(Report Producer/Author: Industrial Securities, Yu Xiaoli)
1. Three major systems of automatic driving: perception, decision-making, and execution
The development of driving technology is the process of replacing human driving with machine driving, so it can be compared with human driving, and autonomous driving technology is divided into three core links: perception decision-making and execution.
Perception refers to the ability to understand the scene of the environment. For example, the classification of data such as the type of obstacle, road signs and markings, detection of driving vehicles, traffic information, and so on. At present, there are two mainstream technology routes, one is a pure visual scheme dominated by cameras represented by Tesla; the other is a multi-sensor fusion scheme represented by Google and Baidu. According to the fusion stage, it is divided into pre-fusion and post-fusion. Pre-fusion refers to the identification of all sensor data as a whole, and post-fusion refers to the integration of the results of identification of different sensors.
Decision-making is to make task decisions based on driving scenarios and driving needs, and plan out the path of the vehicle and the corresponding body control signals. It is divided into four stages: task decision-making, trajectory planning, tracking control and execution control. Safety, comfort and speed of arrival need to be taken into account in the decision-making process.
Execution refers to the process of sending a control signal to the actuator, which executes. Actuators have steering, throttle, brake, light gear, etc. Because electric vehicle actuators are more linear and easy to control, they are more suitable for use as autonomous vehicles than fuel vehicles. In order to achieve more accurate execution capabilities, technologies such as steer-by-wire, brake-by-wire, and throttle-by-wire are constantly evolving. (Source: Future Think Tank)
2. Automatic driving classification
2.1 L1-L2 is driver assistance and L3-L5 is autopilot
The national standards GB/40429-2021 and SAEJ3016 clearly define the classification of automotive autonomous driving, dividing driving automation into levels 0 to 5. The principle that defines the hierarchy is 1) the degree to which the automated driving system is capable of performing dynamic driving tasks. 2) Role assignment of the driver. 3) There are no restrictions on allowing specifications. The national standard stipulates that the L1 and L2 level automation systems are named "driver assistance systems" and L3-L5 are named "automatic driving systems".
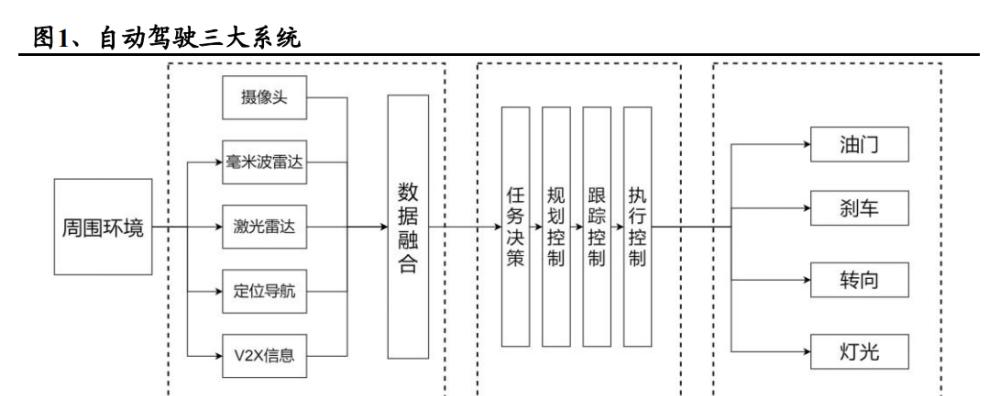
Specifically:
L0 Driving Automation—Emergency Assistance: This level of assisted driving system can sense the environment and provide information or briefly intervene in vehicle motion control, but cannot continuously perform vehicle control.
L1 Driving Automation—Partial Driver Assistance: This level of assisted driving system provides continuous lateral or vertical motion control. However, drivers still need to maintain supervision of road conditions and vehicle driving.
L2 Driver Automation – Combined Driver Assistance: This level of assisted driving system continuously provides lateral and vertical motion control. During the operation of this level of driving system, the driver communicates with the autopilot system to perform all driving tasks, allowing the user to briefly take their hands off the steering wheel, also known as Hands off.
L3 Driving Automation– Conditionally automated driving: The system continuously performs all driving tasks under design conditions. During normal operation, vehicle control, target detection and incident response are the responsibility of the automatic driving system, and the driver is requested to take over if the operating range is about to be satisfied. During operation, the user is allowed to briefly move their gaze out of driving, also known as Eyes off.
L4 Driving Automation – Highly Automated Driving: The system continuously performs all dynamic driving tasks and automatically executes the risk minimization strategy. When the system is out of range, a request for intervention is issued to the driver, and the driver may not respond to the request. During driving, the user's attention can be completely absent from driving, known as Mind off.
L5 Driving Automation – Fully Automated Driving: The system continuously performs all dynamic driving tasks and minimizes risk strategies in any drivable condition.
The essential difference between low-level assisted driving and high-level autonomous driving is the division of responsibility after an accident. According to the SAE's definition, we can find that after an accident, the responsibility for L2 lies with the passenger and the responsibility for L3 lies with the vehicle. Since the current domestic legislation on automatic driving has not yet been perfected, the promotion of automotive vehicle manufacturers for automatic driving can only stay in L2.5 or L2+. In 2021, the Japanese government took the lead in improving regulations, paving the way for the birth of the world's first L3-class self-driving car, the Honda Legend Hybrid EX.
2.2 Looking to the future: different levels of autonomous driving will coexist for a long time, and L5 still has a long way to go in the future
Vehicles below L3 and vehicles below L3 and above may coexist for a long time in the future. From a technical point of view, the implementation of L3 needs to rely on large computing chips, redundant systems at the hardware level, and massive user data. All of this adds a considerable cost to the autonomous driving system. In terms of cooperation, car companies adopt two research and development ideas to treat these two autonomous driving technologies. For low-level driver assistance systems, car companies adopt traditional R&D ideas and international Tier1 collaboration to pursue cost and safety. For high-end automatic driving systems, car companies generally adopt self-developed solutions. The hardware level adopts high-computing chip and redundant sensor configuration; the software level sets up a software development team to develop core perception and decision-making software from the software level, and pursues differentiation between car companies.
The process of increasing the level of autonomous driving is a process in which reliability continues to rise until quantitative change triggers qualitative change. The L5 level of automatic driving system stipulates that there is no design range limit, and the personnel in the car do not need to perform dynamic driving tasks or takeovers, which is also doomed to the L5 level of automatic driving will face greater challenges. (Source: Future Think Tank)
3. Automatic driving carries our good wishes for the liberation of labor
Driving is a dangerous thing. China will have 62,000 deaths in traffic accidents in 2020, with an average of 1 person dying every 8 minutes. According to a recent report from the University of Michigan's Transportation Institute, the highest traffic fatalities in 2014 were in Africa and Latin America, with Namibia having the highest traffic fatality rates in the world, with 45 deaths per 100,000 people. Thailand, a well-known tourist destination, ranks second, with a traffic death rate twice as high as china.
