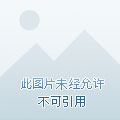
Shin Ji Won reports
Editor: Editorial Office
【New Wisdom Yuan Guide】The model is not open? It's okay, Amazon Web Services helps you open. The official announcement of the Bedrock platform, custom chips to lay down the cost of training inference, generative AI competition, more and more exciting.
The AI race in full swing, Amazon Web Services has also ended.
Somewhat different, in addition to building their own AI models, they also recruit third parties to host models on Amazon Web Services.
With this platform, called Amazon Bedrock, Amazon Web Services officially entered the generative AI track.
Running generative AI applications at scale on a top-performing cloud infrastructure, there's no doubt that Amazon Web Services is revolutionizing.
The "cornerstone" of AIGC: the foundational model
For more than a decade, much of the research in AI has focused on training neural networks to accomplish a specific task.
For example, in CV, image classification, segmentation, or recognition of whether it is a cat in the image; In NLP, semantic retrieval, translation, and so on.
Until the birth of ChatGPT, and even from Stable Diffusion, the ability of generative AI gradually broke people's original cognition.
AI tools with the help of large models can not only create novel content, but also generate pictures, videos, music, and things it has never seen before.
The researchers found that when the number of parameters is sufficient, or reaches a certain scale, a large model will have an unpredictable phenomenon, that is, the ability to "emerge".
Advances in machine learning, especially neural network architectures based on Transformer, have made it possible to develop FMs on a scale of billions of parameters.
It is the rise of super-large models such as GPT-3, DELL-E, GPT-4 and so on, which has made a major change in the training paradigm of language models.
The model training process no longer relies too much on explicit labeling, and can predict words based on existing words in the sentence, realizing the cognitive progress of the agent.
In fact, these large models are the "Foundation Models" proposed by Li Feifei and others in 2021.
Because it is pre-trained on a large amount of data, the base model has become highly adaptable and capable of completing a series of downstream tasks.
The advantage of a base model is that it can be used to fine-tune a specific domain, or to create a starting point for a business model. FM is certainly the best at understanding the behavior of numbers.
To fine-tune the model, you need to further train the model on a specific dataset (with labels).
And this kind of dataset is needed to solve a specific task, because large models can only be "very understanding", but not "very specific".
The fine-tuned model combines two advantages: one is to understand the structure of the data, and the other is to understand the background of the business problem in the form of annotated data.
In this way, the fine-tuned model can also be implemented to generate human-like text or images in specific domains.
For example, Bloomberg launched BloombergGPT, a 50 billion parameter large model for the financial sector.
It can be seen that the basic model is the key to generative AI and the cornerstone of AIGC.
Although the big model is good, the average person can't afford to use it
At present, the highest demand in the entire industry is where big models are available.
We all know that training a super-large-scale language model is also inseparable from the troika: algorithms, computing power, and data.
What is also needed is super algorithms, super computing power and data.
Take training ChatGPT, for example, Microsoft has revealed that it spent billions of dollars for OpenAI and used more than 30,000 NVIDIA A100s to build supercomputing.
In order to get out of this predicament, it is reported that Microsoft invested a team of 300 people as early as 2019 to develop its own chip Athena.
In terms of data, although only a small sample of data is now relied on to train models, data availability is also a big problem.
Recently, the New York Times said that Reddit began planning to charge companies that use platform data to train models, including Microsoft, Google, OpenAI and others.
To train a really good big language model, it not only costs capital, but also takes enough time. In 2022, after the GPT-4 model training was completed, it took another 6 months to fine-tune and test.
This explains why most companies want to use large language models, but don't want to invest too much. I just want to borrow the generalization ability of the basic model and use the data in my own specific domain to fine-tune the model.
In this regard, Amazon Web Services did a lot of user research, and found that the main needs of customers are——
1. Need an easy way to find and access high-performance underlying large models that deliver great results while meeting your goals.
2. Models need to be seamlessly integrated into applications without having to manage huge clusters of infrastructure or incur significant costs.
3. Want to be able to easily acquire a base large model and build differentiated applications using their own data (small or large amounts of data).
Because the data that customers want to use for customization is invaluable IP, they need to maintain complete protection, security, and privacy in the process, and they want to control how their data is shared and used.
Bedrock: The main thing is a model customization + data privacy
To solve these problems, Amazon Web Services believes that generative AI technology should be made universal.
In other words, AIGC is not a small number of startups and well-funded manufacturers, but more companies should benefit from it.
As a result, a basic large-model cloud service called Bedrock came into being.
Bedrock is also Amazon Web Services' largest attempt in the generative AI market, which may be worth nearly $110 billion by 2030, according to Grand View Research.
It is worth mentioning that the most important feature of Bedrock is that it allows developers to easily customize models and build their own generative AI applications.
At training time, Bedrock creates a copy of the base model for the developer and trains this private copy. All data is encrypted and does not leave the virtual private cloud (VPC). In addition, this data will not be used to train the underlying large model.
In addition, developers can fine-tune models for specific tasks by providing annotation examples in Amazon S3, which can produce satisfactory results without large amounts of personal data.
What's more, Bedrock works with other tools and features on the platform, meaning developers don't have to manage any additional infrastructure.
Self-developed "Titan" + third-party SOTA model
Specifically, Bedrock mainly consists of two parts, one is Amazon Web Services' own model Titan, and the other is the basic model from the startups AI21 Labs, Anthropic, and Stability AI.
The basic model includes:
Amazon developed its own Titan
Claude(Anthropic)
Jurassic-2(AI21 Labs )
Stable Diffusion(Stability.AI)
The construction of Titan's basic model is based on Amazon Web Services' more than 20 years of experience in the field of machine learning.
