Xiaopeng in the automatic driving, earned enough eyeballs, but also won the slogan of smart cars, from its Xiaopeng P7 for parking functions, memory parking and share some application innovations in all aspects of the parking route, to now P5 is basically really bought on the market with lidar, and P5 can upgrade multi-layer memory parking, the future to upgrade the city NGP can be described as a flag that has set up a benchmark for automatic driving, making people unconsciously give a thumbs up.
In addition, Tesla is actually the initiator of the main engine factory of visual AI-led applications in autonomous driving, of course, if you delve into the supplier, it may still be Mobileye (from the 2022 CES to see Mobileye autonomous driving product technology and strategy (who said that computing power is the only standard)). Xiaopeng is a domestic follow-up, when Xiaopeng first appeared, Tesla also had a court with its autopilot software, so I believe many people are as curious as I am about this visually dominant autopilot solution and the difference between Xiaopeng and Tesla.
For example
How many cameras does Xiaopeng and Tesla have, and why does Xiaopeng have four more cameras than Tesla? What are the differences between these cameras?
Why is there four cameras under the P7 windshield, while the P5 has only three?
Why Tesla uses a 1.2M camera, the camera resolution is the accumulation of computing power
What is the use of the two lidars of the Xiaopeng P5 (the same can be pushed to the G9)? Urban NGP? Multi-layer memory parking?
Hope to understand through similar questions
How to understand AI image technology simply
The future of autonomous driving and where will the differences be?
About the hardware
The sensors currently applied to intelligent driving are mainly divided into the following four categories:
Ultrasonic sensors, which are currently the most common sensors used for parking, are the same circles that we generally see in front and behind, generally 8-12, for parking to detect whether there are obstacles next to them, which is currently very traditional, and the cost is also very low.
Millimeter wave radar, which is also more common at present, is used for ACC cruise front moving object detection, in addition to placing on both sides of the rear bumper to do similar blind spot detection, rear collision warning and so on.
Lidar, in recent years, China's capital-intensive investment place, due to its high precision, less affected by the environment is currently a very popular sensor for automatic driving, specifically you can see our previous article through the Guangzhou Auto Show - see automatic driving lidar to understand lidar.
Cameras, in fact, is also a very traditional parts, especially mobile phone applications are particularly many, and the development of automatic driving based on visual AI makes it shine on the car, which is also an important reason why the current mobile phone and Internet companies have successively entered the automotive industry, after all, your mobile phone face recognition, the beauty of the mobile phone, and the face change of vibrato. They are essentially similar to the algorithms used in autonomous driving. The cameras currently used on cars are divided into the following according to their position and role.
- Surround view camera, that is, the whole vehicle front and rear left and right four fisheye cameras used to shoot the surrounding environment of the vehicle, this in the domestic application is particularly wide 360 ring view, as well as based on this technology to make out the transparent chassis, etc., based on the surround view camera automatic parking in the past few years very long fire, the current in addition to foreign joint venture brands, the basic domestic main engine factory are equipped with automatic parking assistance based on surround view, because in the eyes of foreigners automatic parking and assistance is chicken ribs, I myself to park, the first does not have this habit of use, Second, there are few such use cases.
-Front view camera, side view camera, rear view mirror camera, this configuration is basically the current intelligent driving for capturing the environment of the camera, front view is generally three, according to the detection distance far, medium, near three cameras; side view is generally one on both sides of the front and rear directions; rear view is used to shoot the rear environment, their design principle is to ensure that the vehicle environment 360 degrees when driving without dead angles to capture, the farther the driving direction to see the better.
- Cockpit camera, arranged in the cockpit to monitor the situation in the cockpit, as in our previous article Smart Cockpit Series Article 1, what is he? describes more intelligent cockpit functions such as driver identification for monitoring driver conditions and expanding the future.
Xiaopeng Car's sensors have changed from P7-P5, in fact, Xiaopeng G3 to P7 and then P5, Xiaopeng's sensors have certain changes, of course, mainly for high-end vehicles. Tesla's sensors are relatively fixed and there are few changes in the middle and high configurations, which mainly reflect its real idea of making money based on software.