In terms of the proportion of traffic fatalities to total deaths, many Middle Eastern countries lead the way. The UAE ranked first with 15.9%. China's value is 3%, higher than the global average of 2.1%, and the US figure is 1.8%.
Driving is a waste of time. Consumers spend a lot of time on the road each year, with the Average U.S. spending more than 70 billion hours on the road in 2017, with an average of more than 52 minutes per American driving a day. In contrast to this figure, a car is used less than 5% of the time per day, and the other 95% of the time is parked in a parking space. In this context, the demand for autonomous driving has emerged.
3.1 Mankind's pursuit of autonomous driving has not stopped
Autonomous Driving Milestones:
Since the 1970s, people have been experimenting with the study of autonomous driving. After 2010, with the development of artificial intelligence, computer science and electric vehicles, autonomous driving began to enter a golden age.
[1920s - 1970s] Experiments with automotive automation began in the 1920s, but it was not until the 1950s that feasible experiments appeared and achieved some results.
The first semi-autonomous car, developed in 1977 by the Tsukuba Mechanical Engineering Laboratory in Japan, drove on specially marked streets and interpreted the markings through two cameras and an analog computer. With the support of elevated tracks, the vehicle reached a speed of 30 km/h (19 mph).
1980s – 2000s] Milestone self-driving cars appeared in the 1980s.
In 1985, Carnegie Mellon University's ALV program demonstrated 31 kilometers per hour (19 mph) of autonomous driving speed on two-lane roads, and in 1986 increased obstacle avoidance and in 1987 enabled off-road driving in daytime and nighttime conditions.
In 1995, Carnegie Mellon University's NavLab program completed the nation's first autonomous "coastal-to-shore driving." Of the 2,849 miles (4,585 km) between Pittsburgh, Pennsylvania, and San Diego, California, 2,797 miles (4,501 km) were realized by autonomous driving, with an average speed of 63.8 mph (102.7 km/h).
【2000-present】Autonomous driving assistance system is gradually being extended to mass production vehicles.
In 2004, Mobileye introduced its first mass-produced Soc, the EyeQ1, to support features such as Forward Collision Warning (FCW), Lane Departure Warning (LDW), and Intelligent High Beam Control (IHC).
In March 2004, the first DARPA Grand Challenge was held, and the participating teams used cameras, lidar and other sensors and computing equipment to realize the autonomous driving of the vehicle, which was the first appearance of the laser radar.
In 2013, four U.S. states (Nevada, Florida, California, and Michigan) passed regulations that would allow self-driving cars. In 2015, both the four states and Washington, D.C., allowed autonomous vehicles to be tested on open roads.
In October 2018, Waymo announced that its test vehicles had traveled more than 10,000,000 miles (16,000,000 kilometers) in autonomous mode, adding approximately 1,000,000 miles (1,600,000 kilometers) per month. In December 2018, Waymo pioneered the commercialization of a fully automated taxi service in Phoenix, Arizona, USA. In October 2020, Waymo launched a geo-fenced driverless ride-hailing service in Phoenix.
In March 2021, Honda launched a limited edition Legend Hybrid EX sedan with a newly approved Level 3 autonomous driving device, including the "Traffic Jam Pilot" system, the first L3 autonomous driving system approved in Japan and the world's first L3 autonomous driving system that can be driven on the road.
3.2 Two major factors drive the continued growth of autonomous driving
The driving force behind low-level assisted driving is the regulation. In addition to the demand from consumers to spontaneously purchase L2 driver assistance models, the driving force that promotes the development of low-level autonomous driving assistance by car companies is the regulation. In order to ensure the safety of drivers and passengers, Europe launched the General Safety Regulation in 2017, which clearly requires new cars sold in Europe in the future to be equipped with basic driving assistance functions such as Speed Limit Assist (ISA) and Emergency Brake Assist (AEB). Mainland China's "Technical Conditions for the Safety of Operating Trucks" requires relevant vehicles to have lane departure alarm functions and vehicle forward collision warning functions from September 2020, and automatic emergency braking systems will be installed from May 1, 2021.
The driving force behind higher-order autonomous driving is productivity. Consumers spend a lot of time on the road each year, and in 2017, the average U.S. spent more than 70 billion hours driving, with the average American spending more than 52 minutes a day. In contrast to this figure, less than 5% of a car is used every day, and the other 95% of the time is parked in a parking space. How to effectively use idle time has become the core driving force of high-level automatic driving.
By 2025, the number of autonomous driving systems for passenger cars in Mainland China is expected to reach 16.305 million units, with an assembly rate of 65.0%.
Front-view: In 2020, the front-view assembly volume of new passenger cars in China reached 4.968 million units, an increase of 62.1% year-on-year, and the assembly rate was 26.4%, up 10.9 percentage points year-on-year. With the improvement of the computing power, the increase of functions, and the relative cost advantage of the front-view system, it is expected that the assembly volume of the front-view system will exceed 16 million in 2025, and the assembly rate will increase to 65%.
At present, front-view monocular is the mainstream solution of domestic passenger cars, and some companies are also exploring the application of front-view cameras such as binoculars. In 2021, Huawei and DJI have successively launched self-developed binocular camera products and solutions. Among them, Huawei's binocular camera has been applied on the Polar Fox Alpha S. DJI also plans to apply the autonomous driving solution using binocular cameras to domestic models in 2021.
Surround View: In 2020, the assembly volume of China's Surround View system was 3.398 million units, an increase of 44% over 2019; the assembly rate was 18%, an increase of 6 percentage points year-on-year. With the replacement of the rear view of the rear view of the rear view of the rear and the addition of the parking function, the assembly capacity will be further increased. With the replacement of the rearview of the reverse rear view by the surround view system and the 360° panoramic surround view + ultrasound becoming the mainstream solution of converged parking, the 360 degree panoramic surround view has entered a new development cycle, and the assembly rate is expected to climb to 50% in 2025.
Visual DMS: According to the statistics of Zosz Automobile Research Institute, in 2020, more than 10 passenger cars in China have been listed with DMS functions, such as Changan Automobile, Weilai, Xiaopeng Automobile, WEY, Xingtu, Nezha Automobile, Zero Run, Geely Automobile, WM Automobile, GAC Aegean, etc. In 2020, dmS system assembly capacity reached 173,000 units, with an assembly rate of 0.9%, and it is expected to reach an assembly rate of about 20% by 2025, achieving leapfrog growth.
