The Heart of the Machine is original
Author: Zenan
HPilot, which has been on the top of intelligent driving in China, has stood first in less than a year.
The speed of autonomous driving is faster than we think: this year, all-scenario assisted driving will land in China.
On April 19, The Company officially launched the "Urban Smart Pilot Assisted Driving System" NOH at the AI DAY event, and is expected to be mass-produced in the middle of the year.
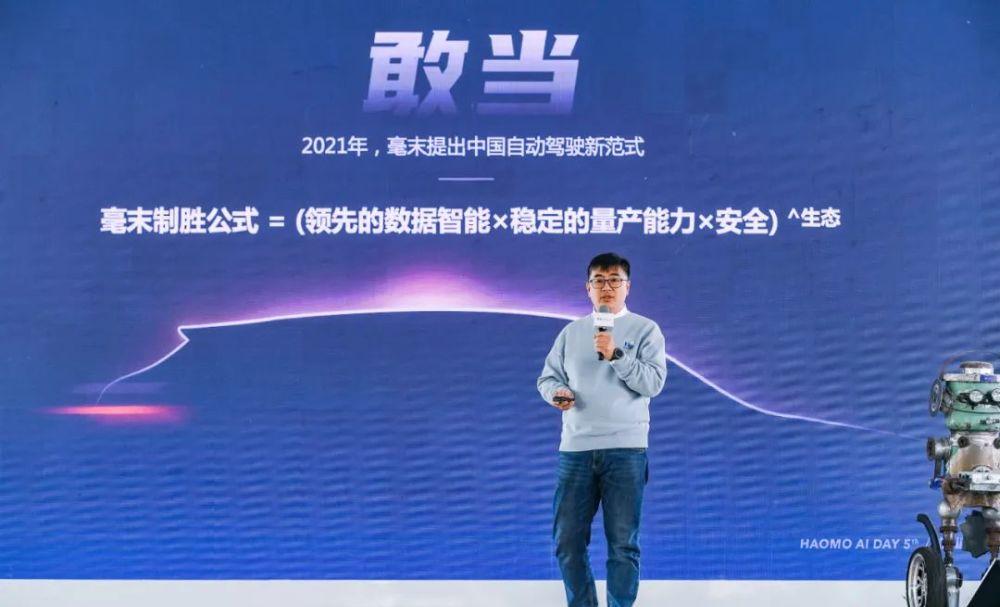
"In 2021, Mo Mo Zhi Xing proposed a new paradigm of china's autonomous driving, that is, the winning formula of Mo Mo Zhi Xing: multiply the leading data intelligence system, multiply the stable mass production capacity by the safety, and then multiply the ecological N power. The winning formula of Miller Wisdom Comes from Miller's deep understanding of the autonomous driving industry and is an important measure for the industrialization of Miller Wisdom's autonomous driving technology," said Zhang Kai, Chairman of Miller Wisdom, at the event.
With the development of technology, high-level intelligent driving is on the eve of mass production. MANA, a self-developed data intelligence system that provides technical power support for all intelligent driving products at the end of the world, is playing a huge role.
In the field of intelligent driving, The company has just released the latest record of total mileage of assisted driving: it has exceeded 7 million kilometers, and the total user use time is more than 130,000 hours.
In terms of the progress of mass production of milliper products, in March last year, Millipede Zhixing demonstrated the HPilot 1.0 system in the brand open day activity, and achieved mass production in May. As of April this year, HPilot has landed on six models of Weipai Mocha, Tank 300 City Edition, Weipai Machiduo DHT, Weipai Latte DHT, Haval Divine Beast, and Tank 500, becoming the most widely used automatic driving system in China.
"In the past year, the basic technology of autonomous driving has undergone a lot of changes, the computing power of in-vehicle chips has been continuously improved, the Transformer transmodal model has been applied, and the camera clarity has increased. Under the premise of the evolution of perception technology, the method of assisted driving is also changing," said Gu Weihao, CEO of Zhixing.
In the urban assisted driving task, MANA's ability from perception to cognition has been greatly upgraded.
MANA Evolution: Learn to look at traffic lights, multimodal Transformers
From highways to urban assisted driving, the complexity of road conditions has multiplied, and getting cars to learn how to look at traffic lights and identify corresponding lane lines is one of the important challenges.
From the perspective of AI technology, this is a small object detection problem: the state of the traffic light will change dynamically, and it has obvious local characteristics, horizontal, vertical, three, five, to be turned, there is a countdown, and there are different kinds. The intelligent driving system must distinguish which line each light specifically indicates.
The solution given by Millima is to accelerate the iteration of the technology through image synthesis and transfer learning, the main challenge of which is to achieve a mix of real data and synthetic data training. Through image synthesis technology, millimeter engineers have expanded the sample size of machine learning and compensated for the imbalance of real-world scene data samples.
In the case of not completely relying on high-precision maps, if you want to successfully achieve traffic light recognition, you need to complete the detection of the lamp type and status, and also complete the binding operation of identifying the target light group in multiple sets of traffic lights in the field of view. Zhixing designed a "dual-stream" perception model for traffic light detection and tying, which decomposes the traffic light detection and tying problem into two channels.
According to Miller, this technology is similar to the visual perception channel of the human brain, in which the ventral stream mainly carries object detection and recognition information, mainly responsible for identifying the pathway (what), dorsal stream mainly carries the information of position and spatial relationship in the field of vision, and is mainly responsible for finding the route (where).
In the dual-flow model, the ventral path is mainly responsible for the identification information of the traffic lights, including the detection of the traffic light box and the classification of the lamp type, and the output of the color, shape and orientation information of the traffic light respectively. The Dorsal pathway is mainly responsible for the traffic light binding, outputting the traffic light group of the target lane, which generates a feature map through training to obtain the position probability of the traffic light position that often appears in the real image.
Subsequently, the model uses a spatial attention mechanism to combine the two, and the dual-flow model outputs the traffic state of the target lane after the tie-in.
After the training was completed, Zhixing conducted a large number of tests on these models, and achieved accurate results in different cities, different distances, different steering targets, and different light conditions.
The Transformer architecture is currently the hottest technology in the field of artificial intelligence. Over the past year, Visual Transformer (ViT) has become a powerful pillar of visual identity. Due to its powerful performance, it has been applied in each individual sensor of autonomous driving.
It has been found that the Transformer structure can bring several advantages to autonomous driving: more efficient use of model volume and data, and the fusion of multimodal data through attention structures, reducing dependence on labeled data.
In the attempt of multi-sensor fusion, cross attention mechanism (Cross Attention) is used as a fusion tool for multi-modal data, which greatly reduces the intervention of human a priori, and can make the combination of optimization-based end-to-end algorithms and data drive more convenient, further realizing the potential of the Transformer architecture.
According to the characteristics of the intelligent driving task, Zhixing proposed its own BEV Transfomer, using the attention mechanism to solve the problem of multi-camera perspective stitching, and made progress in the task of lane line recognition.
Specifically, after obtaining the camera data, the new system first processes the 2D image with Resnet + FPN, and then performs BEV mapping, using Cross Attention to dynamically determine the position of the content in a frame of the image in the BEV space to which the camera belongs. Through multiple Cross Attentions, a complete BEV space is finally formed.
When the visual feature is cast belved, it naturally has the ability to fuse with the LiDAR model. Finally, the algorithm adds time-related features through History BEV to further improve the accuracy and continuity of recognition.
The application of Transformer in autonomous driving is a cutting-edge attempt, and the rewards are considerable. Tesla AI director Andrej Karpathy once introduced the Tesla FSD transformer-based BEV network structure, because the perception results in the BEV space are unified with the coordinate system in which the decision planning is located, so the perception and subsequent modules can be closely linked through the BEV transformation. In addition, the BEV method can effectively fuse the output of multiple sensors, making both large target size estimation and tracking nearby more accurate. The use of this method establishes FSD as a leader in visual perception.
So how effective is the implementation of bev Transfomer? The new method has a higher tolerance for the attitude of the self-driving car, better performance in the longitudinal error of complex road surfaces, and a higher robustness to road surface undulations. In addition, the use of multi-camera output content to assist each other expands the detection field of view, and the automatic driving responds faster to the surrounding things.
At present, in the industry, only Millima Zhixing and Tesla have applied the Transformer architecture on a large scale on autopilot vision. After such perception algorithms mature steadily, they will gradually replace CNN-based perception algorithms.
