Fish and sheep from Ofei Temple
Qubits | Official account QbitAI
This big model wind in the field of AI can be described as sweeping the world, blowing more and more vigorously.
It is said that in the second half of 2021, Microsoft Nvidia joined hands to launch a 530 billion parameter NLP model, and then saw Ali Damo Academy push the universal pre-training model parameters to 10 trillion in one go.
Just recently, Zuckerberg also announced that he would smash 16,000 pieces of NVIDIA A100 to create the world's fastest supercomputer, which is to train trillions of parameter-level models.
The big model is just right, can the small model have nothing to do?
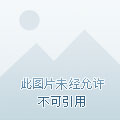
At the "Journal of the Chinese Academy of Engineering: Forum on the Academic Frontiers of Youth in the Field of Information", Alibaba Damo Academy, the Institute for Advanced Study of Zhejiang University in Shanghai, and the Shanghai Artificial Intelligence Laboratory jointly gave a new answer:
Meruzo mustard, mustard seed Nasumi.
The co-evolution of large and small models can make full use of the application potential of large models and build a new generation of artificial intelligence systems.
What do you mean by that?
This brings us to the real dilemma behind the "arms race" of the big model.
Size models co-evolve
The core problem is very simple to summarize, that is, how should the big model land?
The big models with parameter scale of tens of billions, hundreds of billions, and even trillions of dollars, although the language ability and creative ability are in full bloom, but they really want to be deployed into the actual business, but they are facing the problem of energy consumption and performance balance.
To put it bluntly, it is the big model of the competition for the growth of the number of parameters, the scale is too large, it is difficult to really deploy applications on mobile phones, cars and other end-side devices -
You know, with a GPT-3 of 175 billion parameters, the model size has exceeded 700G.
The 2022 Top Ten Technology Trends Report of DAMO Academy also mentioned that after a whole year of parameter competition mode, in the new year, the scale development of large models will enter a cooling off period.
However, in this "pain period", it is not that no one has tried to eat the crab of "big model industrial application".
For example, behind the Alipay search box, the industry's first pre-training model has been piloted.
Of course, it's not about forcing a big model into your phone—
A joint research team from Alibaba Damo Academy, Shanghai Zhejiang University Advanced Research Institute, and Shanghai Artificial Intelligence Laboratory has compressed the M6 model of 340 million parameters to one million parameters through technical means such as distillation compression and parameter sharing, retaining more than 90% of the performance of the large model at the scale of 1/30 of the large model.
Specifically, the compressed M6 minimodel is only 10MB in size, which is nearly 40% smaller than the open source 16M ALBERT-zh minimodel, and the effect is better. What is rare is that the 10MB M6 model still has text generation capabilities.
In terms of mobile sorting model deployment, the research team has also tried.
Mainstream models are compressed, distilled, quantified, or parameter shared, often resulting in a large loss of accuracy for small models.
The team found that after splitting the large model on the cloud, it can form a fine and lightweight submodel of the end side that is less than 10KB, that is, to ensure that the end-side inference accuracy is not lost, and at the same time realizes lightweight application side resources. This is also the end-cloud collaborative reasoning.
In Alibaba's application scenario, based on this collaborative reasoning mechanism, the research team combined with technologies and information such as representation matrix compression, cloud sorting and scoring as features, and real-time sequences, and built a terminal rearrangement model.
The pilot deployment of this technology in Alipay search and Taobao-related applications has achieved significant improvement in reasoning effect, and the relevant 100-mode design has solved the problem of maximizing the user experience of unpopular users without sacrificing the service experience of popular users.
From the above cases, it is not difficult to summarize a feasible way for the implementation of the big model:
Take the essence of the big model, simplify the complex, and transform the big model into a small model available to the terminal through high-precision compression.
The benefit of this is not only to release the ability of the large model to the end side, but also to the end-cloud collaboration of the large and small model, and the small model can also feedback the algorithm and execution effect to the large model, which in turn improves the cognitive reasoning ability of the large model in the cloud.
Damo Academy, Zhejiang University and Shanghai Artificial Intelligence Laboratory further summarized this technical route into the end-cloud collaborative AI paradigm:
As a super brain, the cloud model has a huge amount of prior knowledge and can carry out in-depth "slow thinking".
The small model of the end side, as a limb, can complete efficient "fast thinking" and strong execution.
The two co-evolved to allow AI to move toward cognitive and near-human intelligence.
Based on this kind of thinking and practical experience, the tripartite joint research team recently launched the end-cloud collaboration platform Luoxi.
The platform aims to precipitate the best practices on both sides of the end cloud in the form of documents, algorithm components, and platform services, and provide developers with one-stop end cloud collaborative model training, deployment, and communication capabilities.
Specifically, the Luoxi platform can be disassembled into three parts: end-side, cloud-side, and end-cloud link.
Among them, the end side provides services in the form of Python/js packages, called Luoxi-lite, which contains capabilities such as characterization, text understanding, graph computation, and so on.
On the end cloud link side, the platform provides key communication capabilities for realizing end-cloud collaboration, including solution distribution links and data communication links.
The model training of end-cloud collaboration is precipitated in the cloud, called Luoxi-cloud, including end-model training.
At present, in addition to the M6 model and sorting model deployed in the search scenario mentioned above, the research team has also completed the deployment of graph neural networks, reinforcement learning and other technologies under the end-cloud collaboration paradigm with the help of LuoXi.
It is worth mentioning that on January 12, the core technology of the large model on the cloud in the Luoxi platform, "Super-large-scale High-performance Graph Neural Network Computing Platform and Its Application", won the first prize of the 2021 Science and Technology Progress Award of the Chinese Institute of Electronics.
Mustard Nasumi accelerates the application of large models
Having said all this, a simple summary is that no matter how amazing the effect of the big model is, for the industry, it is the landing application that is true.
Therefore, for the next stage of the development of the big model, the competition will not only be who burns more GPUs and who has a larger scale of model parameters, but also who can fully apply the ability of the big model to specific scenarios.
In this transition period of the big model from spelling "scale" to spelling "landing", the idea of "Mustumi Tibetan Mustard and Mustard Seed Nasumi" proposed by The Dharma Academy, Zhejiang University and Shanghai Artificial Intelligence Laboratory is particularly worthy of attention.
"How does the huge Mount Meru fit into the tinyest seeds?"
For the current speculation of large models and small models, solving such a problem is a step further in making full use of the capabilities of large models and exploring the next generation of artificial intelligence systems.
Combined with the changes in the historical form of computing, with the outbreak of Internet of Things technology, at present, although the cloud computing model has been further strengthened by the blessing of communication technology, local computing needs continue to emerge exponentially, and it is not realistic to hand over all computing and data to centralized cloud computing centers.
That is to say, the development of a computing model that not only exerts the advantages of cloud computing, but also mobilizes the computing agility of the terminal is where the current need lies.
It is also under the trend of such end-cloud collaboration that the collaborative evolution of the size model has a new paradigm to follow: there is a generalized model on the cloud side, a personalized model on the end side, and the two models collaborate, learn, and reason with each other to achieve two-way collaboration between the end cloud.
And this solves the problem of performance and energy consumption balance we mentioned at the beginning of the landing process of the big model.
As Professor Wu Fei, executive vice president of the Shanghai Institute for Advanced Study of Zhejiang University, said, the key from the large model to the small model available to the terminal lies in "taking its essence and simplifying the complex" to achieve high-precision compression; and under the framework of end-cloud collaboration, the practical accumulation of the small model will be "gathering the wisdom of the people fearlessly in the saints" for the large model.
What do you think?
— Ends —