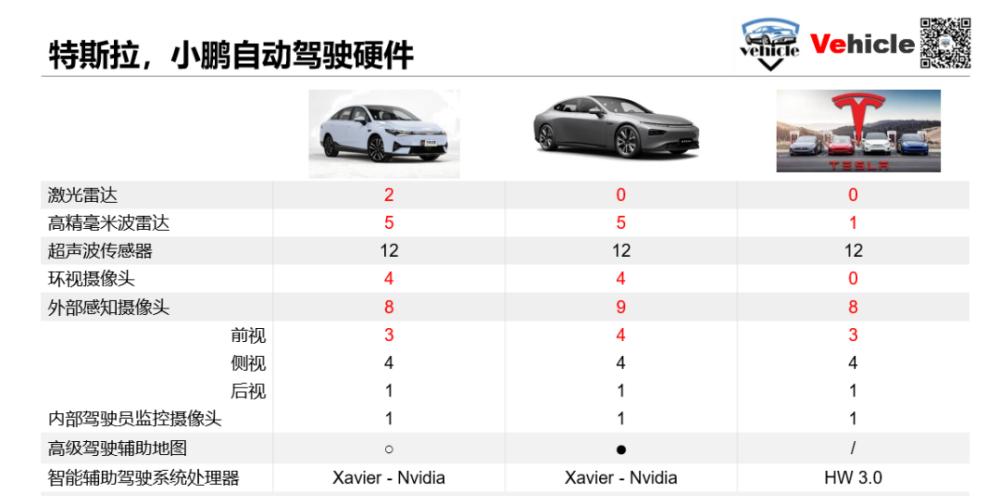
First of all, the camera, let's take a look at the Xiaopeng and Tesla cameras one by one
Surround view camera, first of all, Tesla is no parking assist of the round view camera, it borrows other cameras to help present the rear view, etc., and the Chinese people like to engage in surround view and also use the ring view to engage in innovation. Here we can think of our media teacher clicked a test of automatic parking found that Xiaopeng and many independent brand vehicles can park next to the empty parking space, and our smart Tesla can only use the ultrasonic sensor solution to park a car in the next parking space, of course, I heard that Tesla is testing to find a parking space by vision.
Directly in front of the camera, Xiaopeng P7 has 4 cameras and P5 is 3, and there are messages that they are all 2M pixels, while Tesla also has 3 front cameras, a total of 8 cameras, according to the information of system plus, they are based on the same 1.2 M pixel image sensor released by ON Semiconductor in 2015. They're neither new nor high-resolution, and they're inexpensive, so Tesla genuinely wants to make more money on hardware.
Why Xiaopeng P7 for four front cameras, and to P5 is 3, in fact, it is obvious that P7 on the more may be based on mobileye to achieve AEB and other functions of the camera module, may be P7 at that time its own triple camera development has no information, to P5 may solve the cancellation, save money.
Xiaopeng front camera 2M pixels, frame rate 15/60fps, according to HFOV (Horizon Field of View) is divided into:
HFOV 28: Narrow viewing angle forward camera for AEB (Automatic Emergency Braking), ACC (Adaptive Cruise) and forward collision warning, according to the figure, this camera can be used to focus on road conditions above 150m; it may be 1828 * 948 resolution, 15fps, for long-distance perception;
HFOV 52: Main forward camera for traffic light detection (traffic lights), AEB, ACC, forward collision warning and lane awareness;
HFOV 100: Wide angle of view forward camera for traffic light detection (will look at traffic lights, should be auxiliary main forward camera), rain detection (automatic wiper to rely on it) and anti-jamming (look at a wider angle), guess should be 60fps camera
The Tesla front camera has a resolution of 1280×960 1.2Mp. It provides a forward image capture system up to 250 meters long
The lateral cameras, Xiaopeng's are mounted on the left and right body, are HFOV 100, should be 1M pixels, but the camera in the front direction of the side is 60fps, and the rear of the side is 30fps. The four cameras are actually already usable for 360-degree coverage, and their field of view overlaps a little. Front-view side camera, used for anti-congestion and lateral vehicle detection (another PPT directly said to be anti-congestion camera), resolution 457 * 237, lower resolution can be used to obtain faster response speed; rearview side camera, used for ALC (automatic lane change), door warning and blind spot detection.
Tesla arranged a side-forward-looking camera on the B-pillar, with a sideways rear-view in line with Xiaopeng.
The rear-facing camera, Xiaopeng and Tesla have both placed rearview cameras in the license plate light position, Xiaopeng is an HFOV 52, which should be consistent with the forward-facing main camera, 2M pixels, frame rate 30fps, for ALC, blind spot detection and rear-end warning.
The purpose of the visual scheme for environmental perception is 360-degree coverage, while paying attention to the focus, such as foreground vision, which obviously requires long distances. Below is the Xiaopeng and Tesla Vision FOV diagram.
Tesla and Xiaopeng's front-vision FOV is basically the same, leaving aside the visual distance is not the same, of course, from the figure to see Tesla's front-vision is farther, but the backward visual FOV has some differences, may be the two scheme differences.
Xiaopeng's tail camera has a long but narrow line of sight, while Tesla uses two lateral cameras to cover the rearview scene, while the tail camera has a short but wide line of sight. It can be seen here that Tesla's tail camera is mainly used as a reversing or parking image, and Xiaopeng does not care at all, because it has another set of parking surround cameras.
But in general, the visual 360-degree coverage provides the basis for the AI visual processing of automotive autonomous driving.