In April 2021, the Ministry of Industry and Information Technology issued the "Guidelines for the Administration of Access to Intelligent Connected Vehicle Manufacturers and Products (Trial)", which requires intelligent networked vehicles to have monitoring functions for human-computer interaction and driver participation behavior, and release a strong signal for DMS to get on the car.
Driving recorders: In 2020, the number of driving recorders assembled in China was 1.453 million units, an increase of 7.6% compared to 2019. During the same period, the assembly rate of driving recorders was 7.7%, an increase of 0.9 percentage points over the whole of 2019. The "Guidelines for the Management of Access to Intelligent Connected Vehicle Manufacturers and Products (Trial)" require intelligent connected vehicles to have event data recording and automatic driving data storage functions, which will accelerate the installation of driving recorders on new cars, and it is expected that by 2025, its assembly rate is expected to reach 20%.
3.3 China and the United States have increased financing for autonomous driving companies
After more than ten years of development, capital, industry, consumers and other sectors of the future of autonomous driving continue to be optimistic about the trend has formed a consensus, the road to commercialization is steadily rolling out, since 2021 the Chinese government has gradually accelerated the pace of policy guidance, local government policy support quickly to keep up to support scenario-based, commercial landing.
In recent years, with the popularity of research and development and application of autonomous driving technology, the scale of investment and financing in the global autonomous driving industry has grown rapidly. Since 2015, self-driving cars have gradually become a popular track for investment, and by 2021, there will be about 1,191 investments related to driving in China and the United States, involving more than $86.3 billion.
4. Industry Trends:
4.1 From global collaboration to regional independence:
Legal issues prevent data from circulating across borders, which will lead to inconsistencies in the routes and developments of autonomous driving technology between different countries. The data collected by self-driving cars includes sensor data (data collected by cameras, radar, thermal imaging equipment, and lidar) and driver data (driver details, location, historical route, driving habits). Self-driving cars collected less data in the early days of the industry, but with the development of autonomous driving technology, more and more data will be collected and analyzed. In order to protect this data and prevent the loss or misuse of data, governments around the world have introduced various regulations, which set the threshold for the development of autonomous driving technology.
Taking China as an example, there are two main factors restricting the development of multinational autonomous driving enterprises:
1) Surveying and mapping issues: The approval of licenses is strict and foreign investors are prohibited from participating.
In the perception link, self-driving cars will collect and process relevant data such as the surrounding natural environment and surface artificial facilities through cameras, radar, lidar and other sensors to help the car complete environmental perception. The related geographic data collection behavior is defined as "mapping behavior". Units engaged in surveying and mapping work shall obtain qualification certificates in accordance with law, including qualifications for "navigation electronic map production" and "Internet map services". Regarding the production of navigation electronic maps, according to the relevant provisions of the Special Administrative Measures for Foreign Investment Access (Negative List) (2020 Edition) and the Interim Measures for the Administration of Surveying and Mapping of Foreign Organizations or Individuals in China (2019 Amendment), the production of navigation electronic maps is a prohibited foreign investment project.
In the actual business development process, autonomous driving enterprises with foreign backgrounds often cooperate with surveying and mapping enterprises with corresponding qualifications to solve the license problem. However, with the pursuit of data security in the whole society, the requirements for enterprises carrying out surveying and mapping work in China will become higher and higher. As of March 25, 2022, China has only issued more than 30 navigation electronic map production licenses.
2) Data protection issues: Collection and export are subject to special restrictions
At present, the world's mainstream countries, represented by the European Union's General Data Protection Regulation (GDPR), are accelerating the formulation of personal information protection laws and regulating the collection, use and transfer of data. Generally speaking, for data regulation in the context of autonomous driving, it is mainly based on the provisions of various countries on network security and personal information protection, supplemented by special regulations on data in the automotive industry.
The "default do not collect" principle for key data. The transportation industry where autonomous driving is located is a "key industry" stipulated in the Cybersecurity Law. In addition to the need to process user data in a minimized manner, the "Several Provisions on the Security Management of Automobile Data" issued by the Cyberspace Administration of China on August 16, 2021 clarified six types of important data, namely, traffic flow data in important sensitive areas, high-precision map mapping data, operation data of automobile charging networks, types of road vehicles, traffic and other data, external audio and video data including faces, sounds, license plates and other external audio and video data, and other data that may affect national security and public interests. These 6 types of data use the "no collection by default" principle, which requires in-vehicle processing, non-essential non-provision to the outside of the vehicle, and data localization. At the same time, users have the right to ask car companies to stop data collection at any time.
Data export. According to China's Cybersecurity Law, self-driving service providers need to be particularly cautious when it comes to data export. For example, the principle of local data storage should be taken as the principle, and only in special circumstances can it be exported, and the data export must be subject to security assessment.
Therefore, Tesla's FSD function development in China is seriously affected by regulations, since the launch of the "Several Regulations on Automotive Data Security Management" in 2021, Tesla can not send data collected from China to the United States, resulting in the suspension of software iterations for China, and Tesla's FSD version in China is seriously behind North America.
More and more foreign car companies are aware of the necessity of localized autonomous driving technology research and development in China, and have accelerated the layout of autonomous driving in China: Tesla established a research and development center and data center in China at the end of 2021, and Volkswagen is also actively laying out localized autonomous driving business. In the future, we will see a localization operation model of obtaining data from China, iterating algorithms in China, and doing business in China.
We judge that in the future, the autonomous vehicles running in different regions will have distinct regional ecological characteristics. Data restrictions are also a kind of protection for local enterprises, and domestic enterprises are expected to develop more autonomous driving technologies that adapt to China's national conditions according to local conditions, such as multi-sensor fusion and vehicle-road collaboration as a supplement. In the United States, due to the sparse population, the intelligent route of bicycles will be more suitable.
4.2 It is difficult to open the scene, and the strategy of the self-driving enterprise is shifted to the closed scene
The development of autonomous driving on open roads has fallen short of expectations. Uber had announced plans to purchase 24,000 XC90s from Volvo to form a self-driving fleet by 2021, but in 2020 Uber abandoned self-development and sold the team to autonomous driving startup Aurora; Waymo announced in 2018 that it would purchase 20,000 I-PACE pure electric vehicles and 62,000 Pacifica hybrid vans from Jaguar Land Rover and FCA to build the Robotaxi fleet. However, less than 1,000 vehicles have been deployed so far, and only taxi services have been opened in San Francisco and Phoenix; domestic Ma Zhixing also shrank its strategy in October 2021, merging the truck team into the passenger car team.
After the opening of the road encountered obstacles, enterprises chose the closed scene as a breakthrough. In recent years, the gap between truck drivers and the rise in labor costs are becoming social problems that need to be solved urgently in the industry, and autonomous vehicles can replace drivers, greatly reduce driver labor costs and solve the problem of manpower shortage. At the same time, autonomous vehicles as production tools can improve safety and reduce the incidence of accidents in dangerous scenarios. Moreover, the closed scene of autonomous driving represented by ports and mining areas has developed rapidly due to the characteristics of relatively simple environment and closed environment, clear division of rights and responsibilities, etc., driven by policies and needs. In 2021, a number of new fully automated terminals will be built in China, driving a substantial breakthrough in the commercial operation of autonomous driving in ports; at the same time, under the trend of smart mine construction, a number of large-scale mining autonomous driving projects are entering the trial operation and testing stage.
We judge: 1) In the short term, more enterprises will realize the difficulty of autonomous driving in open scenarios, so as to change their strategies into closed scene tracks. 2) B-side scenario customers are mostly cost-oriented, and self-driving cars are regarded as productivity tools, so balancing safety and economy is the goal pursued by autonomous driving companies.
4.3 The battle for development routes will be reversed, and deep neural networks will replace artificial rules
In the early stage of the development of the industry, there was a dispute over the development route of autonomous driving, and there was a gradual realization of L4 from L1 and a leap forward of direct research and development of L4. The research and development idea of autonomous driving companies with a background of car companies is to gradually add new features from L1 until L4 is realized, representing Bosch, Mobileye and so on. However, some of the Internet background enterprises represented by Baidu and Waymo have adopted a one-step approach to directly develop L4-level autonomous driving, hoping to disrupt the industry with technology. However, with the development of artificial intelligence technology in recent years, the boundaries between gradual and leapfrog development have gradually blurred.
The evolution of software from a Rule based system to a learning Based System has had a profound impact on the decision-making process of software.
Rule based system refers to a system in which humans use inductive methods to use a series of rules in an attempt to derive the desired target. The system cannot handle complex issues such as object recognition and path planning.
The Learning Based System is a system that does not preset rules, allowing the system to learn rules from cases. Deep learning is one such system. Compared with rules-based systems, learning-based systems are more suitable for handling complex tasks because they do not require preset rules.
Many of the low-level autonomous driving systems are rules-based. Before the explosion of neural networks, traditional perception algorithms were traditional computer vision methods, which used rules-based decision-making logic, artificially set a series of rules, and hard-coded into the system. Because it does not require massive data, it has insufficient generalization ability and limited use scenarios, but this type of method has low computing power requirements and low cost, so it is easy to deploy and widely exists in L1 and L2 level automatic driving cars. Under the guidance of rule-based logic, auto companies divide automatic driving into two domains, "parking domain" and "high-speed domain", APA, RPA and other functions are controlled by the parking domain controller, and ACC and TJP functions are controlled by high-speed domain controllers. Car companies will designate different suppliers, and the technology stacks are not interoperable, and the scenes are not interoperable. In the prospectus published by Matrix Partners Hengrun, it can be found that "parking domain controller" and "high-speed domain controller" are two different hardware products, and the functions and purposes are completely inconsistent.
High-level autonomous driving neural networks are replacing rules-based systems. In the wake of the neural network explosion, industry scholars have embraced an end-to-end learning approach, directly training the algorithms needed to learn autonomous driving from the data collected by the sensors.
Tesla introduced the concept of one stack to Rule them All in its own self-driving FSD, that is, replacing the previously manually defined rules with neural networks, increasing the proportion of deep neural networks in the overall software system, replacing the previous hard-coded rules with neural networks, and finally achieving the goal of FSD, parking, summoning and other functions achieved by the same software.
We judge: 1) low-end autonomous driving assistance function (L2 and below) and high-end automatic driving assistance function (L3 and above) will use different technology stacks, low-level driving assistance function uses a rules-based system, and high-level automatic driving function uses neural networks. 2) Low-level and high-level autonomous driving functions will coexist to serve consumers with different needs. The advantage of low-level driving assistance is cost, and the advantage of high-level automatic driving function is the wide range of functions.
5. Route Dispute:
5.1 Bicycle intelligence VS vehicle-road collaboration: Bicycle intelligence is the mainstay, supplemented by vehicle-road collaboration
Different industrial advantages determine the direction of technology. The essence of the dispute over autonomous driving routes is that the advantages of each country are different, China's advantage lies in the communications industry, and the United States' advantage lies in the semiconductor industry. Therefore, China chooses the route supplemented by bicycle intelligence as the main vehicle road collaboration, and the United States chooses the route of bicycle intelligence. Bicycle intelligence uses automotive sensors to identify, and make decisions and execute at the end of the vehicle. Vehicle-to-road collaboration requires the installation of sensors on the road, transforming the road into a "smart road" and sending pedestrian, lane, and vehicle information to the car through V2X technology. Vehicle-to-road collaboration technology can reduce the sensor and computing platform requirements on the vehicle side and shift the cost to the cloud.
Bicycle intelligence: the business model is smooth, and downstream consumers are willing to pay. Autonomous driving in the intelligent mode of bicycles The business model is relatively simple, upstream parts companies (sensors, chips, domain controllers, and wire control chassis) provide parts for midstream automakers, and downstream consumers spend money to buy autonomous driving functions. High-end new energy models launched after 2021 generally reserve sufficient sensors and computing power resources for subsequent higher-end automatic driving. Take the flagship models of Weilai, Ideal and Xiaopeng as an example, they are all equipped with lidar and NVIDIA Orin chips with excess computing power. Although the high-end automatic driving function is still under development for the time being, the car company has sufficient hardware redundancy to support the subsequent realization of higher-order automatic driving functions through OTA iteration.
Vehicle-to-road synergy: The market potential is huge, but it faces business model challenges in the short term. In the context of new infrastructure, most of the existing vehicle-road collaboration projects are To G business, and the government supports autonomous driving enterprises to carry out business in the demonstration area, and there is a lack of end consumers to pay for the vehicle-road collaboration business. At the same time, vehicle-road coordination still lacks general standards in China, and enterprises still need to explore their own, so if vehicle-road coordination is to enter a virtuous development cycle, it is indispensable to balance the interests of the government, depots, highway operating units and other parties.
The core technology of vehicle-road collaboration is communication, and C-V2X is the main development direction of vehicle-road collaboration. The C-V2X standard set up by the mainland is the mainstream of the world's intelligent driving development, and it is currently in the commercial landing stage.
5.2 Pure Vision VS Multi-Sensor Fusion: The technical battle is difficult to have results in the short term
There are two solutions in the path of bicycle intelligent technology: pure vision solution and multi-sensor fusion solution.
