, and the series of questions that arise from it. Xiaopeng does not need to worry about these problems. ”
At the beginning of the birth of XPILOT, it served the Chinese scene.
Xiaopeng adopts the decision-making logic based on high-precision maps, uses high-precision maps and multi-sensor fusion, and realizes the landing of high-level intelligent driving assistance capabilities of high-speed navigation and navigation with relatively low difficulty of perception and decision-making algorithms.
Moreover, the Chinese team can also be specially optimized for local scenes, thus surpassing tesla NoA's performance in China on the experience side.
It is reported that Xiaopeng has optimized the perception aspect of identifying "Chinese characteristics" scenes such as gas plugs and large trucks: adjusting the layout and perception range of the sensor; importing more targeted scenes to the XP perception model for training.
In view of the disadvantage of insufficient "freshness" of the HIGH-precision map, Xiaopeng has enhanced the map system: the new road conditions that are visually perceived to be inconsistent with the high-precision map are modeled and supplemented into the map; the accuracy of the high-precision map is improved by the enhancement algorithm to better adapt to the scene where the road is undulating; the details that are not taken by the high-precision map are completed through technology, and so on.
It's worth noting that enhancing HD maps isn't just a technical issue.
In 2021, Xiaopeng invested 250 million yuan to acquire Jiangsu Zhitu Technology Co., Ltd., obtaining a scarce Grade A map qualification. Not only did it make the "completion" map justified, but it also got the entrance ticket to the self-built high-precision map.
Xiaopeng is also the first new Chinese car-making force to obtain this qualification.
02
Algorithmic divergence
"Every big change in hardware will also bring about a big change in software algorithms." Horizon founder Yu Kai said in his speech.
The difference brought about by the perception hardware solution is the appearance of the "difference" between Xiaopeng and Tesla at this stage, and the deeper difference comes from the difference in the "thinking mode" behind the different perception routes - in the far future to determine whether the goal of mass production of automatic driving can finally land.
The "mindset" is the software algorithm of the autonomous driving system. It is mainly divided into three parts: perception, decision-making and control.
The perception algorithm pursues to solve the problem of what the sensor "feels" is, and finally establishes a vector space highly similar to the actual road conditions at the end of the car by classifying, labeling, and understanding the perceived objects;
The decision-making algorithm needs to comprehensively consider the navigation route, road conditions, the action intentions of other traffic participants, as well as driving standards such as safety, efficiency, and comfort, and first solve the feasible space (convex space) in the vector space, and then use the optimization method to optimize the solution in the feasible space and output the final trajectory.
The control section is responsible for efficiently coordinating the individual actuators of the chassis system in order to faithfully execute the "decisions" of the decision algorithm.
"Electric Vehicle Observer" learned in the interview that in the current high-end driving assistance and automatic driving systems, the vast majority of perception algorithms have used AI neural networks for perception, and the decision-making algorithms have also used neural networks for search and option convergence on the front end, and logical judgment algorithms have been used on the back end.
So, behind the hardware solutions of pure vision and multiple sensors, how big is the divergence of software algorithms?
2.1 | Perceptual algorithm comparison
The AI model of perception algorithms using neural networks is the mainstream model at present.
Back in Time to August 2020, Musk said for the first time that Tesla was rewriting the infrastructure of FSD. A year later, at AI DAY, Tesla announced that the proportion of COMPUTation of CNN convolutional neural networks in the perceptual algorithm model reached 98%, and time series were added through RNNs (recurrent neural networks). Blend disparate camera data by leveraging Transformer with excellent algorithmic parallelism.
Intuitively, the raw data of the 8 cameras on the Tesla car enters the perceptual algorithm model, and the model output is a consistent result of time and space. Musk said in a recent interview that Tesla has completed a complete mapping from vision to vector space.
In the information currently released, Tesla's perception algorithm model contains at least 48 specific neural network structures, which can perform more than 1,000 different recognition and prediction tasks at the same time, and the cycle required for a full training is 70,000 GPU hours.
In contrast, Xiaopeng, which adopts multi-sensor fusion, has to take another step on the basis of completing the visual perception algorithm.
At present, the Xiaopeng P5 is equipped with a sensor solution composed of camera, millimeter wave radar, ultrasonic radar, lidar and high-precision map. Among them, the perception algorithm of radar is relatively simple, and the high-precision map can provide transcendental information in time-space.
Multi-sensor data fusion process, CICC | AI Ten-Year Outlook》
The real difficulty lies in the integration of vision, radar and high-precision map information through algorithmic models to establish a vector space.
