DNA stores our genetic information, but it's proteins that really perform functions in cells. The amino acid chains of each protein are twisted, folded, and wound into complex structures, and "seeing" their structure is essential to understanding their function. But cracking this structure usually takes a long time, and some are even difficult to complete.
"Using machine learning to study protein structure prediction is in the minority in this field. Until 2016, or even 2018, most people in the field were still trying to study this problem with energy optimization, not machine learning or deep learning. Xu Jinbo, a professor at the Toyota Institute of Computing Technology in Chicago and a visiting professor at Peking University, said in an exclusive interview with the surging news (www.thepaper.cn) reporter.
Xu Jinbo was praised by the industry as "the world's first person in AI to predict protein structure". As early as 2016, the RaptorX-Contact method he developed proved for the first time the feasibility of deep learning methods to predict protein structure, so that protein structure prediction, which has always hovered at the "door", has finally taken a substantial step, and has since set off a boom in AI protein structure prediction.
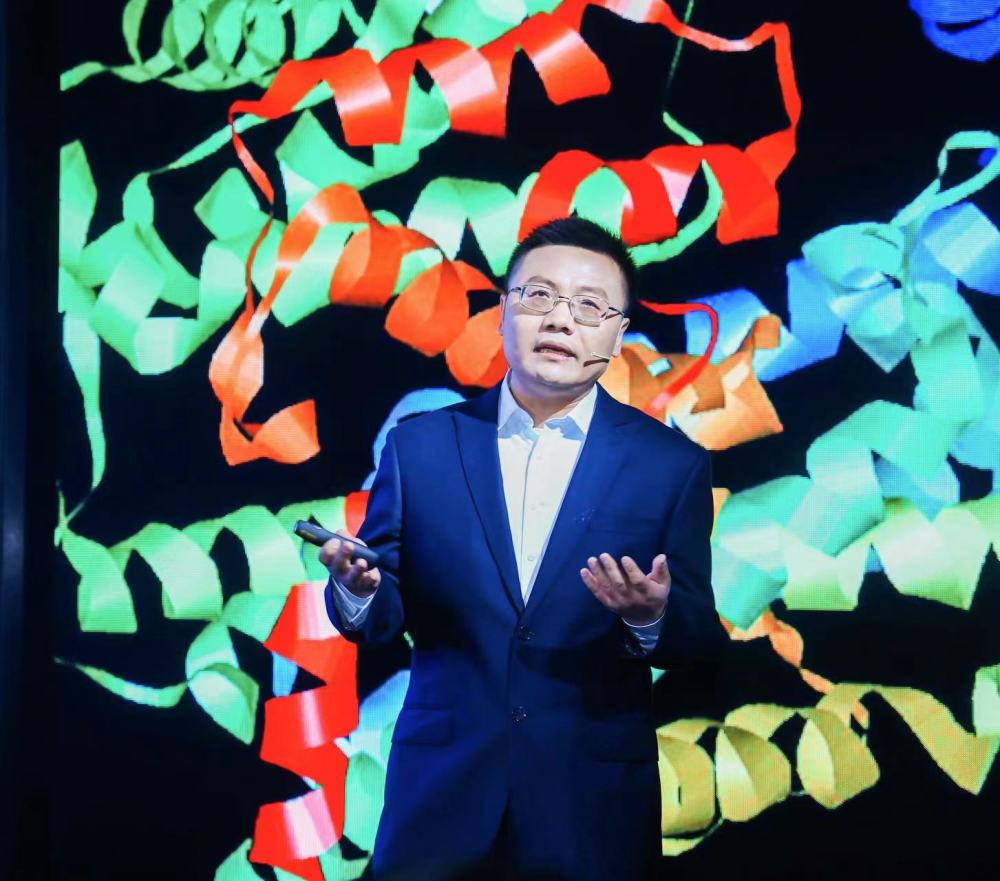
Xu Jinbo, professor at toyota institute of computing technology in Chicago, USA, and visiting professor at Peking University.
The 48-year-old Xu Jinbo has been an out-and-out "school bully" since he was a child. In 1990, at the age of 16, Xu Jinbo won the first place in the Jiangxi Division in the National High School Mathematics League, which was the first time that Linchuan County, Jiangxi Province, had won this category of awards. In 1991, because of his excellent results in the mathematics competition, he was escorted from Linchuan No. 1 Middle School to the Department of Computer Science of the University of Science and Technology of China, and received a master's degree from the Institute of Computing, Chinese Academy of Sciences in 1999. In 2003, He received his Ph.D. from the University of Waterloo, Canada, where he later served as a research assistant professor and a postdoctoral fellow at the Massachusetts Institute of Technology.