The driver of the pure vision scheme is mainly Tesla, in which the camera plays a leading role, and it is necessary to map the 2D image captured by multiple cameras into 3D space, so the requirements for algorithms and computing power are high. The Model 3 and Model Y, which will be available in North America in 2021, eliminated radar and are equipped with only 8 cameras.
Multi-sensor fusion solutions, mainly promoted by Waymo and NVIDIA, introduce lidar that can directly measure distances, and auxiliary cameras calculate the distance and speed of objects to quickly build environmental 3D models.
Technology gaps and cost preferences make businesses choose different routes. Companies can increase the number of sensors to reduce the dependence on high-precision algorithms, and we believe that the choice of solutions is more reflective of the company's preference for technology and cost. Domestic companies lag behind Tesla in the pure visual route, multi-sensor fusion solutions can get rid of the dependence on visual technology, but need to increase expensive lidar, if you need to be equipped with a main lidar and two auxiliary lidar, the cost will increase a lot.
In the multi-sensor fusion scheme, it can be divided into pre-fusion and post-fusion according to the fusion and perception order. Pre-fusion arithmetic: The sensor passes the original data to the perception layer, which directly predicts, embodying the idea of "end-to-end" in a learning-based system. Post-fusion: Each sensor independently completes the target perception, and then the data is fused by another perception layer. We believe that because pre-fusion can reflect the "end-to-end" training method in deep learning, introducing fewer manual strategies, there will be better performance in the future.
6. The three core elements of driving: sensors, computing platforms, data and algorithms
6.1 Sensors: different positioning and functions, complementary advantages
Self-driving cars are often equipped with multiple sensors, including cameras, millimeter-wave radar, and lidar. These sensors have different functions and positioning, complementing each other's advantages; as a whole, they have become the eyes of autonomous vehicles. New cars from 2021 onwards are equipped with a large number of sensors, with the aim of reserving redundant hardware and enabling more autonomous driving functions through OTAs in the future.
Cameras: The industry is affected by the consumer electronics boom
The role of the camera: mainly used for lane lines, traffic signs, traffic lights and vehicles, pedestrian detection, there are comprehensive detection information, cheap characteristics, but will be affected by rain and snow weather and light. Modern cameras consist of lenses, lens modules, filters, CMOS/CCD, ISP, and data transmission. The light passes through the optical lens and filter and then focuses on the sensor, the optical signal is converted to an electrical signal through a CMOS or CCD integrated circuit, and then converted into a standard RAW, RGB or YUV digital image signal through an image processor (ISP), which is transmitted to the computer through a data transmission interface. Cameras can provide rich information. However, the camera relies on natural light sources, the current dynamic of the visual sensor is not particularly wide, in the light is insufficient or the light changes sharply when the visual picture may appear briefly lost, and in the rain and pollution conditions the function will be seriously limited, the industry usually through the way of computer vision to overcome the various shortcomings of the camera.
Car cameras are a high incremental market. The use of in-vehicle cameras has increased with the continuous upgrading of autonomous driving functions, such as 1-3 cameras for front view and 4-8 cameras for surround view. It is estimated that by 2025, the global car camera market will reach 176.26 billion yuan, of which the Chinese market is 23.72 billion yuan.
The automotive camera industry chain includes upstream lens group suppliers, glued material suppliers, image sensor suppliers, ISP chip suppliers, as well as midstream module suppliers, system integrators, downstream consumer electronics enterprises, autonomous driving Tier1, etc. CMOS Image Sensor accounts for 50% of the total cost in terms of value, followed by module packages accounting for 25% and optical lenses accounting for 14%.
Upstream optical components: divided into optical lenses, filters, protective films, wafers, etc. The industry players include Dali Optics, Sunny Optical Technology, Lianchuang Electronics, etc., with many participants and fierce competition.
The midstream is divided into lens groups, glued materials, DSP chips, CMOS image sensors, etc. Among them, CMOS is a semiconductor industry that can be mass-produced, with significant scale effects. The initial investment is huge, research and development is difficult, but the price of the product is not high, resulting in high barriers to entry in the industry. The CIS chip industry requires a long certification cycle, so it is difficult for new entrants to affect the existing pattern and will continue the competitive landscape of the strong and the strong. The automotive CIS chip industry has a high concentration, CR2 is 74%, mainly monopolized by international giants, ON Semiconductor is the world's largest supplier, with a market share of 45%; Weir shares have become the second in the world through the acquisition of Howey (USA), with a market share of 29%; Sony's market share of 6% is the third. This year,the chip industry is seriously out of stock, and the insufficient supply of ON Semiconductor CIS chips has led to some shares being cannibalized by other CIS companies, and we should continue to pay attention to changes in supply and demand.
CMOS sensor companies have four supply models, an IDM model that includes manufacturing, a partially outsourced Fablite model, a fully outsourced Fabless model, and a foundry foundry model. IDM companies can optimize iterate on new technologies more quickly due to the vertical integration of design and production, with the disadvantage of high cost of building moats. The Fablite model combines the advantages of the IDM model with the advantages of the Fabless model and is a model of collaboration that we believe has broader prospects.
Midstream module packaging: The module packaging market was originally monopolized by the traditional car Tier1 Bosch and Valeo, but in recent years, camera manufacturers in the field of consumer electronics (Sunny Optical Technology, Lianchuang Electronics, etc.) have also joined the competition. However, the performance of new entrants is vulnerable to the consumer electronics boom.
The first-mover advantage of the midstream packaging market is obvious, the parts and components of the car specification certification cycle is long, once the rail certification, that is, it can be bound to the downstream core customers, accompanied by customers to grow together. Globally, LG is currently Tesla's core camera supplier, signing a trillion won (53.5 billion won) with Tesla. 4.7 billion RMB) order. In China, only Lianchuang Electronics and Sunny Optical Technology have achieved large-scale vehicle camera shipments. Lianchuang Electronics is the supplier of the domestic version of Tesla, the supplier of NIO ET7, and the only camera company to obtain NVIDIA certification by December 2021; Sunny Optical Technology is the supplier of BMW, Mobileye, Mercedes-Benz, Audi, Tesla and other car companies.
Our judgment is:
At present, the revenue of consumer electronics business of in-vehicle camera companies is relatively high, and we should pay attention to the impact of the consumer electronics industry boom on the performance of camera companies.
The certification of core customers is the source of growth for camera companies.
The scale effect of the CIS chip industry is obvious, and the market share of the leading market is high.
LiDAR: The technical route is undecided, and the probability of Chinese companies winning is high.