About the software
Vision-led, certainly based on camera-based picture processing software technology, the current AI algorithm is the main important application is target recognition, behavior prediction. Tesla, for example, uses CNNs to identify targets, and RNNs to constantly update the map and environment based on kinematic states and perception results, with time domain characteristics. These two words sound quite advanced, but in fact, it is not a new technology, we have used it in our mobile phones.
CNN extracts feature information - such as identifying faces and then wearing various decorations, his first step needs to identify where your head is, your head, nose and then determine the location to carry out various decorations, automatic driving is also used to identify vehicles, pedestrians, bicycles, street signs and so on.
RNN - has a natural way to acquire the time series of images (i.e. videos) and produce state-of-the-art time prediction results, so he can use contextual information to predict future motions such as our commonly used static GIFs. (Sorry, for the RNN application on the static GIF Pirate Jack is not completely sure, but it seems that where to see, if there is a real expert welcome to correct).
So how to understand this AI algorithm? Convolutional Neural Networks – The first problem solved by CNNs is to "simplify the complex problem", reducing the dimension of a large number of parameters to a small number of parameters and then processing them.
He can effectively reduce the dimensionality of large data amounts of pictures into small data volumes, effectively retain the characteristics of pictures, in line with the principles of image processing, and more importantly: in most of our scenarios, dimensionality reduction will not affect the results. For example, a 1,000-pixel picture is reduced to 200 pixels, which does not affect the naked eye to recognize whether the picture is a cat or a dog, and the same is true for machines.
In fact, AI algorithms and the like are not the latest things, it is recommended to read one of the three giants of deep learning, the father of convolutional neural networks Yang Likun's "Scientific Road", AI algorithm development bumpy, in fact, decades ago in research and commercialization, halfway through several twists and turns slow development.
Now AI is so hot mainly due to the accumulation of current Internet big data, so it is conceivable that the future AI technology will be important for data and for network security.
Another piece of software is path planning, which can be viewed in our previous article Introduction to Automated Driving Real-Time Path Planning Algorithms (RRT and Lattice Planner).
And the current algorithm is mainly the application level, so each family is declaring their own algorithm, in fact, the essence does not change much, mainly based on their own data training model is different, another point is the fusion of sensors, there are few pre-fusion programs, the former fusion is to put all the above mentioned sensor information first set unified processing, the current main use of post-fusion, such as visual processing output conclusion, radar output conclusion, lidar output conclusion, Then different weights are processed according to the advantages and disadvantages of each sensor. One of the better things about this approach is redundancy.
About features
The automatic driving function can be divided into functions that respond to three scenarios according to the degree of ease, and then no matter how detailed each is, it is actually based on the micro-classification and expansion of these scenarios.
Low-speed scene - parking, the current most primitive parking mainly relies on ultrasonic sensors, is currently using fisheye camera-based visual fusion schemes, looking for parking spaces and auxiliary parking, while Xiaopeng uses the front camera's Visual simultaneous localization and mapping (SLAM) Most visual SLAM systems work by tracking setpoints through continuous camera frames to measure their 3D position in a triangle, This information is also used to approximate the camera attitude. Basically, the goal of these systems is to associate their surroundings with their own location for navigation purposes.
High-speed scene - high-speed or elevated closed road cruise. This is also a relatively mature industry, mainly based on high-precision maps to implement automatic access ramps, as well as cruising on closed roads. For example, we mentioned earlier (the pioneering place of autonomous driving - logistics and transportation industry) in Tucson and other parts are working hard.
This part is mainly circumvented, some complex traffic participants, and closed ports, similar to the mine scene.
Urban roads - complex scenes. You can look at the planning of each automatic driving, the final difficulty is in this area, here is mainly urban roads, the situation is complex and congested and the speed is medium, and the high speed is covered. The biggest difficulty is the cut in the car and various types of ghost probes, which can understand why the Xiaopeng P5 and G9 are each equipped with a lidar on the left and right of the front, which is mainly to use a more accurate and less environmentally disturbed lidar to detect the cut in and ghost probes that may occur in the advance.
summary
This paper is mainly based on the broad discussion of visual automatic driving solutions based on hardware, software, and functions. In fact, it is very clear that automatic driving should currently form a complete set of tool industry chain led by visual AI, relying on computing power (computing unit click to understand the six mainstream on-board chips and their solutions of intelligent automatic driving), and big data as the base.
Therefore, the difference points in the future of autonomous driving may still return to the subtle experience differences of brand characteristics and personality, rather than various functions.
Of course, the level is limited, the right to throw bricks and jade, welcome to leave a message to learn from each other.
Reference articles
Autonomous DrivingTechnology for Connected Cars - 日立
Developing Autonomous Driving EVs for theChina Market XPENG Motors’ Approach - 谷俊丽
*Reprinting and excerpting without permission is strictly prohibited - how to obtain reference materials: