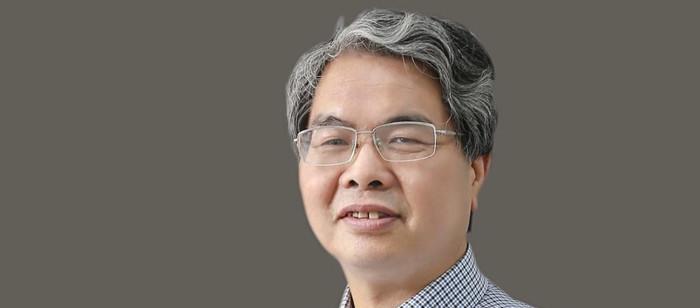
Author | Don
Edit | Wang Ye
Promoting cognitive artificial intelligence requires not only "perception" but also "cognition".
At the 2021 Chinese Intelligence Conference (CCAI 2021), Academician Jiao Licheng made a speech with the theme of "Challenges and Thinking of Brain-like Perception and Cognition?" Academic reports.
This report first reflects and sorts out the development process of artificial intelligence, and on this basis, it summarizes the achievements of its research group in the corresponding fields in recent years for the three themes of cognitive modeling, automatic learning and gradual evolution.
Jiao Licheng, doctor of engineering, professor, doctoral supervisor, foreign academician of the European Academy of Sciences, foreign academician of the Russian Academy of Natural Sciences. His research interests include intelligent perception and quantum computing, image understanding and object recognition, deep learning and brain-like computing. He is currently the director of the Department of Computer Science and Technology, the dean of the Institute of Artificial Intelligence, the director of the Key Laboratory of Intelligent Perception and Image Understanding of the Ministry of Education, the member of the Ministry of Education Science and Technology Committee, the expert of the Artificial Intelligence Science and Technology Innovation Expert Group of the Ministry of Education, the chairman of the "Belt and Road" Artificial Intelligence Innovation Alliance, the chairman of the Shaanxi Artificial Intelligence Industry Technology Innovation Strategic Alliance, the 6th-7th vice chairman of the Chinese Engineering Intelligence Society, and the IEEE/IET/CAAI/CAA/CIE/CCF Fellow, who has been named to elsevier's list of Cited Scholars for seven consecutive years. The research achievements have won the second prize of the National Natural Science Award and more than ten scientific and technological awards of the first prize or above at the provincial and ministerial levels.
The following is the full text of the speech, which has been collated without changing the original meaning.
At present, neural networks and deep learning are developing rapidly, and in this emerging field, the most core and compelling direction is the optimization method of networks.
This article will be divided into five parts: the relationship between artificial intelligence and deep learning, post-deep learning - cognitive modeling, automatic learning, gradual evolution, and summary.
1
Artificial intelligence and deep learning combing
Artificial intelligence has been born more than 60 years ago, at the Dartmouth Conference in 1956, mcCarthy, Minsky, Rochester and Shannon and other scientists first proposed the term "artificial intelligence", thus marking the official emergence of artificial intelligence as a science, and clarifying its complete academic path, but also marked the official birth of a new field of artificial intelligence.
Not only did they give birth to the concept of artificial intelligence in their discussions, but their forward-looking work also had a profound impact on future generations, especially in the IT field.
According to its history of natural development, ARTIFICIAL intelligence can be divided into four stages: expert systems, feature engineering, speech image and word processing, and the current stage of technologies represented by augmented learning, adversarial learning, self-supervised learning, meta-learning, and reinforcement learning.
In the expert system stage (1960-1980), artificial intelligence is relatively rudimentary, and the main technology relied on is the manual design of the rules. At this stage, people mainly hope that artificial intelligence systems can do search work.
In the feature engineering phase (1980-2000), people began to process raw data to extract features, and used simple machine learning models for tasks such as classification and regression.
In the third phase (2000-2010), people began to process natural information such as speech, images, and words. In this phase, the AI system feeds raw data and answer labels into the deep learning model. However, machine learning models based on the traditional binary string structure at that time could not learn such a complex system to complete the corresponding complex tasks, so AI entered the next stage.
In the fourth phase (2010-2020), people give data to machines, hoping that machines can automatically mine the knowledge contained in the data. However, in practical applications, the system still relies on humans to organize and arrange models and data, so as to guide the model to dig for knowledge. Although we hope that AI models can automatically mine knowledge, the successful operation of models is difficult to leave human supervision and guidance.
