--Follow reply "40429"--
Collect the Automotive Driving Automation Classification (GB/T 40429-2021)
2022 is the window period for intelligent driving from L2 to L3/L4, more and more car manufacturers have begun to lay out higher-level intelligent driving mass production, and the era of automobile intelligence has quietly arrived.
With the technical improvement of lidar hardware, vehicle specification-level mass production and cost downturn, high-level intelligent driving functions have promoted the mass production of lidar in the passenger car field, and a number of models equipped with lidar will be delivered this year, and 2022 is also known as the "first year of lidar on board".
Lidar sensor vs image sensor
Lidar is a sensor used to accurately obtain the three-dimensional position of an object, essentially laser detection and ranging. With its excellent performance in target contour measurement, universal obstacle detection, etc., it is becoming the core configuration of L4 autonomous driving.
However, the ranging range of lidar (generally around 200 meters, the specifications of mass production models of different manufacturers vary) result in a perception range much smaller than that of image sensors.
And because its angular resolution (generally 0.1 ° or 0.2 °) is relatively small, resulting in the resolution of the point cloud is much smaller than the image sensor, when perceived at a distance, the point projected on the target may be extremely sparse, or even unable to image. For point cloud object detection, the effective distance of the point cloud that the algorithm can really use is only about 100 meters.
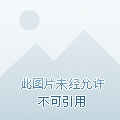
Image sensors can acquire complex surrounding information at high frame rates and high resolutions, and are inexpensive, and multiple sensors with different FOVs and resolutions can be deployed for visual perception of different distances and ranges, and the resolution can reach 2K-4K.
However, the image sensor is a passive sensor, the depth perception is insufficient, the ranging accuracy is poor, especially in the harsh environment to complete the difficulty of the perception task will be greatly improved.
In the face of strong light, low illumination at night, rain, snow and fog and other weather and light environments, intelligent driving requires high requirements for sensor algorithms. Although lidar is not sensitive to ambient light, ranging will be greatly affected by waterlogged pavement, glass walls, etc.
It can be seen that lidar and image sensors have their own advantages and disadvantages. Most high-level intelligent driving passenger cars choose to fuse different sensors to complement each other's strengths and integrate redundancy.
Such convergent perception schemes have also become one of the key technologies for high-level autonomous driving.
Point cloud and image fusion perception based on deep learning
The fusion of point clouds and images belongs to the technical field of multi-sensor fusion (MSF), there are traditional stochastic methods and deep learning methods, according to the degree of abstraction of information processing in the fusion system, it is mainly divided into three levels:
Early Fusion
The observational data of the sensor is first fused, and then the features are extracted from the fused data for identification. In 3D object detection, PointPainting (CVPR20) adopts this method, the PointPainting method first does semantic segmentation of the image, and maps the segmented features to the point cloud through the matrix of point-to-image pixels, and then sends the point cloud of the "drawn point" to the detector of the 3D point cloud to return to the target box.
Deep Fusion
Each natural data feature is extracted from the observational data provided by each sensor, and the features are fused and identified. In the fusion method based on deep learning, this method adopts feature extractors for point clouds and image branches, and the networks of image branches and point cloud branches are fused semantically level by semantic level in the pre-feedback level to achieve semantic fusion of multi-scale information.
The feature layer fusion method based on deep learning requires high space-time synchronization between multiple sensors, and once the synchronization is not good, it directly affects the effect of feature fusion. At the same time, due to the difference in scale and viewing angle, it is difficult for LiDAR and image feature fusion to achieve the effect of 1+1>2.
Late Fusion
Relative to the first two, it is the least complex fusion method. It is not fused at the data layer or feature layer, it is a target-level fusion, and the different sensor network structures do not affect each other, and can be trained and combined independently.
Because the two types of sensors and detectors fused by the decision layer are independent of each other, once a sensor fails, sensor redundancy can still be performed, and the engineering robustness is better.
With the continuous iteration of lidar and visual fusion perception technology, as well as the accumulation of knowledge scenarios and cases, more and more full-stack converged computing solutions will bring a safer and more reliable future to autonomous driving.
-- END --