In 2001, Xu Jinbo, who was still studying for a doctorate, began to contact computational biology, and his supervisor at that time proposed, "There is a difficult question, that is, to study protein folding, do you want to do it?" Over the next two decades, one of the key topics of Xu Jinbo's research was to develop and optimize software to infinitely narrow the gap between protein structure prediction results and real configurations.
Recently, at the 2022 "Understanding the Future" Science Lecture 01 "AI+ Protein Structure and Function Prediction" hosted by the Future Forum, Xu Jinbo also first talked about the problem of protein structure prediction that has been studied for decades, and in the past this field has been relatively deserted, especially in the 10 years from 2006 to 2016, "At that time, everyone felt that this problem could not be done, so many people left this field to do other problems." ”
Such a desertion is a thing of the past. In recent years, breakthroughs have been made in this area. In 2020, artificial intelligence predicted protein structure was also rated as one of the top ten scientific breakthroughs by the international top academic journal Science Magazine. "Artificial intelligence is now receiving much more attention than it has paid in the past few decades." Xu Jinbo said.
However, Xu Jinbo, who was accustomed to walking on a deserted road, did not show much excitement about the current hilarity. Talking about the companies that have been established in the past two years to apply artificial intelligence to the life science field, he frankly said, "I don't know a lot about the industry, and in recent months I have begun to contact some industry knowledge and investment people." Of course, Xu Jinbo believes that for the industrialization of "AI For science", the current situation is indeed in a relatively good time.
But Xu Jinbo stressed that in terms of artificial intelligence predicting protein structure, the repeated realization of AlphaFold2 of star company DeepMind should not be the goal of other teams, "This improvement is just a gradual improvement, not a very big breakthrough, there are still a series of problems in this field that really need to be solved." For the application of artificial intelligence in life fields such as drug research and development, he said, "I hope to be able to make something really useful." ”
Speculation began half a century ago
Protein structure prediction, which began with a scientist's assumption, is it possible to obtain the three-dimensional structure of proteins without experimentation?
In the decades of protein structure analysis, structural biologists have used X-ray crystallography, nuclear magnetic resonance spectroscopy (NMR), and cryo-electron microscopy (Cryo-SEM) techniques to resolve the structure of many proteins, and to better promote disease mechanisms and drug research and development.
However, these means are seen as laborious and expensive. So far, the structure of about 100,000 proteins has been resolved experimentally, but this is only a small fraction of the 1 billion proteins that have been sequenced.
As a scientist with a computer background, Xu Jinbo understands the protein he has studied for nearly 20 years: protein is made up of many amino acids connected together by chemical bonds, if each amino acid is regarded as a bead, then there are 20 different color beads, these beads are strung together to form a series of amino acids of the protein, each different color is represented by a letter, so the protein amino acid sequence can be seen as a string composed of 20 letters. Each amino acid is made up of dozens of atoms, so the whole protein is made up of thousands of atoms, which interact with each other inside the cell and finally form a stable configuration.
"We can use different software to display these structures, but when using these software to display protein configurations, we need to know the position of these atoms in three-dimensional space, we need to know their three-dimensional coordinates, how can we know these three-dimensional coordinates?" Xu Jinbo mentioned that over the past many years, scientists have developed three main experimental techniques to determine the three-dimensional coordinates of these atoms.
In addition to the three laboratory techniques mentioned above, scientists are also investigating whether the derivation of computational methods is feasible.
Xu Jinbo told the surging news reporter that Christian Boehmer Anfinsen, an American biochemist and winner of the 1972 Nobel Prize in Chemistry, put forward his own conjecture through experiments, "The experimentalist's guess is basically correct, and he himself has done a series of experiments to support this theory." ”
Much of Anfensen's work revolves around the correlation between the structure and function of proteins. In 1961, he studied how RNAases could be refolded after denaturation, returning to their original spatial structure while retaining the enzyme's activity. Anfinson therefore argues that all the protein information needed to create the final conformation is encoded in its amino acid sequence, i.e., the protein's first-ordering determines the three-dimensional structure.
This is known as Thermensee's Law, which is the cornerstone of protein structure prediction.
American biochemist and 1972 Nobel Laureate in Chemistry Christian Anfensen.