In this brilliant fourth phase, a variety of fields such as machine proof, machine translation, expert systems, pattern recognition, machine learning, robotics and intelligent control are produced. Although their cores are different, they are all an indispensable and important part of the fourth phase of AI development.
In addition to combing through artificial intelligence from time, we can also divide artificial intelligence into five academic schools according to its core ideas: symbolism, connectionism, behaviorism, Bayesian school and analogy school.
In the early stage of the development of artificial intelligence, there was not much integration and reference between these five schools, but they all worked hard, worked independently, and were full of confidence in their own application fields.
Today, we find that these schools actually explain artificial intelligence and machine learning from their own perspectives. The development of artificial intelligence needs to integrate these five university schools into each other.
The winner of the IEEE Neural Network Pioneer Award attests to the development of artificial intelligence:
Shun-ichi Amari proposed the kinetic theory of neural fields, especially in the field of information geometry.
Paul J. Werbos, who graduated with a Ph.D. from Harvard University in 1974. Werbos mainly established and proposed the backpropagation algorithm BP, and it can be said that Werbos was the first person to BP algorithm. Even Geoffrey Hinton, who has a deep influence on the popularity of deep learning today, was a member of the BP algorithm team that worked with Werbos at that time, and he also made many contributions to the widespread use and dissemination of BP algorithms.
Leon O. Chua is a Chinese-American scientist who is regarded as one of the big three in the EE field at the University of California, Berkeley. He created the high-order original of nonlinearity, proposed Chua's circuit, promoted the development of nonlinear circuit theory, set off a boom in the study of nonlinear circuits, and made outstanding contributions to the transition of chaos from theory to practice. In addition, he also proposed the CA cell neural network, which has a huge influence in the world and is the pride of the Chinese. Many magazines have introduced his scientific discoveries such as CA and chaotic circuits. To this day, he is still active in the front line of Sino-US scientific exchanges.
Fukushima is the initiator of neurocognition. Oja is a Finnish scientist and the originator of "subspaces". Teacher Yao Xin's contribution to evolutionary computing is enormous. Professor Wang Jun has also made important contributions to the research of neural networks. LeCun won the award in 2014 for its convolutional neural networks proposed in 1990-1992; Bengio later won in 2019. This year's winner is Professor Liu Derong of Guangdong University of Technology, who is also the former editor-in-chief of IEEE Trascations on Neural Networks, TNN.
After 70 years of development, neural networks have also entered a new stage. Technically, he is different from the traditional way of pure "feeding data". In fact, from a macro perspective, simple data training methods based on BP algorithms have become a thing of the past.
Nowadays, we are faced with scenarios and problems such as the expression, learning and interpretation of massive, noisy, small sample, non-stationary, and nonlinear data. This is very different from the traditional method.
Artificial intelligence has experienced a new stage from "feature engineering" and "feature search" to the current "representation learning" and "learning to understand". This brings a new paradigm to the field of computer vision driven by characterization learning, recognition, and optimization.
The learning of neural networks involves many factors. The most fundamental of these is the study of scientific problems; the other is to learn the understanding of theory, including representation theory, optimization theory, and generalization theory. The algorithm base is not only the network model structure itself (such as CNN, self-editing, RNN, GAN, Attention and other deep learning structure combinations), but also the mechanism behind it, biological mechanism, and physical principles. Of course, it also includes computational methods to improve the effectiveness and feasibility of algorithms and online processing.
The optimization method of the model plays a very important role in the neural network. Optimization is not only a system development based on traditional gradients. Among them, the most widely used naturally inspired planning algorithms such as global Darwin and local Lamarck are used, but this type of algorithm faces many problems, such as randomness, orthogonality and convergence. Of course, from the perspective of the data foundation itself, the system is also facing problems such as the expansion of data matching, the adaptive processing of the field, and the normalization. In addition, we now have a lot of strong platform infrastructure technologies such as Pytorch, Tensorflow, Keras, and Caffe.
However, deep learning also faces many difficulties, including inherent defects in its own theory or technology (nonlinearity, small sample size, noise, etc.), and the open environment for real-world artificial intelligence problems. These bottlenecks need to be addressed theoretically. First of all, we need to study the elaboration method of the problem to solve the problem of unclear relationship between features and decisions and the priority of interpretation; in addition, we also need to solve cognitive defects, that is, concept abstraction, automatic learning, progressive learning, intuition and forgetting; of course, in the bottleneck problem of learning, mathematical problems such as convergence consistency, stability, and gradient stationary properties also need to be overcome.
