Edit/Keich
With the rapid development of advanced engineering computing, economic data analysis, and cloud computing, the demand for ultra-high-speed and energy-efficient computing is growing exponentially. Conventional electronic signal processors under the existing von Neumann architecture are difficult to achieve both high speed and low energy consumption.
Using photons as information carriers is a promising option. Due to the weak third-order nonlinear optics of traditional materials, building integrated photonic computing chips under the traditional von Neumann architecture has been a challenge.
Recently, the research team of Gong Qihuang of the School of Physics of Peking University proposed a new strategy based on convolutional neural network (CNN) to achieve ultra-fast and ultra-low energy consumption all-optical computing chip solution to support the execution of multiple computing tasks. This work points the way for the next generation of all-optical computing systems.
The study, titled "All-optical computing based on convolutional neural networks," was published In Opto-Electronic Advances on November 25.
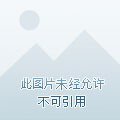
The transformation of electrons into photons
The existing computing instruments are mainly electronic processors, with electronics as the information carrier, with the von Neumann architecture, and the storage and processing are physically separated. The expansion of computing speed is limited not only by the data transfer between memory and processing units, but also by the RC latency associated with integrated circuits. In addition, overheating due to ohmic losses is becoming a serious bottleneck in speed and power scaling.
All-optical computation using photons as information carriers offers a promising alternative. Currently, optical computing typically relies on third-order nonlinear optics to achieve full-light control. However, ultrafast response times and large nonlinearities often present an inherent trade-off in optical materials, so greater nonlinear sensitivity can often only be obtained at the expense of slower response times.
This trade-off presents significant challenges for building integrated photonic processors that follow the von Neumann architecture, often requiring complex heterogeneous integration of various photonic devices in a single chip. Therefore, it is imperative to explore new architectures and unconventional computing solutions for all-optical computing.
Here, a new strategy for ultrafast, ultra-low energy all-optical computation is reported, including equation solving and CNN-based multifunctional logic operations.
The researchers said: This is the first CNN to be physically fixed on an all-optical chip.
Universal architecture of the all-optical computing framework.
Optical CNNs consist of cascaded silicon Y-shaped waveguides and side-coupled silicon waveguide segments for complete phase and amplitude control in each waveguide branch. This simple concept and architecture uniquely provides ultra-fast computing times and low energy consumption.
All-optical computing performance
General-purpose device concepts can be used for equation solving, multifunctional logic operations, and many other mathematical operations. Next, a variety of calculation functions including the transcendental equation solver, various logic gate operators, and half-adders were experimentally demonstrated to verify the performance of all-optical computing.
All-light transcendent equation solver
Equations are an effective tool for describing the state and processes of a system, and solving the equations can inform the state of the system under study and predict the trajectory of system evolution. Since the transcendent equation can only be solved numerically except in a few cases, numerical solution of the transcendent equation remains an important subject in mathematical calculations.
The team developed a solver that can predict the solution of the transcendent equation using optical CNNs with excellent computational performance.
All-light transcendent equation solver.
The test results show that the solver beyond the equation proposed in this paper has a high solution accuracy, with a maximum deviation of less than 5% and a deviation of less than 3% in most cases. This deviation is due to the limited number of output waveguides and imperfect sample preparation. Therefore, it should be emphasized that the accuracy of the solution can theoretically be improved by increasing the number of output waveguides.
In addition to excellent solution accuracy, the all-optical equation solver features ultrafast (~1.3 ps of light through feature structures) and energy-efficient computations (~92 fJ/bit).
Multiple logical gate operators
All-optical logic gates form the basic building blocks of ultra-high-speed all-optical chips, and any complex optical logic circuit can be composed of these logic gates. In addition, logic operations lay the foundation for more complex optical signal processing functions. However, current all-optical logic device designs based on signal ray coherence or nonlinear interactions still face challenges in enabling high-speed, low-power reconfigurability and versatile operation that implements multiple logic functions in a single chip.
The researchers took advantage of the scalability of the network to optimize a variety of on-chip all-optical logic devices. The design optimizes 6 input ports, including 2 signal inputs and 4 control bits, for a total of 5 layers. Sixteen logical functions can be implemented through seven different CNN structures. The choice of one of the optical CNN structures is illustrated.
Multiple logic gates.
Studies have shown that energy consumption as low as 10.4 aJ/bit can be achieved while maintaining a low error rate. In addition, the output logic states of an optical CNN are easily distinguishable when performing multiple logic functions. That said, more cascading extensions may still work in the future.
Semi-adder
The all-optical half-adder can perform the computational task of adding two input data bits together and generating a sum and a carry bit in the full light implementation. Here, an all-optical half-adder based on its optical CNN platform is shown.
Semi-adder.
Algorithmic optimization determines 12 network weights. The average light intensity contrast between logic states 0 and 1 is 14.2 dB. The flight time calculation time is 2.7 ps and the energy consumption is 50.8 fJ/bit. Further analysis showed that energy consumption as low as 23.8 aJ/bit could be achieved while maintaining a low error rate of 10^-9. The successful demonstration of the half-adder's capabilities while achieving high-intensity contrast further validates the high scalability of CNN designs and their wide range of all-light processing functions.
Ultra-fast and ultra-low energy consumption
In summary, the researchers experimentally demonstrated the first silicon waveguide-based physically fixed CNN for all-optical computing. Designed to implement a full-light transcendence equation solver, various logic gate operators, and half-adders, all exhibit ultrafast picosecond operations and ultra-low energy consumption in the order of tens of fly focal lengths per bit.
This optical network architecture is easy to extend and has the potential to be further extended by cascading the basic element structure to perform other complex computational tasks.
In addition, the platform offers the possibility of parallel computation using wavelength multiplexing. The work therefore points a promising direction for the next generation of all-optical computing systems.
Thesis link: https://www.oejournal.org/article/doi/10.29026/oea.2021.200060
Reference: https://phys.org/news/2021-11-all-optical-based-convolutional-neural-networks.html
Artificial Intelligence × [ Biological Neuroscience Mathematics Physics Materials ]
"ScienceAI" focuses on the intersection and integration of artificial intelligence with other cutting-edge technologies and basic sciences.
Welcome to follow the stars and click Likes and Likes and Are Watching in the bottom right corner.