The role of lidar: mainly used to detect the distance and speed of surrounding objects. At the emitting end of the lidar, a high-energy laser beam is generated by the laser semiconductor, and after the laser collides with the surrounding target, it is reflected back, captured and computed by the receiving end of the lidar to obtain the distance and speed of the target. Laser radar has higher detection accuracy than millimeter wave and camera, and can detect long distances, often reaching more than 200 meters. According to its scanning principle, lidar is divided into mechanical, rotary mirror, MEMS and solid-state laser radar. According to the ranging principle, it can be divided into time-of-flight ranging (ToF) and frequency modulated continuous wave (FMCW).
At present, the industry is in the exploration stage of lidar applications, and there is no clear direction, and it is impossible to clarify which technical route will become the mainstream in the future.
The lidar market is vast, and Chinese companies will lead the United States. We predict that by 2025, China's lidar market will be close to 15 billion yuan and the global market will be close to 30 billion yuan; by 2030, China's lidar market will be close to 35 billion yuan, the global market will be close to 65 billion yuan, and the annualized growth rate of the global market will reach 48.3%. Tesla, the largest autopilot company in the United States, adopts a pure visual solution, and other car companies have no specific plans to get on the car with laser radar, so China has become the largest potential market for vehicle-mounted lidar. In 2022, a large number of domestic automakers will launch products equipped with lidar, and it is expected that the shipment volume of on-board lidar products will reach 200,000 units in 2022. The reason why Chinese companies have a better probability of winning is that Chinese companies are closer to the market, have a high degree of cooperation with Chinese automakers, and are more likely to obtain market orders, so the cost reduction speed will be faster, forming a virtuous circle. China's vast market will help Chinese lidar companies to bridge the technology gap with foreign companies.
The upstream of lidar is manufactured for components, which can be divided into transmitting systems, receiving systems, scanning modules, and control and processing modules according to their functions.
The middle reaches of lidar are lidar design and manufacturing enterprises. At present, there are 7 lidar companies listed on the US stock market, representing different technical routes. Luminar's technical route represents 1550nm fiber laser hybrid solid-state lidar; Israel Innoviz's technical route represents hybrid solid-state lidar with silicon-based MEMS galvanometers; Aeva's technical route is to develop lidar with FMCW technology; Velodyne's technology route is rotating mechanical lidar; Ouster is Flash lidar with VCSEL+SPAD technology; and Cepton is adopted The dynamic motor plus two-dimensional scanning technology way to do lidar; Aeye provides camera and lidar fusion product iDAR. China's lidar companies are also making rapid progress, among which Livox, Sagitar Juchuang, Tudtong, and Hesai Technology have all achieved mass production.
The stock prices of these 7 companies have all fallen significantly after listing, because 1) Tesla's pure visual route is progressing too fast, and the market questions the necessity of lidar. 2) The mass production progress of lidar in the United States is less than expected.
Each technical route in the current stage has its own advantages and disadvantages, and our judgment is that in the future, FMCW technology will coexist with TOF technology, the 1550nm laser emitter will be better than 905nm, and the market may skip the semi-solid state and directly cross to the all-solid stage.
FMCW technology and TOF technology coexist: TOF technology is relatively mature, with fast response speed, high detection accuracy advantages, but can not directly measure the speed; FMCW can directly measure the speed through the Doppler principle and high sensitivity (more than 10 times higher than ToF), strong anti-interference ability, long-distance detection, low power consumption. In the future, FMCW may be used for high-end products and TOF for low-end products.
1550nm is better than 905 nm: 905nm belongs to the near-infrared laser, which is easily absorbed by the human retina and causes retinal damage, so the 905nm scheme can only be maintained at low power. 1550nm laser, the principle is visible spectrum, the laser under the same power conditions of less damage to the human eye, detection distance is farther, but the disadvantage is that InGaAs is required to do the generator, and can not use silicon-based detectors.
Skip the semi-solid state and go directly to the all-solid state: the existing semi-solid solution mirror type, angular type, MEMS, there are a small number of mechanical components, the vehicle environment has a short service life, it is difficult to pass the vehicle regulation certification. The VCSEL+SPAD solution of solid-state lidar adopts chip-level process, simple structure, easy to pass the car rules, and has become the most mainstream technical solution of pure solid-state laser radar. The lidar behind the iPhone12 pro uses the VCSEL + SPAD solution.
Our judgment is:
Pay close attention to the dispute over the automatic driving route, the hundred flowers of the sensor are based on the premise that there is no specific plan to dominate the automatic driving for the time being, and the rapid progress of the pure visual route will adversely affect the lidar enterprises.
Domestic lidar companies are closer to the market.
Pay attention to the progress of the main promoters of domestic lidar, Huawei, Weilai, Ideal, and Xiaopeng's autonomous driving function.
Bullish VCSEL + SPAD route
Companies on the 1550nm laser route: Luminar (lidar host), Lumentum (1550nm laser).
High-precision map: There is a possibility of being subverted, and grade A surveying and mapping qualifications build a moat
There is a possibility that HIGH-definition maps will be subverted. The battle of routes continues in the field of advanced maps, Tesla proposed a high-precision map that does not need to be mapped in advance, based on the data collected by the camera, using artificial intelligence technology to build a three-dimensional space of the environment, using crowdsourced thinking, providing road information by each vehicle, and unified aggregation in the cloud. Therefore, we need to be wary of technological innovations that subvert high-precision maps.
Some practitioners believe that high-precision maps are indispensable for intelligent driving, from the field of view, high-precision maps are not obscured, there is no distance and visual defects, in special weather conditions, high-precision maps can still play a role; from the error point of view, high-precision maps can effectively eliminate some sensor errors, in some road conditions, can effectively supplement the existing sensor system for supplementary correction. In addition, high-precision maps can also build a driving experience database, through the mining of multi-dimensional spatio-temporal data, analyze the danger area, and provide drivers with a new driving experience data set.
Navigation electronic map production qualification is the enterprise moat. In the perception link, self-driving cars will collect and process relevant data such as the surrounding natural environment and surface artificial facilities through cameras, radar, lidar and other sensors to help the car complete environmental perception. The relevant geographic data collection behavior is defined as the "mapping behavior". Units engaged in surveying and mapping work shall obtain qualification certificates in accordance with law, including qualifications for "navigation electronic map production" and "Internet map services." Regarding the production of navigation electronic maps, according to the relevant provisions of the Special Administrative Measures for Foreign Investment Access (Negative List) (2020 Edition) and the Interim Measures for the Administration of Surveying and Mapping by Foreign Organizations or Individuals in China (2019 Amendment), the production of navigation electronic maps is a project prohibited from foreign investment.
In the actual business development process, autonomous driving enterprises with foreign backgrounds often cooperate with surveying and mapping enterprises with corresponding qualifications to solve the license problem. However, with the pursuit of data security in the whole society, the requirements for enterprises carrying out surveying and mapping work in China will become higher and higher. As of March 25, 2022, China has only issued more than 30 navigation electronic map production licenses.