Because the detection frequency, information type and accuracy of different sensors are different, the fusion algorithm model receives sensor information with inconsistent time, inconsistent information, and even inconsistent "appearance", and it is difficult to integrate it into a vector space with consistency in time and space.
Moreover, compared with the pure visual algorithm that relies only on "seeing" and information consistency, the multi-sensor plus high-precision map scheme still has the multiple choice question of "who to believe" - the "confidence" problem.
Some experts told the Electric Vehicle Observer that the "confidence" problem of the perception fusion system is currently mainly verified by third-party data in simulation and real road conditions.
The "confidence" problem that Xiaopeng deals with is not a generalization. In the high-speed NGP stage, Xiaopeng adopts the strategy of high-precision maps, and enters the urban NGP stage, and will adopt a visual perception-based solution.
"In the urban NGP, high-precision maps are still very important inputs. However, due to the existence of lidar and the rapid improvement of visual perception capabilities, we can handle various scenes more safely and naturally, and we can have more strong fault tolerance when the boundaries of the map or the data are errors and omissions. Wu Xinzhou told the Electric Vehicle Observer, "(With the construction of system capabilities), we are confident of catching up with or even surpassing Tesla's visual capabilities." ”
2.2 | The "easy" of pure vision and the "difficulty" of multi-sensor fusion
Catching up with Tesla in terms of visual ability is not a big sentence from a theoretical point of view.
Visual perception neural networks based on image recognition have a "long" history, and have accumulated many concise and efficient open source algorithms.
This is the reason why Tesla dares to disclose the logic of its perception algorithm model, and it has also become the basis for Xiaopeng to catch up with or even surpass Tesla in terms of visual ability.
From the current results, XPILOT and FSD are the only car companies that currently produce side -- and-column (A-pillar) viewing cameras in the mass-produced autonomous driving system. The reason is that the algorithm that stitches and integrates the side image with the image of the wide-angle front-view camera has a high threshold, especially on the mass production model.
It is especially important to do a good job of visual perception algorithms. Experts interviewed by The Electric Vehicle Observer generally believe that visual perception will continue to be the core perception solution of future autonomous driving systems.
But why do you need to do a multi-sensor fusion route? At the heart of it is the ultimate pursuit of responsiveness and safety redundancy.
As camera capabilities continue to improve, visual perception continues to advance in its ability to cope with harsh weather and road conditions. But because there is always a 2D to 3D "translation" process, and the resulting delay of about 1 second, this is sometimes fatal for a moving car.
Tesla has now removed the image pre-processing (LSP) function of the camera to adapt to human eye viewing through the underlying software rewriting and system integration, and directly transmitted the original information to the model, thereby reducing the latency of 8 cameras for a total of 13 milliseconds.
Radar can directly give distance/depth/speed information, and the data of multiple sensors can "check and fill in the gaps" with each other.
After forming its own perception architecture on the P7, Xiaopeng applied lidar on the P5 and replaced the previous front-line tri-eye camera with a binocular camera on the G9 - 1 narrow vision + 1 fisheye.
"(With) XPILOT 4.0's stronger capabilities and increasing requirements for camera resolution, the camera is the next generation to achieve higher resolutions in the context of the current trinocular camera resolution that cannot meet the demand." Wu Xinzhou explained this.
The problem is that there are currently fewer open-source algorithms for multi-sensor fusion "on the market".
Therefore, taking the multi-sensor fusion route, its fusion algorithm will rely more on self-research, verification and iteration of each company, during which different styles will be formed, but it also lacks the advantages of visual perception "accelerating the world in multiple fields together".
Moreover, the current multi-sensor fusion route will lead to a strong binding between car companies and suppliers.
Unlike cameras, which have standard data formats and common data interfaces, radar and HIGH-precision maps are still "non-standard". LiDAR also has a mechanical, solid-state, semi-solid route dispute, data format and interface has not yet formed a unified standard in the industry. High-precision maps also differ in data calibration methods and accuracy due to different map dealers.
Therefore, although car companies generally pursue soft and hard decoupling, in fact, in some sensor fields with particularity, changing suppliers means changing the algorithm model. It has also led to car companies with multi-sensor fusion routes being more cautious in the selection of suppliers, not only establishing procurement relationships, but even many have established in-depth cooperative relations of investment and joint research and development.
2.3 | What's harder is the decision algorithm
Solving the "what feels" and building a vector space is just the beginning.