However, for more than 50 years, scientists used a variety of methods to accurately calculate the three-dimensional structure of proteins. "Under the assumption and theory of Anfinson, scientists do protein fold predictions from the perspective of energy optimization." Xu Jinbo explained that it is generally believed that proteins are folded to the minimum energy state, which also means that, in theory, if this energy function can be better optimized, the minimum energy state of the protein can be found.
But this line of thinking has a natural flaw. "First, a protein is a very large system, made up of thousands of atoms, corresponding to a very large search space, and the configuration is kaleidoscopic." Xu Jinbo went on to raise the second difficulty, "Although it is generally accepted that proteins are folded to the minimum energy state, what is the energy function? Our own understanding of the energy function is not particularly good. ”
Xu Jinbo initially used traditional optimization algorithms to study this problem at the doctoral stage. In 2001, he took over the topic that his mentor threw at him, and the next year he achieved good results, winning the 2002 global protein structure prediction competition, CASASP (for fully automated high-throughput protein structure prediction).
Recalling the results at that time, Xu Jinbo slightly downplayed, "Although the ranking is the best, the significance is not so great, and it does not change the status quo of this problem, but the result is a little better than others." After continuing this line of thinking for more than a year, he realized that traditional optimization algorithms might not be a good path.
In 2006, Xu Jinbo began to turn to machine learning, and he had formed an independent laboratory at the time, believing that the strategy should be changed. "We did a little better with machine learning than the traditional method, and in the protein structure prediction competition, we also achieved very good results, a little better than other groups, but there was no particularly big change."
This path will be another 8 years, which should also be the most deserted 8 years on Xu Jinbo's scientific research road, many people have changed careers, and there is almost no attention in the field.
Why AI can succeed
In 2014, Xu Jinbo began the second conversion route.
"In 2012, deep learning began to achieve very good results in image recognition, so we began to try to study this problem with deep learning in 2014." The real incorporation of AI into Xu Jinbo's toolbox for predicting protein structure began this year. At that time, only a very small number of people in the same field were paying attention to this new tool.
"The new method is not to optimize energy, but to predict the interaction relationship between atoms."
Xu Jinbo further explained that if there is already an amino acid sequence, then find out those proteins that are homologous to this protein (the same family), and then compare the amino acid sequences of all these proteins of the same family together. "Under the multi-sequence comparison, we use the matrix to represent the interaction relationship between amino acids in the protein, and then according to the interaction relationship matrix, we can predict the coordinates of the atoms of the protein, which is the general idea of this new method."
Of course, there can be different implementation methods within the framework of the general idea, "but the key point of the new method is whether we can accurately infer the interaction relationship between atoms or amino acids in proteins, this step is very critical." ”
Xu Jinbo said that in order to predict the interaction relationship between atoms, the earliest method explored by scientists is the global statistical method for co-evolution analysis. However, this method is only effective for a very small proportion of proteins, and often the three-dimensional structure of some proteins in these protein families has been measured by experimental techniques, which means that the prediction with this method is not too significant.
He believes that the turning point in the real prediction of the role of a large number of protein structures is 2016. After turning to deep learning for 2 years, Xu Jinbo began to use deep learning to predict the three-dimensional structure of proteins. In the previous 2 years, the team started with a simpler problem, trying to predict the secondary structure of the protein, that is, the spatial placement of the atoms of the peptide chain backbone skeleton, without involving amino acid residue side chains.
"For such a simple problem to be done well, we think it should be effective for the more difficult problem, which is to predict the three-dimensional structure of proteins." Xu Jinbo mentioned a detail that in 2015 he organized students to solve the problem of three-dimensional structure, but it did not come to fruition, "They did not understand my idea very well, because at that time no one in this field used deep convolutional networks to solve this problem." ”
In 2016, Xu Jinbo, who had set aside some time, began to write his own code to implement his own algorithm, "Probably in the summer of that year, I got very good results, and found that I could do much better than the previous method, and in the fall of 2016, I wrote the results as a paper and posted it on the Internet." "The first month after the release caused a wave of attention in the field.
Xu Jinbo released the first generation of artificial intelligence method RaptorX that he developed. The basic principle of this method is that through the deep convolutional residual network (ResNet), the sequence of proteins is convoluted to extract valid information, and the interaction relationship between protein residues is also convoluted. Through the different convolutional transformations of the two, the interaction relationship between protein amino acids can be predicted very accurately. "Then based on this interaction relationship, we can reconstruct its three-dimensional structure."
