Reports from the Heart of the Machine
Editors: Chen Ping, Xiao Zhou
ICLR 2022 announced the list of winners, with 7 outstanding paper awards and 3 honorary nominations for outstanding papers.
ICLR 2022 Outstanding Paper Awards announced! A total of 7 papers were selected as Outstanding Papers Awards this year for their outstanding organization, insight, creativity and lasting impact. In addition, ICLR 2022 has announced 3 honorary nominations for outstanding papers.
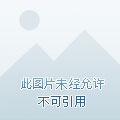
At the beginning of this year, ICLR 2022 released the results of the conference' papers: 54 Oral (oral report) papers and 176 Spolight papers, with a total of 1095 papers received, 3391 final submissions, and a paper acceptance rate of 32.3%.
Now, ICLR 2022 has officially selected 7 outstanding papers from 1095 received papers, and the winning authors are from Tsinghua University, the Hillhouse School of Artificial Intelligence of Chinese University, Google Research, the University of Antwerp, Stanford University, Cornell University, the University of Toronto, DeepMind and other institutions. Among them, Academician Zhang Cymbal and Professor Zhu Jun's paper "ANALYTIC-DPM: AN ANALYTIC ESTIMATE OF THE OPTIMAL REVERSE VARIANCE IN DIFFUSION PROBABILISTIC MODELS" won the Outstanding Paper Award.
Outstanding papers
论文 1:ANALYTIC-DPM: AN ANALYTIC ESTIMATE OF THE OPTIMAL REVERSE VARIANCE IN DIFFUSION PROBABILISTIC MODELS
Author: Fan Bao, Li Chongxuan, Zhu Jun, Zhang Cymbals
Institutions: Tsinghua University, Chinese University, Hillhouse School of Artificial Intelligence, etc
Thesis link: https://openreview.net/pdf?id=0xiJLKH-ufZ
Why: Diffusion probabilistic models (DPM) are a powerful class of generative models that are a fast-growing topic in machine learning. This article aims to address the inherent limitations of the DPM model, which is that the calculation of the optimal inverse variance in DPM is slow and expensive. The authors begin by giving a surprising result that both the optimal inverse variance of DPM and the corresponding optimal KL divergence have their parsed form of a scoring function. They then proposed a novel and elegant training-free inference framework: Analytic-DPM, which uses Monte Carlo methods and pre-trained score-based models to estimate variance and KL divergence in the form of analysis.
This paper is important in terms of both theoretical contributions (showing that both the optimal inverse variance and KL divergence of DPM have an analytical form) and the practical benefits (proposing training-free inferences for various DPM models) and is likely to influence future research on DPM.
论文 2:Hyperparameter Tuning with Renyi Differential Privacy
Written by Nicolas Papernot and Thomas Steinke
Institution: Google Research
Thesis link: https://openreview.net/pdf?id=-70L8lpp9DF
Reason for winning: This paper provides a new insight into an important blind spot in differential privacy analysis of learning algorithms, namely that learning algorithms run multiple times on the data to tune hyperparameters. The authors note that in some cases, some of the data may distort the optimal hyperparameters, thereby revealing private information. In addition, the authors provide privacy safeguards for the hyperparametric search process under the Renyi Differential Privacy Framework.
This is an excellent paper that considers the day-to-day use of learning algorithms and their impact on social privacy and proposes solutions. This work will provide the basis for subsequent work on differential privacy machine learning algorithms.
论文 3:Learning Strides in Convolutional Neural Networks
Written by Rachid Riad, Olivier Teboul, David Grangier, Neil Zeghidour
Institutions: Google Research, etc
Thesis link: https://openreview.net/pdf?id=M752z9FKJP
Reason for winning: This article discusses an important issue for any researcher using convolutional networks, which is to set strides in a principled way that ignores possible experimentation and trial and error. The authors propose a novel, very clever mathematical formula that can be used to learn stride, and demonstrate a practical approach that implements SOTA results in a synthetic benchmark. The main idea in this article is DiffStride, the first downsampling layer with a learnable stride, which allows learning the size of the cropped mask in the Fourier domain, effectively resizing in a way that is suitable for microprogramming.
This is an excellent paper that proposes a method that could become part of a commonly used toolbox as well as a deep learning curriculum.
论文 4:Expressiveness and Approximation Properties of Graph Neural Networks
Written by Floris Geerts and Juan L Reutter
Institutions: University of Antwerp, etc
Address of the paper: https://openreview.net/pdf?id=wIzUeM3TAU
Why: This theoretically strong paper shows how to simplify (sometimes greatly simplifying) the excision and separability of GNN architectures of different graph neural networks in relation to common combinatorial concepts such as treewidth. In particular, this paper proposes that the boundary of the GNN separation force can be easily obtained by the Weisfeiler-Leman (WL) test, which has become the standard for measuring the GNN separation force. This framework also has some guiding significance for studying the approximation of functions through GNN.