Lidar + vision technology, collection car + crowdsourcing mode is the mainstream scheme of high-precision maps in the future.
HIGH-precision maps need to balance the two measures of accuracy and speed. Too low acquisition accuracy and too low update frequency can not meet the needs of autonomous driving for high-precision maps. In order to solve this problem, HIGH-precision map companies have adopted some new methods to deal with it, such as the crowdsourcing model, where each self-driving car provides high-precision dynamic information as a collection device for high-precision maps, and summarizes them and distributes them to other cars. In this mode, the leading head HD map enterprises can collect more accurate and fast HD maps due to the large number of models that can participate in crowdsourcing, so as to maintain the situation of strong and strong.
Our judgment is:
Focusing on advances in self-driving technology, Tesla's pure vision scheme does not require advanced maps and could have a fundamental impact on the industry.
6.2 Computing platform: The requirements for chips are constantly increasing, and semiconductor technology is a moat
Computing platforms are also called autonomous domain controllers. With the increase in the penetration rate of automatic driving above L3, the requirements for computing power have also increased, although the current L3 regulations and algorithms have not yet been introduced, but the vehicle enterprises have adopted the redundant computing power scheme to reserve space for subsequent software iterations.
The future development of computing platforms has two characteristics: heterogeneity and distributed elasticity.
Heterogeneous: For high-end autonomous vehicles, the computing platform needs to be compatible with multiple types, multiple data sensors, and have high safety and high performance. The existing single chip can not meet many interface and computing power requirements, the use of heterogeneous core hardware solutions. Heterogeneous can be reflected in the integration of multiple architecture chips on a single board card, such as Audi zFAS integrated MCU (microcontroller), FPGA (programmable gate array), CPU (central processing unit), etc.; it can also be embodied in the powerful single chip (SoC, system-on-chip) at the same time integrated multiple architecture units, such as Nvidia Xavier integrated GPU (graphics processor) and CPU two heterogeneous units.
Distributive elasticity: Current automotive electronic architectures are increasingly integrated into domain controllers by a number of single-function chips. High-end automatic driving requires the on-board intelligent computing platform to have the characteristics of system redundancy and smooth expansion. On the one hand, heterogeneous architecture and system redundancy are used to achieve system decoupling and backup; on the other hand, multi-board card distribution and expansion are used to meet the requirements of high-level automatic driving for computing power and interface. Under the unified management and adaptation of the same automatic driving operation system, the overall system cooperates to realize the automatic driving function, and adapts different chips by changing the hardware driver and communication service. With the improvement of the level of autonomous driving, the demand for computing power and interfaces of the system will increase day by day. In addition to increasing the computing power of a single chip, it is also possible to repeatedly stack hardware components to achieve flexible adjustment and smooth expansion of hardware components, so as to realize the improvement of the computing power of the entire system, increase interfaces, and improve functions.
The heterogeneous distributed hardware architecture is mainly composed of three parts: AI unit, computing unit and control unit.
AI Unit: Uses a parallel computing architecture AI chip and configures the AI chip and necessary processors using a multi-core CPU. At present, AI chips are mainly used for efficient fusion and processing of multi-sensor data, and the output is used for key information for execution layer execution. AI units are the largest part of the computing power requirements in heterogeneous architectures, and need to break through the bottlenecks of cost power consumption and performance to achieve industrialization requirements. AI chips can be selected from GPUs, FPGAs, ASICs (dedicated integrated circuits), etc.
Compute unit: A compute unit consists of multiple CPUs. It has the characteristics of high single-core main frequency and strong computing power, and meets the corresponding functional safety requirements. It is equipped with hypervisor, Linux kernel management system, manages software resources, completes task scheduling, executes most of the core algorithms related to autonomous driving, and integrates multiple data to achieve path planning and decision-making control.
Control unit: Mainly based on conventional vehicle controllers (MCUs). The control unit is loaded with the basic software of the Classic AUTOSAR platform, and the MCU is connected to the ECU via a communication interface to achieve cross-longitudinal control of vehicle dynamics and meet the requirements of the ASIL-D level of functional safety.
Tesla FSD chip, for example, FSD chip using CPU + GPU + ASIC architecture. Contains three quad-core Cortex-A72 clusters with a total of 12 CPUs running at 2.2 GHz, a Mali G71 MP12 GPU running at 1 GHz, 2 neural processing units (NPUs), and a variety of other hardware accelerators. There is a clear division of labor between the three types of sensors, with the Cortex-A72 core CPU for general-purpose compute processing, the Mali core GPU for lightweight post-processing, and the NPU for neural network computing. The GPU hash rate reaches 600GFLOPS, and the NPU hash rate reaches 73.73Tops.
The technical core of the autonomous domain controller is the chip, followed by software and operating system, and the short-term moat is the customer and delivery capabilities.
The chip determines the computing power of the automatic driving computing platform, which is difficult to design and manufacture, and it is easy to become a card neck link. The high-end market is dominated by international semiconductor giants Nvidia, Mobileye, Texas Instruments, NXP, etc.; domestic enterprises represented by Horizon in L2 and below markets have gradually gained customer recognition. China's domain controller manufacturers generally cooperate with a chip manufacturer in depth, purchase chips, and deliver their hardware manufacturing and software integration capabilities to automakers. Cooperation with chip companies is generally exclusive. From the perspective of chip cooperation, Desay SV is bound to NVIDIA and Zhongke Chuangda is bound to Qualcomm, and the advantages are the most obvious. Huayang Group, another domestic autonomous driving domain controller company, has established a cooperative relationship with Huawei HiSilicon and Neusoft Ruichi and NXP and Horizon.
The competitiveness of domain controllers is determined by the upstream cooperative chip companies, and the downstream OEMs often purchase a complete set of solutions provided by chip companies. For example, the high-end models of Weilai, Ideal and Xiaopeng are purchased from NVIDIA Orin chips and NVIDIA automatic driving software; Extreme Kr and BMW are purchased from chip companies Mobileye's solutions; Chang'an and Great Wall are purchased from Horizon L2 solutions.
Our judgment is:
Continuous tracking of the penetration rate of automatic driving above L3, and the increase in penetration rate is the core logic of the chip track.
Pay attention to the opportunities for domestic substitution at the chip level: Horizon (autonomous driving chip), Fudan Microelectric (FPGA).