In the 2016 Global Protein Structure Prediction Competition (CASP12), this method, which had not yet been perfected, came to the fore, "at that time it was already doing very well, doing better than other traditional methods." ”
In January 2017, Xu Jinbo officially published his previous results in PLOS Computational Biology, entitled "Accurate De Novo Prediction of Protein Contact Map by Ultra-Deep Learning Model". In this paper, the research team shows that by using deep residual convolutional networks, the accuracy of protein prediction can be greatly improved, and this learning method can also be easily generalized to different types of protein levels, such as some membrane proteins and protein complexes.
To this day, this is still Xu Jinbo's most satisfactory paper. "After our paper came out, we actually defined the problem very clearly. From the perspective of AI, it is to tell everyone what the input and output of this problem are, and you just need to do a good job of the AI algorithm. As for what AI algorithm you use, it is nothing more than a matter of engineering and computing resources. ”
He also recalled to The Paper a small episode in which the research team actually initially submitted the paper to a sub-journal of Nature, but the editors were not too convinced of their results. "Because we've been working on this issue for years, and there's been no progress, he doesn't think we've done this well, and a judge in another journal doesn't think our results are reliable."
To Xu Jinbo's relief, both academia and industry have paid extensive attention to the research after the paper was published. He felt that, in general, people with computer backgrounds were more receptive to their results, while those who studied physics or biophysics were not very receptive to the results because they had not been accustomed to using similar methods before.
It is worth mentioning that in the past 30 years in the field of protein structure prediction, the development of this field can be roughly divided into three stages. The first stage, that is, for more than 20 years, has been very slow in the field under the traditional method; the second stage, that is, the use of raptorX, the first generation of artificial intelligence method developed by Xu Jinbo and others, has greatly improved the accuracy of predicting the structure of the more difficult proteins; and the third stage is the world's best-performing protein structure prediction tool so far, that is, DeepMind's AlphaFold2 launched in 2020. "By using a network of attention mechanisms, the accuracy of protein structure prediction can be greatly improved."
In Xu Jinbo's view, DeepMind was actually re-implementing his algorithm in 2017 and 2018, "Of course, they are doing a better job in engineering than us." For deepMind's attention mechanism network in AlphaFold2, it was first applied to natural language processing.
"People in computational biology don't know a lot, and the first people to really use this network in this field was Facebook, which they didn't use to make protein structure predictions, but to model protein sequences." Xu Jinbo mentioned that even if people in the field of computational biology later noticed the network based on attention mechanisms, the network needed too many computing resources, "no one in academia has so many resources to do this." ”
Xu Jinbo admits that his team in 2020 once considered how to simplify the network based on the attention mechanism, "hoping to make it run on our computing resources, which is what I did at the time, because we didn't have hundreds of GPUs (chips on graphics cards)." In contrast, DeepMind, backed by Google, has no "resource dilemma" at all, and can train their models with a lot of GPU cards.
Xu Jinbo believes that from the perspective of ideological innovation, this step taken by AlphaFold2 is not without people being very surprising. "What's really amazing is that they were able to mobilize 30 people at once to do this thing, and they were able to achieve it very well, and I think that's their strength."
Overall, AI has played a very big role in the field of protein structure prediction, and why has only deep learning been able to do it for so many years?
Xu Jinbo shared his personal understanding, the first premise is that deep learning is based on existing theoretical foundations, especially evolution. "First, although we don't have their configuration, we know that the protein structure of the same family should be very similar. Second, it is also very important that adjacent amino acids in the same protein interact with each other and co-evolve. ”
In addition to the theoretical basis, Xu Jinbo believes that data is of course indispensable for training deep learning algorithms. "Now that we have a lot of protein sequence data, it's important to infer the distance of atoms in space based on the evolutionary relationships of proteins in the same family." Another important data source is that we also have some protein structure data, although not so much, but now we have at least some, so by guiding deep learning models to learn the relationship between amino acid coevolution and the middle distance between atoms. ”
Something more important than implementing AlphaFold2 repeatedly
Especially after the emergence of AlphaFold2, the field of artificial intelligence predicting protein structure has received unprecedented attention and has finally "become lively".