Realize the "cognitive" ability of autonomous driving with large models
The complex problems faced by urban autonomous driving often exceed the level of perception. On higher-level cognitive issues, there are also some new achievements in the wisdom of the end. For example, when turning left in a very delicate intersection game scene, the auxiliary driving vehicle needs to wait for the U-turn in front of the car, and also observe the avoidance of the opposite straight vehicle and interact with the opposite right turn.
To deal with such a scenario, in the past, the automatic driving algorithm needed to write a lot of regular scene judgments and parameters, and the code was difficult to debug. When there are more and more rules, it will trigger a logic explosion, causing the rules to fail. Millimeter uses machine learning models to replace handwritten rules and parameters for broader applicability.
The TarsGo model proposed by Milli can currently handle many complex assisted driving scenarios, such as roundabouts, auxiliary road entry, pressure change lanes, and so on.
Last year, Ali proposed a 10-trillion-parameter super-large-scale Chinese pre-trained model M6, becoming the first multimodal large model in China to achieve commercialization. Zhixing and Ali Damo Academy collaborated to use M6 to label the automatic driving data for image interpretability, and achieved unprecedented results.
Through the attention mechanism, the AI model can quantify the safety risks with the surrounding traffic participants in a heat map, with the output of Attention High in the close distance and the attention Middle in yellow.
The application of M6 in the field of autonomous driving reflects the universalization of AI capabilities - data that was previously used by other industries can now be iteratively improved for autonomous driving capabilities.
Based on the 128-card A100 cluster, Zhixing also cooperated with Alibaba to implement distributed training of Swin Transformer models, and explored the optimization of mixed-precision training, operators and compilation, which reduced the training cost of large models by 60% and accelerated by more than 96%.
In machine learning tasks, data processing often takes up most of the time. Through the automation of the labeling process, the efficiency automation rate of data labeling has been increased to 80%, which has greatly reduced the cost of automatic driving algorithm training.
Through deep integration with large computing power platforms, the cognitive ability of autonomous driving has been rapidly improved, and these technological advances have finally made urban intelligent driving possible.
HpILOT 3.0, the first mass-produced urban driver assistance system in China, was launched this year
At present, the domestic highway mileage is about 160,000 kilometers, and the urban road mileage has already exceeded 10 million kilometers, and there are 400,000 urban intersections and 1.3 million traffic lights within its range. According to millimae data, 85% of commuting in urban scenarios is congested and semi-congested. Congestion lane changes, detours, intersection games, and the emergence of non-motorized vehicles are the problems that urban assisted driving must face.
The full function development of the city's NOH has been completed, and its data intelligence system MANA has a learning time of up to 197273 hours, and the virtual driving age is equivalent to 20,000 years of human drivers.
The upcoming next-generation urban intelligent driving system HPilot 3.0 will be equipped with a new generation of autonomous driving chips with AI computing power of 360T, cache of 144M, and CPU computing power of 200K+ DMIPS. The whole vehicle is equipped with a multi-redundant perception system formed by 2 lidar, 12 cameras and 5 millimeter-wave radars.
Urban NOH can cope with various complex traffic scenarios in the urban environment according to the navigation route, and realize safe and easy smart travel from point to point in the urban area. Based on current tests, the system achieves a 70% intersection pass rate and a 90% lane change success rate.
In just over a year, Zhixing helped the Great Wall complete the upgrade of intelligent driving ability and took the lead in standing on the starting line of the next stage of urban intelligent driving. In addition to the domestic wisdom of the end, at present, only Xiaopeng has clearly stated that it will assist in driving in the upper-tier cities this year.
The goal set for this year is that the NOH system will cover more than 30 new cars. Over the next two years, the number of passenger cars equipped with millimae assisted driving systems will exceed 1 million. According to this goal, Millima will continue to maintain the first place in China's mass production of autonomous driving in the future.
"With the maturity of autonomous driving and assisted driving, these new technologies can not only effectively protect the life safety of traffic participants, but also gradually release the driving time of drivers, alleviate driving fatigue, and improve the effectiveness of travel," Gu Weihao said.
After large-scale mass production, mana data intelligence, the core of intelligent driving, is bound to set a benchmark in the industry with the accumulation of data and technology.