6.3 Data and algorithms: Data helps to iterate on algorithms, and algorithm quality is the core competitiveness of autonomous driving enterprises
User data is extremely important for retrofitting autonomous driving systems. In the process of automatic driving, there is a rare scenario with a low probability of occurrence, which is called a corner case. If the perception system encounters a corner case, it poses a serious security risk. For example, Tesla's Autopilot, which occurred in previous years, did not identify a large white truck that was crossing, directly hitting it from the side, resulting in the death of the owner; in April 2022, Xiaopeng hit a vehicle that overturned in the middle of the road while turning on self-driving.
There is only one solution to such a problem, that is, the car company takes the lead in collecting real data, and at the same time simulates more similar environments on the autonomous driving computing platform, so that the system can learn to better deal with it next time. A classic example is Tesla's shadow pattern: identify potential cornercases by comparing them to human driver behavior. These scenes are then annotated and added to the training set.
Correspondingly, car companies need to establish data processing processes so that the real data collected can be used for model generation, and the iterative model can be installed on real mass production vehicles. At the same time, in order to make machine learning cornercases on a large scale, after obtaining a cornercase, large-scale simulations will be carried out for the problems encountered by this cornercase, and more cornercases system learning will be derived. Nvidia DriveSim, a simulated platform developed using metacostem technology, is one of the simulation systems. Data-leading companies build data moats.
1) Determine whether the autonomous vehicle encounters a corner case and upload it
2) Label the uploaded data
3) Simulate and create additional training data using simulation software
4) Iteratively update the neural network model with data
5) Deploy models to real vehicles via OTA
According to NVIDIA's statement at 2022CES, companies investing in L2 driver assistance systems only need 1-2,000 GPUs, while companies developing complete L4 autonomous driving systems need 25,000 GPUs to build data centers.
1. Tesla currently has 3 major computing centers with a total of 11544 GPUs: the auto-tagging computing center has 1752 A100 GPUs, the other two computing centers used for training have 4032 and 5760 A100 GPUs respectively; the self-developed DOJO supercomputer system released in 2021 AI DAY has 3000 D1 chips, with a hash power of up to 1.1 EFLOPS.
2. SenseTime's Shanghai Supercomputing Center project under construction has planned 20,000+A100 GPUs, and the peak computing power will reach 3.65EFLPOS (BF16/CFP8) after all of them are completed.
Our judgment is:
Algorithm talents are an important resource of autonomous driving enterprises, and the flow of core technical personnel of autonomous driving enterprises should be observed in real time.
Data is not a panacea, but it is impossible without data. Businesses with data leaders are more likely to have an advantage.
Autonomous driving will have a lot of cloud computing needs.
Self-driving companies need to set up training centers.
7. Tesla's autopilot is thriving
Tesla's core advantages in autonomous driving are: 1. The software and hardware are completely self-developed. 2. Massive amount of real data. 3. The Dojo supercomputer gives Tesla the ability to iterate quickly.
1. Years of experience in autonomous driving exploration, let Tesla know how autopilot software and hardware need to cooperate, in 2019 officially entered the era of software and hardware completely self-research.
Tesla has been groping for a long time in the computing platform and finally chose the path of self-research. Before 2019, Tesla used autonomous driving chips from external suppliers Mobileye and Nvidia, and HW3.0 after 2019 used two self-developed FSD chips. FSD chip designed by Tesla, manufactured by Samsung 14nm process, encapsulated about 6 billion transistors on the silicon wafer of 2602, neural network accelerator (NPU), Tesla uses a self-developed architecture, designed 2 NNA cores, each core can perform 8-bit integer calculations, running frequency of 2GHz, the peak computing power of a single NNA is 36.86TOPS, and the peak computing power of 2 NNA is 73.7TOPS.
In 2022, Tesla will also launch HW4.0 to further strengthen computing power.
Camera: Remove the ISP and tap into the potential of the camera. Tesla is the only self-driving company in the world that uses a pure vision approach and has a unique insight into how to use cameras. The latest FSD beta version camera raw data will no longer need to be processed by the ISP module and can be fed directly into the neural network for the next calculation. This method ensures that as much data as possible is carried in the image data, while also reducing latency during image processing by 20%.
In response to the question that the visual solution cannot accurately measure the distance, Tesla has developed a "pseudo-lidar" algorithm based on pure visual ranging technology, which has achieved excellent results.
Data & Algorithms:
Led by AI Director Andrej Karpathy, Tesla's algorithm has undergone multiple iterations, seven iterations in Q1 2022 alone.
Tesla was the first company in the world to dare to announce its self-driving software architecture. Judging from the information released on Tesla AI day:
Perception Layer: Tesla automatically annotates based on images from 8 cameras, while considering temporal information when modeling in 3D and predicting the intentions of environmental objects (e.g., humans, cars). This modeling method can take a sufficient number of vehicle models and build a complete 3D model of the road in the cloud, thus removing the dependence on high-precision maps.
Planning Layer: Terrass uses the Monte Carlo tree search algorithm to achieve a balance between shorter time, safer routes, and more comfortable rides, which requires a lower number of iterations than traditional planning algorithms.
2. Unswervingly take the path of pure vision, 1.5 million stock models give Tesla a large number of users of real data. In terms of data, with the help of thousands of Tesla models running on the road, Tesla can collect more data than other car companies to achieve data hegemony. It is expected that by the first quarter of 2022, there will be about 1.5 million models equipped with HW3.0 platform hardware for Tesla to collect data. Based on the 5,000 kilometers driven by bicycles per quarter, Tesla has collected about 9 billion kilometers of data (excluding simulations).
3. The Dojo supercomputer platform gives Tesla the ability to quickly train iterations.
On August 20, 2021, at Tesla AI Day, Tesla released its self-developed AI chip D1 for training the supercomputer Dojo. It is the building block of the computing plane based on a large multi-chip module (MCM), which is composed of 120 MCM tiles and integrates to achieve ultra-high computing power of 1.1 EFLOPS, which is the fastest AI training computer in the world.
Dojo is a supercomputer that can use massive amounts of video data to do unsupervised annotation and training. This includes two key points: the first focus is on the collection of massive data; the second is on unsupervised labeling and training. Created from an array of 354 training nodes, the D1 chip uses a 7nm manufacturing process to implement 362TFLOPS machine learning calculations that automatically learn and identify pedestrians, animals, potholes, and other data on marked roads. After collecting massive amounts of data in Dojo, the algorithm evolution is continuously enhanced by automating deep neural network training without the need for a large number of researchers, greatly improving the training efficiency, and finally achieving fully automatic driving (FSD).
Tesla's timeline for achieving autonomous driving is to achieve Full Self Driving, which is safer than human driving by the end of 2022, and to commercialize autonomous driving in 2024. We think tesla is highly likely to achieve autonomous driving along this timeline.
(This article is for informational purposes only and does not represent any of our investment advice.) For usage information, see the original report. )
Featured report source: [Future Think Tank].