Ai technology continues to grow in perception with the blessing of deep learning, but it still lacks the ability to "think": the ability to deal with complex relationships such as conditional probability, cause and effect, and complete the task of reasoning and inference.
Such a capability, in the process of landing on automatic driving, is a matter of life and death.
In 2018, Uber's test vehicles had the world's first fatal accident. According to the official US report, the vehicle observed the "obstacle" 6 seconds before the accident, and in the first 1.3 seconds, it was judged that it was a bicycle and needed to take emergency braking. However, "in order to reduce the possibility of unstable behavior (lack of comfort) in the car", the automatic emergency brake did not start, and the slow braking was taken, coupled with the distraction of the safety officer, which eventually led to the accident.
This case illustrates the importance of decision-making systems, especially in urban conditions with complex road conditions and game scenarios.
Cruise, GM's L4-level self-driving technology company, defined a good decision-making system at last year's technology day: timeliness; interactive decision-making (considering the future actions and impacts of other traffic participants and vehicles); reliability and repeatability (being able to make the same decisions in the same scenario), thereby outputting a safe, efficient, and old-driver-like ride experience.
Tesla clarified at the previous AI DAY that the criteria of its decision-making system are safety, comfort and efficiency;
Wu Xinzhou introduced to the Electric Vehicle Observer that the decision-making elements of XPILOT in more difficult urban scenarios are: safety, availability, and ease of use.
The standards are similar, but it is not easy to achieve the same handling performance as the old driver.
In low-speed or simple scenarios, the decision-making algorithm draws a collision-free safe path based on the perceptual data, and the vehicle moves along the specified route.
However, in complex traffic flow and scene road conditions, there are often problems such as planning trajectory jumps and collisions. The core is due to the lack of predictiveness of the decision algorithm for the future behavior of obstacles, and the algorithm only relies on the perception data of the current moment to solve the local, rather than the global, road condition.
Therefore, when the vehicle is in an unfamiliar and complex scene, it often repeatedly brakes or dangerous actions, and the decision-making criteria of "safety, efficiency and comfort" are difficult to meet.
When the vehicle is autonomously driving, there may be hundreds of traffic participants in a traffic scene interacting with the autonomous vehicle, and the decision-making system needs to consider the future actions of other traffic participants in the scene, project the various behaviors of the predicted social vehicle, line into a drivable space, and then search out the trajectory.
Among them, prediction is considered to be the most difficult part of the landing of automatic driving system engineering. Vehicles must not only understand the various possible future movements of themselves and the environment, but also judge the most likely traffic participant behaviors from countless possibilities.
In order to establish the predictive ability of the system, in addition to continuously optimizing the algorithm, the industry currently needs to self-supervise the learning of THE AI in the world model. The massive traffic participant behavior collected by Tesla through shadow mode in the real world has become the best teaching material for FSD to build prediction capabilities.
At last year's AI DAY, Tesla showed a narrow road car scene. The self-driving vehicle initially thought that his car would continue to drive, so it waited on the right, found that his car had also stopped and gave way, and immediately moved forward.
Tesla self-driving car narrow lane traffic case, AI DAY
In this regard, an autonomous driving control engineer told the "Electric Vehicle Observer" that most autonomous driving companies cannot handle such a scene at present, and often conservatively choose to stop and give way, or stop with other cars, resulting in a collision risk. "But Tesla can handle the scenario very well, proving that its predictions and decisions work very well together."
Even with "prediction", "search" is not easy.
Autonomous vehicles typically need to sample and calculate more than 5,000 alternate trajectories to make the right decisions.
But "time is not equal", decision planning algorithms usually run at a frequency of about 10Hz-30Hz, that is, every 30ms to 100ms need to be calculated once, and making the right decision in such a short period of time is a huge challenge.
Tesla FSD can search 2500 times in 1.5ms, and the optimal trajectory is selected after a comprehensive evaluation of the alternative trajectory.
However, such an approach often leads to supercomputing (beyond the computing power of the computing platform) in urban road conditions with mixed flow of people and vehicles and complex road structures.
To this end, Tesla introduced the MCTS framework (Monte Carlo tree search), which is more than 100 times more efficient than traditional search methods.
MCTS can be more effective in solving some problems with huge exploration space, such as the general Go algorithm is based on MCTS implementation. Both Apple's autopilot patent and Google's Alpha Dog have adopted the method.