Xu Jinbo concluded that artificial intelligence has indeed subverted protein structure prediction, and this will bring about very big changes, especially for molecular biology, "I think this result has now changed the research paradigm of many molecular biologists, the previous molecular biologists basically based on the amino acid sequence of proteins to analyze the function of proteins, and now many people have begun to use predicted structures to do research and analyze the function of proteins, so this is a very big change in research paradigm." ”
But now that the end is far from being reached, how can we continue to advance the application of artificial intelligence in structural biology and even broader biology in the future?
Xu Jinbo said that there are many teams working on repeated implementation of AlphaFold2, "Of course, this is a necessary path, but this improvement is only a gradual improvement, even if we can do a little better, it is not a very big breakthrough." He also cautioned that if a lot of teams or startups rush to do this, "I think it's a bit of a waste of resources." ”
In his view, those problems that are not solved well enough at the moment need to really invest more energy.
For example, can we make very accurate predictions about an orphan protein? Is it possible to predict the folding process of proteins, not just the final configuration? Can you accurately predict the structure of a protein complex or a multidomain protein? Is it possible to predict the interaction of proteins with peptides, DNA or RNA? Is it possible to predict the effect of single or multipoint mutations on the structure and function of a protein?
He further told The Paper that our requirements for protein structure prediction depend on our goals. If the goal is simply to know the final three-dimensional shape of the protein, for most proteins it has already done so. "But what we can do now is predict the structure of individual proteins very well." But for more complex cases such as protein complexes, artificial intelligence methods can indeed do much better than before, but they have not yet reached a very satisfactory state, and this direction still needs to spend more time to study. ”
Xu Jinbo also threw out a more worthy question, "Now all the successful methods are actually a bit of a rating. "It's also a problem in principle.
It's not hard to understand why this is so, because current methods require the use of a lot of protein homology information, "the more homologous proteins can be found, the better this prediction." Without this part of the information, all methods are now ineffective. Xu Jinbo said that in the cell, that is, when the proteins in nature are folded, "it does not know what proteins are in the same family, it can fold themselves, it does not need to know how many 'brothers and sisters'." ”
It is worth mentioning that Xu Jinbo has returned to China and decided to shift his focus to China. "The innovation-driven development strategy is a strong guarantee for the development of our country's comprehensive national strength," Xu Jinbo told the surging news reporter, "I hope to do something truly original and can be landed, and promote the integration and development of scientific research and industrialization." ”
Speaking of the industrial application value of "AI + life science", Xu Jinbo said that the current industrialization environment of "AI for Science" is very good, especially "AI for BioTech". "The state attaches great importance to the field of 'AI for BioTech', and investment institutions are also very supportive of early and long-term investment in the field of hard technology." From the perspective of the industry, he believes that because AI empowers all aspects of the biopharmaceutical field and helps the industry improve efficiency and accuracy, the industrialization of AI in this field also has good prospects.
It is worth noting that in January this year, Xu Jinbo founded Beijing Molecular Heart Technology Co., Ltd. (hereinafter referred to as "Molecular Heart") in Beijing. Just in April, the company announced that it had completed tens of millions of dollars in angel round financing, led by Sequoia China, followed by Baidu Venture Capital, Life Park Venture Capital, NeuX Capital, and Future Qichuang Fund. Heart of Molecule said the round of funding will be used to further expand the team, the continuous evolution of the AI protein platform, and the productization of scientific research results.
He told the surging news reporter that the company currently has only a small team to continue to study the problem of protein structure prediction, "our main goal is whether we can do the optimization and design of various proteins." For example, an antibody can be optimized better so that it can bind better to the antigen; or whether it can be designed to be a protein that does not exist in nature and used for medicine or other purposes; or whether an enzyme can be optimized better. This is the focus of our company now. ”
Finally, it is said that the integration of multiple disciplines is more important than ever, and how to attract more people to join the interdisciplinary discipline and attract more students into the field still faces some challenges.
Xu Jinbo said with his own experience, "When I first entered the field of computational biology, I found that my communication with biologists was actually very difficult. Only after a period of time can the conversation and cooperation continue, and I think it is very important to communicate more. ”
More critically, he believes that the evaluation system should make some changes. "From my experience, making protein structure predictions or doing computational biology has not received much attention before. Previous papers have not been published in particularly high impact factor journals, and the impact factor is related to how many people are doing it in this field. If you use impact factors to evaluate a computational biology job, often these people are at a disadvantage, and in turn suppress the students who do computational biology. ”
Xu Jinbo's view is that everyone should be more open-minded and tolerate the development of people in different fields.