Currently, researchers do not have systematic theories and solutions to interpretability.
We can divide interpretability studies into three categories:
First, we can understand and elaborate on the distribution characteristics of the data before modeling.
Second, we can explore the interpretability of the model by establishing rules.
Finally, we can effectively systematically study and explain the actions and functions of the model (including the biological and physical mechanisms of the model) after modeling, which is a more macroscopic approach.
Theoretical flaw: instability
In the problem of unstable gradients, the problems of gradient disappearance and overfitting have long plagued artificial intelligence algorithms. We usually solve it by formulating methods such as loss functions and norms, but the problem is not completely solved because of this. Neural networks have long-term memory and short-term memory, so it also has the problem of catastrophic forgetting. The theoretical characterization, learning methods, selective forgetting, and design of dynamic spaces for these catastrophic forgetting are also important topics.
Neural network models that people design and deploy run and work in complex environments where human involvement is involved. As a result, they operate in an open and dynamic environment where multiple attacks (black box, white box, gray box) can exist.
Then its security is a big problem. Therefore, the self-defense of neural networks in the anti-attack environment is also an important topic.
The benefit ratio of an algorithm (i.e., the cost of deployment) is an important issue to consider before deployment. We want to design a green, resource-optimized hardware and software environment. It is hoped that the algorithm can use the sparse method to make it lightweight. Therefore, learning using key samples and small samples is particularly critical.
The problems faced by small sample learning can be divided into three aspects: modeling, measurement and optimization.
The problem with models is how to build stable models with sparsity, selectivity, and variable updates.
The problem with measurement is how to design measurement methods that are tailored to the actual data set so that the network learns the best parameters.
The problem of optimization is to complete the classification and regression tasks of massive small samples by adjusting the optimization method.
This is the "old comrades" encountering new problems, but also to let the "new comrades" join the big family of artificial intelligence.
In addition, there are some other bottlenecks that need to be solved.
The success of deep learning relies heavily on data sets. There is also data, and the problem is also in the data. Therefore, the search and collection of high-quality data and the formulation of consistent decision-making methods are the fundamental crux of the matter. How to solve the model collapse problem, feature homogeneity problem, imbalance problem, security problem, and local minimum problem are all bottlenecks that plague the development of deep learning.
2
Post-deep learning – cognitive modeling
So in the post-deep learning era, how should we solve the above problems?
Our thinking is cognitive modeling.
Neural networks originate from the calculation of brain nerves, but when we look back at the process of brain nerves in biology, we will find that the real biological brain does not use simple calculations to achieve brain cognition.
All modeling in brain-like structures is sparse, learnable, selective, and directional. Unfortunately, these natural biological properties are not fully taken into account in our current neural network designs.
This is both a pity and an opportunity.
Current deep learning techniques utilize only parallel inputs, outputs, and massive amounts of neurons to solve the problems encountered.
Therefore, a careful review of the structure of the human brain is beneficial to guide researchers to design better neural network structures.
It can be said that the biological basis of brain-like perception and brain cognition provides new ideas for the realization of efficient and accurate complex perception and interpretation.
The idea of neural networks is: sense, know, use.
Macroscopically, the neural network model needs to first model the cognitive characteristics of humans, combined with the macroscopic simulation of deep structure, multi-source synthesis, microscopic simulation of neuronal sparse cognition, direction selection, and mesoscopic simulation information such as significant attention and lateral inhibition between neurons, and design units with characteristics of sparsity, selective attention, and directionality to build a new deep learning model. Improve the ability to characterize, process and extract complex data through the modeling of cognitive characteristics. Realizing this idea is an important task for us.
To sum up, cognitive modeling is the analysis and simulation of the microscopic, mesoscopic, and macroscopic characteristics of the cognitive process of the human brain.
But our work in that area is far from enough.
For example, as early as 1996, corresponding physiological findings and treatises were made on the sparseness of neurons. And published in Nature, Science and other well-known journals. It's been around for two or three decades, but we still haven't fully exploited it. The sparse modeling of cognition is a problem that we urgently need to solve. Technically, sparsity modeling refers to the development of a new paradigm of sparse cognitive learning, calculation and recognition of sparse cognitive learning, such as the efficient scene information sparse learning based on the mechanism of biological retina, the dynamic information processing and sparse calculation of various neurons in the primary visual cortex, and the sparse recognition characteristics of the characteristics of neurons in the middle/advanced visual cortex. We have published these ideas and findings in the Journal of Computer Science in 2015.
