On April 12, iFLYTEK, the first State Key Laboratory of Cognitive Intelligence in Mainland China, topped the Common Sense Reasoning Challenge Common Sense QA 2.0 with a score of 76.06%, setting a new world record and taking a big step in making machines "understand and think"!
CommonsenseQA 2.0 is an international common sense reasoning assessment dataset led by the Allen Institute for AI in 2021, which aims to assess the machine's understanding and mastery of common sense knowledge, attracting many top international institutions including Google, Allen Institute for AI, university of Washington and many other top international institutions to participate in the challenge. iFLYTEK's first participation in the competition, that is, innovatively proposed the ACROSS model, with the absolute advantage of the new deep learning algorithm, to refresh the world record of the level of common sense reasoning of the machine.
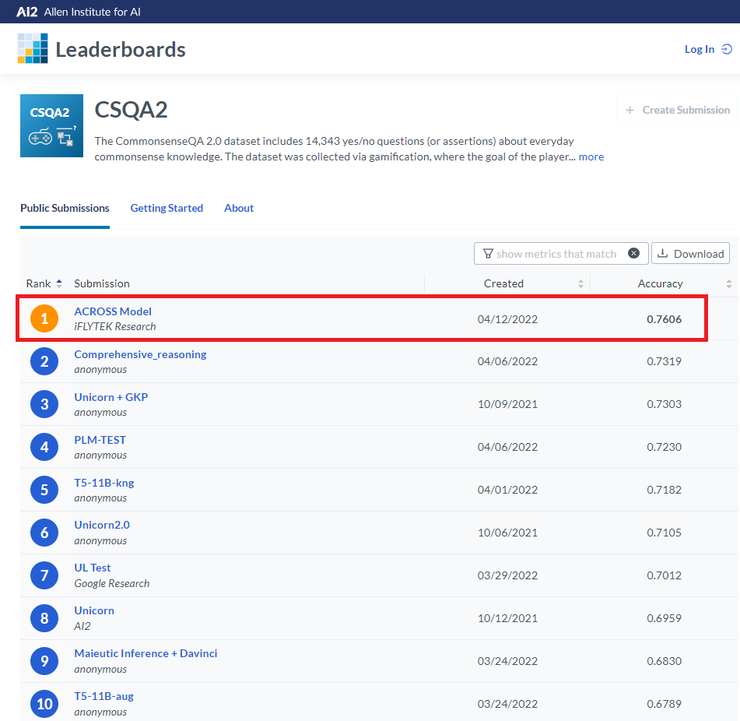
From doing "multiple choice questions" to "judgment questions", how difficult is it for machines to learn common sense reasoning?
Commonsense Reasoning is one of the most important directions forward for NLP, and its purpose is to help computers learn common sense knowledge and use the knowledge they have to make deep understanding and reasoning. Progress and technological breakthroughs in this field are of great significance to the development of artificial intelligence.
The types of questions that the current typical reading comprehension model focuses on are mainly factual questions, and the answers to these types of questions can often be found directly in the original text, but how to reason based on common sense and background knowledge to obtain answers is still a huge challenge.
CommonsenseQA is a dataset set up to train machines to respond like humans based on prior knowledge combined with real-world situations. When people answer questions, they often use their knowledge combined with specific backgrounds to judge the answers to the questions. For example, common sense, background knowledge, spatial relations, scientific facts, social conventions, etc.
CommonsenseQA 1.0 task example
The second question in the example of the task in the figure above, "Where can I stand on the river and watch the current without wetting myself?" "It can be inferred from the selection that I am on the bridge. This knowledge may seem easy to understand for humans, but how to get machines to learn common sense and background knowledge and make accurate reasoning is still a huge challenge.
CommonsenseQA 2.0 is a binary classification dataset of 14,343 questions divided into training/development/testing sets that require judging whether common-sense statements are right or wrong. The problems examined in version 1.0 are built on the knowledge triad in the existing common sense knowledge base ConceptNet, which gives the machine the knowledge to focus directly on the reference when working on this task. Compared with the 1.0 "multiple choice question" and the 2.0 "judgment question" challenge is more difficult, only given a subject entity or concept, a common sense class relationship (and the relationship does not necessarily appear in the existing knowledge base), allowing humans to construct the common sense knowledge that is difficult for machines to grasp in a natural language way.
The common-sense reasoning problem constructed by this constructor method has a huge imagination space, most of which is not covered in the current knowledge base, which undoubtedly significantly increases the difficulty of the machine to deal with such problems. At the same time, in the process of data construction of the evaluation task, iterative design is also continuously iteratively designed through the continuous game confrontation between man and machine, and the final set of problems is basically a problem that the current mainstream algorithms have not completed well.
At present, the Chinese intelligent forces represented by iFLYTEK have made great progress in the field of common sense reasoning, but they are still far below the level of 94.1% of human beings, which shows that there are still great challenges and room for progress in the direction of common sense reasoning.
CommonsenseQA 2.0 task problem example
Examples of common sense question types covered by commonsenseQA 2.0 tasks
iFLYTEK proposes an innovative ACROSS approach to solve the problem
In the challenging common sense reasoning evaluation task of CommonsenseQA 2.0, the mainstream medium-sized pre-trained model method in the industry can only achieve a level of 55%, which is slightly higher than the average random guess. Previously, the optimal method of this task in the world was to generate relevant knowledge for common sense QA 2.0 common sense reasoning problems through a GPT3 model with a parameter size of 175 billion, and fuse processing based on the T5 model, which achieved an accuracy rate of 73%.
The Automatic Commonsense Reasoning on Semantic Spaces model, which was innovatively proposed by the team of the State Key Laboratory of Cognitive Intelligence undertaken by iFLYTEK, is the successor to the Neural Association Model proposed by iFLYTEK in 2016 and the Winograd Schema Another masterpiece after the Challenge championship.
The model achieves an effective fusion of external knowledge in a unified semantic space, significantly improves the problems existing in the hyperscale pre-training model, and achieves a 76% accuracy rate on the CommonsenseQA 2.0 task.
The common-sense reasoning questions in this review are difficult to find direct answers to, whether in knowledge bases such as ConceptNet or on the Internet. Starting from the habit of human beings to use common sense knowledge and reasoning, for a complex problem, it is first necessary to consult the relevant knowledge base or classics, and then use the Internet search to find relevant information. The ACROSS model is to learn from this idea, fully collect the knowledge base, Internet related information, in a unified semantic space for fusion processing, and finally give the super-large-scale pre-training model a stronger knowledge input, to achieve accurate common sense knowledge reasoning. The results of this method also prove to a certain extent that the machine has the ability to understand and apply all kinds of complex text information and knowledge.
The mainland's technological attack in the field of common-sense reasoning continues.
The next development of artificial intelligence technology must break through the bottleneck of common sense reasoning, in order to make artificial intelligence products more practical in the national economy and people's livelihood scenarios such as education, medical care, and pensions.
"Let the machine listen, understand and think" is a clear and predictable future, and iFLYTEK has not stopped searching.
Leifeng Network