With the deepening of national data strategies, data has become an element affecting socio-economic development. At the same time, with the accelerated implementation of new governance regulations such as the Data Security Law and the Personal Information Protection Law, balancing data utilization and security compliance has become an important direction for the construction of data resources. Driven by the dual drive of data fusion applications and privacy protection, the private computing boom has risen rapidly.
However, at present, the privacy computing industry is still in its early stages, its commercialization is facing four major challenges of ecological barriers, computing performance, security and availability, the market environment and commercial scale are not mature enough, what is the commercial road ahead of privacy computing?
"Combined with the development process of AI, the future development of privacy computing can learn from two major experiences," Xu Shizhen, chief architect of RealAI of Ruilai Wisdom, put forward the idea of responding to the challenges facing privacy computing at the Data Security and Privacy Computing Forum with the title of "Privacy Computing Helps Build a New Infrastructure for AI".
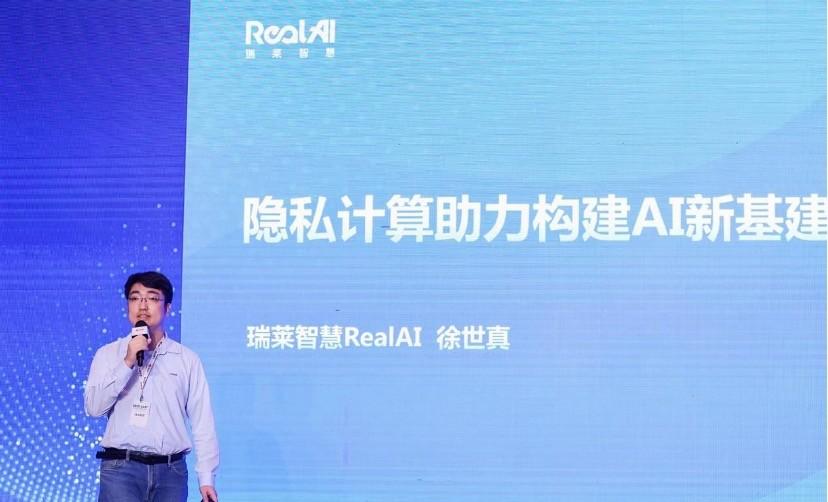
Privacy computing has opened up a new data collaboration model, under the premise of not leaking the original data information, the data is analyzed and calculated, the separation of data ownership and use rights is realized, and the loss of data assets and privacy information leakage in the circulation process is avoided. The data circulation 1.0 stage transmitted directly from plaintext, the privacy calculation mode is the data circulation 3.0 stage.
Founded in July 2018, Beijing Ruilai Intelligent Technology Co., Ltd. is a scientific and technological achievements transformation enterprise initiated by the Artificial Intelligence Research Institute of Tsinghua University, which is committed to providing safe and controllable artificial intelligence infrastructure platforms and solutions. Zhang Cymbal, Academician of the Chinese Academy of Sciences and Honorary Dean of the Institute of Artificial Intelligence of Tsinghua University, and Zhu Jun, Professor of the Department of Computer Science of Tsinghua University, jointly served as the chief scientist of the company, and Tian Tian, Ph.D. of the Department of Computer Science of Tsinghua University, served as CEO.
Starting from the current problem, in Xu Shizhen's view, the commercialization of privacy computing at this stage still faces four major challenges.
First, ecological barriers. At present, the privacy computing technologies of various manufacturers are not interconnected with each other, nor can they be connected to each other, and the process of solving the problem of data islands will bring about the problem of technical islands, which means that a lot of integration is required at the upper level.
Second, computational performance. The introduction of cryptographic operations, distributed communication problems, and homomorphic encryption have led to slow computing performance, which is difficult to support large-scale data training.
Third, security. From the perspective of intellectual property protection, each manufacturer will not disclose the underlying agreement, resulting in the problem of opaque agreement and difficult to audit.
Fourth, availability. The current privacy computing technology service providers do not have the ability of data ecology and data linking, and cannot provide out-of-the-box data and solutions, and the application cost and difficulty of users increase.
Based on the recognition of these challenges, what are the lessons for the future development of private computing?
Xu Shizhen first mentioned the technical path, "the compiler route based on the underlying data flow graph will promote the compatibility and interoperability of the technology; performance optimization can be achieved by optimizing the underlying cryptographic library at present, and new hardware will still need to be used in the future; and the security needs to be protected against malicious attacks at the cryptographic protocol layer and the application layer."
Secondly, in terms of industrial path, Xu Shizhen believes that private computing needs to be implemented on a scenario-by-scenario basis, and appropriate technical routes are adopted according to different scenario requirements, such as multi-party secure computing efficiency, security can be proved, but the traffic volume is large, only simple computing logic is supported; federated learning supports complex machine learning, but mainly for modeling scenarios; TEE route has better performance and algorithm ecology, but relies on hardware vendor hardware credibility and user acceptance of data centralized processing.