In terms of cognitive modeling and the utilization of sparsity, we combine the characterization of sparsity and deep learning, as well as the randomness characteristics of the data, to propose a variety of neural network models. This is not only manifested in the adjustment of parameters in the training process, the improvement of training skills and performance, but also in the study of the internal relationship between deep learning and various traditional machine learning models, in order to understand the working principle of deep learning and build a more powerful and robust theoretical framework.
Model sparsity is not only manifested in the approximation of the activation function, but also in the design of the classifier, but also in the treatment of random characteristics. The results of the study we present include the treatment of structures, but also include sparse regularization, pruning of connecting structures, low rank approximations, and sparse self-coded models. These methods are very effective in actual operation.
In addition, we propose fast and sparse deep learning models, sparse deep combinatorial neural networks, sparse deep stack neural networks, sparse depth discriminant neural networks, and sparse depth differential neural networks. In practice, the effectiveness and advanced nature of these methods have also been verified.
In terms of the combination of brain-like learning and deep learning, we found that humans can learn generalized knowledge from a small amount of data, that is, the ability to learn "abstract knowledge". We can hope to express this property in neural networks.
Let's take a typical example.
In this work, we combine the Wishart distribution characteristics and DBA of polarized SAR data, and at the same time use the characteristics of local spatial information coding of data to establish a rapid polarized SAR classification model, which has a good implementation effect.
At its core is the combination of the mechanisms of physics and the model of deep learning. The article, published in IEEE Transcation on Geosciences and Remote Sensing, received a lot of attention.
Similarly, in order to make the model structure more efficient, we combine the stack and the model to propose a fast deep learning model with high speed, high automation, and good robustness.
It achieves automatic, efficient and accurate classification through automatic high-level semantic feature extraction of target data. The work was published in IEEE Transactions on Image Processing. This work is fast because it incorporates physical properties into deep learning's parallel processing models.
We also used brain-like selectivity for research. Its biomechanics were published in the journal Science science in 2011 and in the journal Neuron Neurology in 2012. Its biological principles suggest that the processing of visual information has a significant attention mechanism. This mechanism of attention is the same as that of the human brain.
Modeling attention mechanisms in the human brain enhances the ability to learn concepts and cognitively. Attention is an important part of human cognitive function. When human beings are faced with massive amounts of information, they can pay attention to some information while selectively ignoring some information. The attention mechanism in the field of computer vision and the signal processing mechanism of the brain are also similar. For example, the Transformer technology of the recent fire is a similar principle.
In terms of brain-like directional binding studies, the victory mechanism we are based on was published in the journal Nature in 2015. It points out the presence of cells in the biological brain that can sense directional and oblique angles of direction and position. In the field of artificial intelligence computer vision, the actual processing of image and video information also has direction and orientation of the variable information, it is the same as the background of the human brain.
In our work considering directionality, we modeled the geometry and designed a multiscale tensor filter with directionality.
This work has shown great use in military products.
In addition, we have used multi-scale geometry theory more than 20 years ago to establish a new generation of decomposable and reconstructable deep learning theory. We can not only construct the differential features of the hierarchy, but also make the differential features of different levels of abstraction form a new signal representation, becoming a new deep decomposition reconstruction model. All of the above work is based on the research process and development inertia of multi-scale geometry and deep learning.
Looking back, in the early 1990s we proposed the theory of multi-wavelet networks, then the wavelet SVM support vector machine, the multi-scale Ridgelet network, and most recently the deep Contournet network.
We combine the directionality, approximation capabilities, and convolutional neural networks of TheCountlet to form a new job to achieve better experimental results. The work was also accepted by IEEE Transcations on Neural Networks And Learning System 20.
The Ridgelet network we propose, also known as the ridge wave network, can itself regularize spots and waves. We combined it with ridge wave networks to achieve excellent results in the classification scenario of SAR images.
We also modeled synaptic structures. Synapses are physiological structures that have a variety of functions, such as memory and storage, which are not currently fully utilized. Our main findings include long-term enhancement and inhibition, both of which were rarely reflected in the prior work.
In order to effectively and efficiently process massive amounts of data, another problem in the post-deep learning era is the automatic learning and processing of data.