By providing a common framework for describing, comparing, and analyzing GNN architectures, this paper has the potential to have a significant impact on future research. In addition, this article provides a toolbox that GNN architecture designers can use to analyze the separation capabilities of GNNs without understanding the complexity of WL testing.
论文 5:Comparing Distributions by Measuring Differences that Affect Decision Making
作者:Shengjia Zhao, Abhishek Sinha, Yutong (Kelly) He, Aidan Perreault, Jiaming Song, Stefano Ermon
Institution: Stanford University
Address of the paper: https://openreview.net/forum?id=KB5onONJIAU
Why: The study proposes a new class of discrepancy that compares two probability distributions based on the optimal loss of the decision-making task. By properly selecting the decision task, the method generalizes the Jensen-Shannon divergence and the maximum mean difference family. Compared to competitive baselines on various benchmarks, the method achieves excellent test performance and has broad application prospects for understanding the impact of climate change on different social and economic activities, assessing sample quality, and selecting characteristics for different decision-making tasks. The review committee found the paper to be of extraordinary experimental significance, as the method allows users to directly specify their preferences when comparing distributions by decision loss, which means that practical applications will be more interpretable.
论文 6:Neural Collapse Under MSE Loss: Proximity to and Dynamics on the Central Path
By X.Y. Han, Vardan Papyan, David L. Donoho
Institutions: Cornell University, University of Toronto, Stanford University
Address of the paper: https://openreview.net/forum?id=w1UbdvWH_R3
Why: The study provides new theoretical insights into the phenomenon of "neural collapse" prevalent in today's deep network training paradigm. During a neural crash, the last layer of features crashes to the class mean, both the classifier and class mean crash into the same Simplex Equiangular Tight Frame, and the classifier behavior crashes into the nearest class mean decision rule.
Instead of employing cross-entropy losses that are mathematically difficult to analyze, the study proposes a new mean squared error (MSE) loss decomposition in order to analyze each component of the loss under a neural collapse, which in turn forms a new "central path" theoretical construct in which linear classifiers maintain MSE optimality for feature activation throughout dynamics. Finally, by exploring the renormalized gradient flow along the central path, the researchers derived precise dynamics that predict neural collapse. This study provides novel and highly enlightening theoretical insights for understanding the experimental training dynamics of deep networks.
Paper 7: Bootstrapped Meta-Learning
作者:Sebastian Flennerhag, Yannick Schroecker, Tom Zahavy, Hado van Hasselt, David Silver, Satinder Singh
Institution: DeepMind
Address of the paper: https://openreview.net/forum?id=b-ny3x071E5
Why: Meta-learning has the potential to enhance artificial intelligence, but meta-optimization has always been a huge challenge to unlock this potential, and this research opens up a new direction for meta-learning. Inspired by TD learning, researchers have proposed a way to guide metalearies from themselves or otherwise updated rules. The study conducted thorough theoretical analysis and multiple experiments to implement new SOTA for model-free agents in the Atari ALE benchmark and improve performance and efficiency in multitasking element learning.
Honorary nominations
In addition to the 7 winning papers, 3 papers were nominated for the Outstanding Paper Award, namely:
提名论文 1:Understanding over-squashing and bottlenecks on graphs via curvature
作者:Jake Topping, Francesco Di Giovanni, Benjamin Paul Chamberlain, Xiaowen Dong, Michael M. Bronstein
Institutions: Oxford University, Imperial College London, Twitter
Address of the paper: https://openreview.net/forum?id=7UmjRGzp-A
提名论文 2:Efficiently Modeling Long Sequences with Structured State Spaces
作者:Albert Gu, Karan Goel, Christopher Re
Institution: Stanford University
Address of the paper: https://openreview.net/forum?id=uYLFoz1vlAC
Nominated paper 3: PiCO: Contrastive Label Disambiguation for Partial Label Learning
作者:Haobo Wang, Ruixuan Xiao, Yixuan (Sharon) Li, Lei Feng, Gang Niu, Gang Chen, Junbo Zhao
Institutions: Zhejiang University, University of Wisconsin-Madison, Chongqing University, RIKEN
Address of the paper: https://openreview.net/forum?id=EhYjZy6e1gJ
For reference:
https://blog.iclr.cc/2022/04/20/announcing-the-iclr-2022-outstanding-paper-award-recipients/