Xiaopeng XPILOT road interaction
At present, Xiaopeng has not disclosed the type of model used by its decision-making algorithm. However, Wu Xinzhou told the Electric Vehicle Observer that in the urban scene, due to the different traffic participants and the complexity of the scene, there are completely different requirements for prediction, planning and control. Therefore, Xiaopeng's ability to locate, perceive, and integrate is greatly enhanced on the basis of high-speed scenes.
"For the decision-making part, we introduced a completely new architecture to meet the higher requirements of urban NGPs. This part of the architecture also has a very strong reverse compatibility, so we also look forward to the future in XPILOT 3.5, our high-speed and parking lot scenarios can also benefit from this new architecture, to give users a better experience. ”
03
How Xiaopeng is catching up with Tesla around the world
Tesla FSD opens in China sooner or later, and Xiaopeng Motors intelligent driving also has to go out of China. Sooner or later, the two sides will face each other. Can Xiaopeng fight Tesla in the Eastern Hemisphere and even the world?
What really gives He Xiaopeng confidence in Tesla is the end-to-end full-stack self-developed system capability completed by Xiaopeng Automobile from 2020.
3.1 | Build your own algorithmic data closed loop
What is full-stack self-development?
Wu Xinzhou told the Electric Vehicle Observer that Xiaopeng Automobile's "full-stack self-research" is not only the self-developed visual perception, sensor fusion, positioning, planning, decision-making, control and other aspects of the car side.
It also includes a range of tools and processes needed to operate data in the cloud.
That is, data upload channel, front-end data upload implementation, cloud data management system, distributed network training, data collection tool development, data annotation tool development, software deployment and other aspects to achieve self-research.
"This forms a fully closed loop of data and algorithms, laying a solid technical foundation for rapid function iteration."
Different from the logical judgment algorithm model depends on how smart the engineer is, the neural network algorithm model mainly used in the automatic driving system has the characteristics of "data growth": the algorithm is gradually matured in the data flow formed by the early data collection, intermediate data storage and migration, and the training and management of the core data in the later stage.
The algorithm is iterated by data, and the iterative algorithm brings new data, and the improvement of system capabilities is essentially a cyclical process in the data.
In this growth closed loop, any link will affect the speed and quality of the iterative upgrading of the company's "own" autonomous driving system.
Previously, there was no "own" rhythm in the traditional automobile industry. Although the main engine factory occupies a strong position in the industrial chain, the model iteration cycle is more limited by the technical and commercial rhythm of parts suppliers. Until Tesla broke this industry convention.
Tesla AP Change History Data Collation: Electric Vehicle Observer
In the June 2016 Model S death, the disagreement over when to achieve AEB (emergency braking) through the visual scheme led to a complete "breakup" between Tesla and Mobileye.
In response to the accident vehicle not activating the Emergency Braking (AEB) function, Mobileye Chief Communications Officer Dan Galves issued a statement saying: "At present (2016) AEB is classified as a rear-end accident avoidance system, (and therefore unable to cope with vehicles that appear laterally in front). However, Mobileye will bring road lateral turn (LTAP) detection from 2018. ”
But Tesla is not willing to wait until 2018, nor is it willing to follow the traditional visual perception route that Mobileye is good at.
As a result, Autopilot Vision (TV), Tesla's visual perception self-developed software algorithm group, which has only been established for a year, "forcibly" replaced Mobileye's position in October 2016 and determined the technical route of AI visual perception at the end of the year.
At the beginning of the launch, TV did not complete all the application development of the AP software terminal, including AEB, anti-collision warning, lane keeping, adaptive cruise and other key functions missing for several months, and also used millimeter wave radar to do AEB's "work" for a period of time, resulting in many "ghost brake" cases.
Until April 2017, Tesla pushed V8.1, and the self-developed AI visual algorithm ability caught up with the HW1.0 era supported by Mobileye, which opened up the iteration speed that the automotive industry had never had, and "forced" the entire industry to "catch up" with Tesla's rhythm.
Xiaopeng is the first car company in the industry to catch up with Tesla's rhythm from the full stack, rather than just the functional level.
In 2018, through the Xiaopeng G3, XPILOT 2.0 was officially productized, and the end-to-end self-developed data closed-loop automatic parking system was mass-produced;
In 2019, the XPILOT 2.5 system installed on the Xiaopeng G3i realized the ALC automatic lane change function in addition to parking. Among them, Xiaopeng independently developed the lowest-level line control, path planning and control part of the algorithm, and the perception algorithm still depends on the supplier to provide;
In 2020, Xiaopeng P7 and XPILOT 3.0 appeared together, which can realize NGP and parking lot memory parking functions, so that Xiaopeng completed the in-depth software full-stack self-research for the first time, established its own visual perception ability, data closed-loop system that drives perception evolution, high-level assisted driving algorithm and software architecture, and became the second car company in the world to realize the full-stack self-research of automatic driving system, algorithm and data closed-loop.