The evolution of learning models has evolved over the decades: from shallow neural networks in the 1960s, to the discovery of backpropagation in the '70s, to convolutional networks in the '80s, to the resurgence of unsupervised, supervised deep learning around 1990 and 2000, to the current network model. Looking back at this evolutionary process, we find that more effort should be made to study adaptive deep learning.
In terms of adaptive deep learning, we still have many problems and challenges to solve. These problems are that on the basis of feature engineering, feature learning, perception + decision-making and environmental adaptation, we must enable machines to learn to learn, learn to adapt to the environment, and learn to perceive decision-making. We need not only to enable machines to generate adversaries, architecture searches, and transfer learning, but also to enable models to learn automatically and to explore new structures.
This diagram sorts out some of the international deep learning structures and capabilities. After combing through the context of these works, we can find that these works are solving some basic problems in deep learning. Of course, this is also building some new processing structures, including the effective use of knowledge and knowledge, and the effective combination of edge cloud.
Although we have an overview of the structural development of the model, automatic learning still faces great challenges. In particular, considerable problems were encountered in the automatic determination of network structure hyperparameters. In this regard, many people are caught up in the field of hyper-ginseng engineering. But in my opinion, this work does not have much scientific thinking, and it is all the routine work of some "code farmers and ginseng". It's the lowest level, hard, inefficient, and meaningless work. Neural network architecture search NAS is a new way to liberate manpower. Our question now is how to search for the best structure for the problem that needs to be solved.
For the adaptive neural tree model, we used a combination of neural networks and decision trees to assemble. The work was first proposed by researchers at UCL, Imperial And Microsoft. They propose a prototype adaptive neural tree model ANT that relies on patterns of various data. For complex, live, and varying data, it is a challenge to design an adaptive, fast, differentiated, BP-trainable training algorithm.
Another problem is deterministic reasoning for probabilistic generation. In the process of model learning, many times "inspiration" is needed. Memory and learning are always effective and reversible. This is not only a contradiction, but also a two-sided body of contradiction. So how do we take advantage of this relationship in the process of model learning? Similarly, in the architecture search of the approximation theory of functions, we propose a deep Taylor decomposition network to solve the problem of difficult differentiation. It adopts a layer-by-layer disassembly approach to solve the problem that the deep network is too complex to be derived.
3
Post-deep learning – progressive evolution
Another problem facing the post-deep learning era is "gradual evolution." Why did we come up with this concept? This is because we go from cognitive modeling, automatic learning, to gradual evolution, not only to locate the fragile problems such as noise and nonlinear transformations of scenes and devices, but more importantly, to solve the problems arising from the complexity of facing massive, small samples of data.
The nature of progressive evolution is inspired by the aforementioned artificial intelligence, biological intelligence, and computational intelligence. We hope that the network can carry out full perception and comprehensive cognition, and then carry out the coordinated development of perception and cognition. The basic idea of progressive evolution is dynamic evolutionary optimization, similarity between learning moments, and finally domain-adaptive learning.
That is, to combine the machine learning algorithms that are now based on gradient learning with the natural evolutionary Darwinian evolutionary calculations to construct efficient algorithms. This is the basic meaning of gradual evolution.
The perception and cognition of the human brain is at the heart of evolution and optimization. These include weight optimization, structure optimization, sparse network optimization, and network pruning methods. They all rely on a combination of traditional gradient algorithms and evolutionary computations. Therefore, we must consider the optimization of co-evolution in the combination of network model and learning algorithm. This is one of the important issues we need to consider. We also deployed deep learning algorithms into the time FPGA system and achieved very good results.
Once again, we look back at the origins, foundations and innovations of ARTIFICIAL intelligence. This is a research direction that needs to break through the card neck technology from the source, and we must combine the biological mechanism, the physical and chemical mechanism, the mathematical mechanism, the algorithm design and the hardware environment to achieve a benign closed loop from brain science to cognitive computing and finally to artificial intelligence.
In fact, the development of deep learning and artificial intelligence has also undergone a similar process. The Nobel Prize in brain science, the Turing Prize in artificial intelligence, and the nobel prize in cognitive science are all the foundations for the development of artificial intelligence.
Therefore, the organic combination of brain science, artificial intelligence and cognitive science is an important direction for the next stage of artificial intelligence development.
Artificial intelligence, from brain-like perception to cognition, requires us not only to perceive things, but also to recognize, and to learn to think, make decisions and act. This involves a variety of disciplines, including psychology, philosophy, linguistics, anthropology, artificial intelligence, and neuroscience.
Thank you.
Lei Feng network Lei Feng network