Specifically, Ruilai Wisdom's solution idea is to create an integrated privacy computing solution of "platform + data + service + scenario" for scenario requirements, introduce dozens of external data sources such as operators and payments, promote privacy computing from the functional demonstration stage to the closed loop of business landing, and realize the rapid empowerment of different business scenarios such as finance and government affairs.
Rayleigh wisdom launched the industry's first compilation-level privacy protection computing platform RealSecure, which takes the compiler architecture and full homomorphic encryption as the core breakthrough, and realizes automatic compilation and one-click adaptation with traditional algorithms. At the same time, based on the underlying data flow diagram, and the construction of a comprehensive security assessment system before, during and after the event, to achieve a traceable and verifiable high security level.
Privacy computing is an important complement and extension of AI capabilities
Privacy computing is often closely integrated with AI, and Xu Shizhen said that from a technical point of view, private computing is an important supplement to AI capabilities. AI is highly dependent on data foundation, large-scale and diverse high-quality data, can train better performance models, private computing by solving the "link" problem of data, providing data supplement for the continuous evolution of algorithms.
Correspondingly, this also forces enterprises to increase the expansion of data in the process of landing AI applications. But as more and more data is collected and utilized, data risk and privacy protection have also become a challenge for AI systems in the development and application process. On September 26, the National New Generation Artificial Intelligence Governance Professional Committee issued the "New Generation Artificial Intelligence Ethics Code", in which data and privacy security content runs through the specific ethical requirements of specific activities such as artificial intelligence management, research and development, and supply.
At the product level, low replication and poor versatility are a major limitation of the current productization of privacy computing. Xu Shizhen provides two solutions, one is to try to start from the requirements of mature specifications and less customization, and the other is to embed privacy computing into existing mature products, such as privacy protection databases and privacy protection big data analysis engines. Under the latter idea, in combination with AI technical capabilities, privacy computing can be seen as AI middle platform 2.0, that is, adding a privacy computing function module to the original machine learning platform.
"This is also the user's ideal form of privacy computing products, the external still output AI modeling capabilities, the user operation level is almost indifferent, while using the original machine learning modeling technology, the underlying layer has achieved privacy protection functions through cryptography and MPC technology." Xu Shizhen said.
To some extent, AI can also be seen as an upper-level application of privacy computing. Xu Shizhen introduced that there is currently no universal solution for scenarios for privacy computing, and a single technical route cannot be adapted to all scenarios. In practical applications, privacy computing cannot be decoupled from upper-level applications, nor can it be decoupled between different technical routes, in most cases, users still need AI-related functions, and AI has thus become a core requirement for driving privacy computing.
Privacy computing is just one part of the corporate compliance building
Privacy computing is not just a technical act, but also a corporate compliance organization building act. However, in the early stages of the market, there are often misunderstandings in the user's understanding of the application mode and scenario of private computing. For example, in terms of compliance, enterprises often want end-to-end security, full-process security in line with legal norms in the public sense, including data collection, anonymization, and use authorization mechanisms. However, private computing only solves the security problems in the process of data circulation and model training/prediction, and there is a deviation from the user's expectations.
Xu Shizhen stressed that privacy computing is only a part of corporate compliance construction, which needs to be carried out within the framework of laws and regulations. At present, Ruilai Wisdom and Zhong Lun Law Firm have launched strategic cooperation, giving full play to the resource advantages of both parties, applying the construction of compliant legal and technical systems with the strong regulatory requirements of regulatory agencies in the era of digital economy for new scenarios such as artificial intelligence and data exchange, and providing consulting services and system construction services for enterprises.
On the other hand, Xu Shizhen said that although policy supervision has been introduced, it is often difficult for enterprises to simply pay for the safety input of "cost items". In essence, while privacy computing solves the problem of data being "able to" come out, it does not solve the problem of the willingness of enterprises to share data.
The key here is the closed loop of data value, fully release the value of data, so that each data participant benefits from it, and transforms the "cost item" into a "revenue item", so that users can have a degree of continuity, open the specific use rights of data to participate in subsequent data circulation, and promote continuous data circulation. This process usually requires the cooperation and confirmation of the advantages of the business units within the enterprise.
Xu Shizhen said that AI technology can effectively solve the problem of willingness to circulate data. AI technology has strong data processing and analysis capabilities, which is the key technology to realize the value of data, and privacy computing solves the problem of safe data circulation. Through the in-depth combination of "artificial intelligence + privacy computing", it is possible to achieve in-depth mining and release of data value on the basis of realizing cross-industry and cross-domain data security integration.