"Compared with non-self-research, the use of the 'full-stack self-research' model is definitely heavier in terms of organization, talent, and R&D investment, but the advantages are also obvious." Wu Xinzhou said.
3.2 | Nuggets full stack self-research
The advantages are indeed obvious.
Only from the perspective of high-speed navigation pilot function, Xiaopeng NGP landed in 2020; although Weilai was slightly earlier, it was still based on Mobileye's semi-self-developed products; ideally, it landed the function after upgrading in September 2021.
Brands including Extreme Kr + Mobileye (ZAD), Pole Fox Huawei HI Edition (Huawei ADS), Zhiji Automobile + Momenta (IM AD), Nezha Automobile (Huawei + Horizon), Zero Run Car (Leap Pilot) and other brands have plans for L3-level high-end intelligent driving capabilities, but there is still a considerable time difference with Xiaopeng.
Compared with "himself", Xiaopeng has also made rapid progress.
Xiaopeng XPILOT system change Information: "Electric Vehicle Observer"
In 2020, in addition to the self-developed solution, the Xiaopeng P7 also uses a front-facing camera and an intelligent controller based on Infineon Aurix MCU 2.0 as a redundancy scheme, and its perception and decision-making algorithms are also from Bosch. By 2021, the P5, this set of redundancy schemes from third parties has been eliminated, and the P5 will only be equipped with the NVIDIA Xavie platform, and lidar will be added as a sensor.
According to the plan, Xiaopeng will implement the most core urban NGP function of XP3.5 on the NVIDIA Xavie platform with a hashrate capacity of 30TOPS. Tesla's FSD chip, which also implements this high-end driver assistance function, has a computing power of 144TOPS.
"(Full stack self-development) exercised the team's ultimate engineering ability, and achieved relatively complex functions under limited computing power." Wu Xinzhou told Electric Vehicle Observer: "From XPILOT 3.0 to 3.5, and then to the future 4.0 and 5.0, Xiaopeng's technical route is a very continuous natural evolution. ”
3.3 | Efficiency competition
Different means to the same end. Although Xiaopeng has embarked on a technical route that is quite divergent from Tesla, the path and goal of the two are the same: through full-stack self-research, mass production of autonomous driving technology.
In Wu Xinzhou's view, the competition for this goal lies in the amount of data, and the other lies in the correct network architecture.
"Tesla's current network architecture has high requirements for system capabilities, whether from the perspective of data acquisition, labeling and training, other manufacturers have a huge gap with Tesla in the construction and investment of system capabilities."
In terms of data volume, Tesla is currently unbeatable in the world.
Tesla Artificial Intelligence Director Andrej said at CVPR (IEEE International Conference on Computer Vision and Pattern Recognition) 2021 that as of the end of June 2021, Tesla has a fleet of millions of people and has collected 1 million highly differentiated scene video data of 36 frames and 10 seconds, accounting for about 1.5 petabytes of storage space. 6 billion object annotations containing precise depth and acceleration were obtained, and a total of seven rounds of shadow mode iteration process were carried out.
This data scale is not only far from Tesla, but even a number of self-driving companies are difficult to match. In October last year, Waymo released the latest data, the cumulative road test mileage reached 10 million miles. Tesla's figures as of Last June were nearly 15 million miles, of which 1.7 million were collected at the startup of Autopilot.
Data is the fuel for the iterative growth of algorithm models of autonomous driving systems. Tesla has built an efficient data closed-loop system to process this massive amount of data into "anthracite coal".
The three stages of Tesla's data production are "CICC | AI Ten-Year Outlook》
On the basis of the million-vehicle fleet, Tesla collects massive corner case (rare and special long-tail scene) scene data through "shadow mode" and the operation data of human drivers in this scenario to provide higher-quality semi-supervised learning or supervised learning guidance for neural networks;
These raw data need to be labeled with various features before they can be used as learning material for neural networks.
Previously, this kind of unstructured data relied on a large number of manual labels, which was a labor-intensive industry, and companies outsourced their work to third parties. However, third-party labeling has pain points such as low efficiency and slow feedback, resulting in higher latency in labeling, analyzing, and processing training data.