Titan includes two large language models, one is Titan text for generating text, and the other is Titan Embeddings that personalizes web searches.
Titan text is aimed at summarizing, text generation, classification, open-ended question answering, and information extraction.
Text embedding models enable text input (words, phrases, large articles) to be translated into digital representations with semantics (embeddings embed coding).
Users can customize the Titan model with their own data. Moreover, Amazon Web Services is very protective of user data privacy and will not use user data to retrain Titan models.
Moreover, unlike the "illusion" that often occurs in other large models, Titan pays great attention to accuracy when training, in order to ensure that the resulting response must be of high quality.
In addition to Amazon Web Services' Titan model, developers can also take advantage of other base models.
These include the Jurassic-2 family of multilingual large language models developed by AI21 Labs, capable of generating text content based on natural language instructions, and currently supports Spanish, French, German, Portuguese, Italian and Dutch.
There is also Claude, a large language model developed by Anthropic, capable of performing multiple rounds of dialogue and text processing tasks.
The third basic model is Stability AI's text image generation model Stable Diffusion.
With these models, developers can customize their own models with just 20 samples.
For example, a marketing manager wants to develop an ad creative for a new handbag product, and he simply provides Bedrock with the best advertisement and a description of the new product, and Bedrock can automatically generate media tweets, display ads, and product pages.
Again, all data is encrypted, and no customer data is used to train the underlying model.
Currently, partners such as Coda AI, Deloitte, Accenture, Infosys and others have used Bedrock.
The outbreak of AIGC has led to a surge in demand for cloud service providers
As technology evolves, the demand for AIGC content from all walks of life is increasing, from marketing to customer service to news and entertainment.
This is a very good opportunity for those providers who provide infrastructure services.
Gartner predicts that AIGC data will account for 10% of all data by 2025, up from less than 1% today.
In order to take a place in this space, Microsoft and Google have successively launched their own model-based cloud services - the former announced the integration of ChatGPT into Azure OpenAI Service, and the latter also launched enterprise-grade generative AI tools on Google Cloud.
In contrast, Amazon Web Services has a richer model in Bedrock, not only self-developed titans, but also open source models from other startups. And, it is said that more models will join it.
With its flexibility and customization options, as well as its commitment to privacy, Bedrock is better suited to the unique needs of different industries.
Self-developed chip: The cost of training inference has been knocked down
Although the model is now available, the cost of training and inference needs to be further reduced in order to make it available to more people.
Amazon Web Services' self-developed chips undoubtedly bring great advantages to it.
These include Inferentia2 and Trainium, which allow customers to work on machine learning with minimal costs.
Trainium-powered Trn1 compute instances can save up to 50% in training costs compared to other EC2 instances.
And optimized to distribute training tasks across multiple servers connected to a second-generation EFA (Elastic Fabric Adapter) network of up to 800Gbps.
800 Gbps is a lot of bandwidth, but Amazon Web Services also announced new network optimizations for the universal availability of Trn1n instances.
It offers 1600 Gbps of network bandwidth and is designed to deliver 20% better performance than Trn1 for large, network-intensive models.
In addition to the massive computing power required for training, millions of inference requests per hour also cost a lot when the underlying model is deployed at scale.
Amazon Web Services released its first inference-specific chip, Inferentia, in 2018, which has run trillions of inferences and saved hundreds of millions of dollars in costs.
Inf2 instances not only deliver 4x higher throughput and 10x lower latency than the previous generation, but also enable ultra-high-speed connectivity between accelerators to support large-scale distributed inference.
Runway, the company that released the latest text-generated video model Gen-2 some time ago, is expected to use Inf2 to double the throughput of some models.
20 years, AI engraved in DNA
For more than 20 years, artificial intelligence and machine learning have been the focus of Amazon Web Services.
It can be said that invention and innovation in the field of machine learning have been deeply rooted in the DNA of Amazon Web Services.
Currently, many of the features users use on Amazon Web Services are powered by its machine learning, such as an e-commerce recommendation engine, optimizing robotic picking routes, and drone Prime Air.
There's also Alexa, a voice assistant powered by more than 30 different machine learning systems that respond to billions of requests a week from customers to manage their smart homes, shopping, information, and entertainment.
To date, 100,000 customers have innovated using Amazon Web Services' machine learning capabilities.
Amazon Web Services has the deepest to broad portfolio at all three levels of the AI and machine learning stack.
Through continuous investment and continuous innovation, Amazon Web Services provides high-performance, scalable infrastructure for machine learning, and cost-effective machine learning training and inference.
In addition, Amazon SageMaker provides maximum convenience for all developers to build, train, and deploy models;
Amazon Web Services has also launched a number of services that allow users to add AI capabilities to applications such as image recognition, prediction, and intelligent search through a simple API call.
Similarly, in generative AI technology, Amazon Web Services also has to take an important step to empower thousands of industries.
What Amazon Web Services does is make the capabilities of the underlying models accessible to many customers, provide the infrastructure for machine learning inference and training, and make coding more efficient for all developers.
Amazon Web Services has previously worked with companies such as Hugging Face and Stability AI, but has never revealed plans to release homegrown large-language models.
But Swami Sivasubramanian, vice president of database, machine learning and analytics at Amazon Web Services, said the company has long worked on big language models, and LLM has long been used to help shoppers search for products on Amazon Web Services' retail sites, as well as support apps like the Alexa voice assistant.
Several of Amazon Web Services' innovations in generative AI are just the beginning. This is the early stages of a technological revolution that will last for decades.
At this latest node, Amazon Web Services will lower the threshold of AI through its own innovation, thereby empowering thousands of industries.
According to reliable news: the Amazon Web Services Big Model and Generative AI Release In-depth Interpretation Conference will be held on May 25, so stay tuned.