Tesla has built a data annotation team of more than 1,000 people, divided into four teams of manual data annotation, automatic data annotation, simulation and data scale, and the technical level has completed the progress from 2D annotation to 4D annotation and automatic annotation, and the automatic annotation tool can realize the synchronous annotation of all cameras from multiple perspectives and multi-frame screens through one annotation, and can also be marked on the time dimension.
After building its own data labeling system, Tesla also built its own data training ground - a supercomputing cluster with a computing power of up to 1.1EFLOP composed of 3,000 Tesla's self-developed Dojo D1 chips, and the world's first computing power echelon with Google (1 EFLOP) and SenseTime (1.1 EFLOPS).
Moreover, compared with the universal supercomputing cluster of Google and SenseTime, Dojo focuses more on video processing in design, and the model training of Tesla's autopilot system is more targeted, which effectively reduces the cost of algorithms.
"We believe that the gap in the system is more important than the gap in the data, and Xiaopeng has been committed to building its own system capacity in the past few years." The results of complex system engineering in the terminal are not determined by a single variable, but also depend on the degree of matching of the overall design with the hardware.
In the future, we will continue to balance algorithm optimization and sensor selection or change, use suitable hardware to make higher-level driving assistance capabilities, and continue to evolve to automatic driving. Wu Xinzhou told the Electric Vehicle Observer.
3.4 | Xiao Peng's chance point
Efficiency and cost are decisive factors for the successful mass production of any product. Tesla's construction of this data closed-loop system of efficiency and cost reduction not only relies on its own technical capabilities, but also closely related to its strong financial strength.
In 2021, Tesla's research and development expenses will be about 16.8 billion yuan (2.591 billion US dollars, 6.5 yuan / US dollar). This compares to 4.114 billion yuan for Xiaopeng and 9.07 billion yuan for the Great Wall.
But that doesn't mean Xiaopeng has no chance of winning the second half of the race against Tesla's mass-produced autopilot.
Zhu Chen told the "Electric Vehicle Observer" that compared with Tesla's product ideas that are completely from the perspective of technology companies, Xiaopeng is more thinking about whether it can combine China's applicable scenarios and truly bring help to the life of car owners when launching product functions.
The functions that are more suitable for the needs of Chinese users will help Xiaopeng to scale up sales in China, so as to achieve the mass production of XPILOT in the true sense, and help it establish data and system advantages in The Chinese scene.
In 2020, the purchase rate of FSD (Tesla's fully automated driving system) in China is only 1-2%, which is lower than the proportion of 10%-15% in North America (calculated by foreign media). In Q4 2021, the FSD occupancy rate on the Model 3 model was 0.9% in the Asia-Pacific region, and 21.4% in Europe and 24.2% in North America. (Long-time Tesla blogger Troy Teslike statistics)
Xiaopeng XPILOT landing situation, Q3, 2021
As of the end of the third quarter of last year, the activation rate of THE XPIRAT 3.0, which is similar to tesla's Autopilot Enhanced, was nearly 60%. Wu Xinzhou did not disclose Xiaopeng's data acquisition mode, but said that "the world's advanced experience will be learned." ”
In terms of computing power and dojo, the "external force" that Xiaopeng can currently rely on is not weak at all.
The Xiaopeng G9 will be equipped with XPILOT 4.0, which will be equipped with the 508 TOPS NVIDIA Orin-X chip, and a highly integrated domain controller with Gigabit Ether. And NVIDIA released this year's AI training server EOS hashrate as high as 18.4 EFLOPS.
And Xiaopeng's ability to grasp the scene compared to Tesla has begun to appear.
In March this year, Xiaopeng Automobile pushed Xmart OS 3.1.0 version, realizing the 2-kilometer-long VPA-L cross-floor parking lot memory parking function. Almost at the same time, there are rumors that Tesla is developing a "Smart Park": under the premise of having a driver, the vehicle automatically stops at designated locations such as "the closest door", "near the shopping cart exit", "the end of the parking lot" and so on. In terms of functional description, Smart Park is very similar to memory parking, with the front runner and the chaser position inverted.
Farther overseas, just as Xiaopeng said earlier: "We will meet." ”
Acknowledgements for this article (in alphabetical order):
Abao 1900 autonomous driving expert
Diddy Autonomous Driving Data Engineer
Wu Xinzhou Xiaopeng Automobile Vice President of Autonomous Driving
Algorithm engineer for autonomous tractor decision planning
Chen Zhu, General Manager of thoughtworks IoT business line
Reference: CICC Software and Services | Ten-Year Outlook on Artificial Intelligence
——END——