Fish and sheep from Ofei Temple
Qubits | Official account QbitAI
Microsoft's Twin Transformer came out last year, breaking through the problem of Transformer's excessively complex visual tasks.
This practice of "convolutional networking" of Transformer has also become a popular direction in the field of ViT research.
But now, the latest paper by He Kaiming's team makes a different point:
On object detection tasks, complex operations like Swin Transformer may not be necessary.
Only using ordinary ViT as a backbone network can also score high on the target detection task.
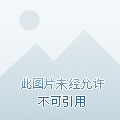
No layered design is introduced into ViT
ViT can be said to open a new door for Transformer to cross-border visual tasks.
But the problem with the original ViT is that it's a non-hierarchical architecture. That is, ViT has only a single-scale feature map.
So in the task of object detection, ViT faces two problems:
First, how to use a pre-trained backbone network to deal with various objects of different sizes in downstream tasks?
Second, the complexity of the global attention mechanism is proportional to the square of the input image size, and the processing efficiency is inefficient when facing high-resolution images.
Represented by Swin Transformer, the solution presented is to learn from CNNs and reintroduce layered designs into the backbone network:
Based on hierarchical feature maps, intensive predictions are made using techniques such as feature pyramid networks (FNs) or U-Net
Limiting self-attention computation to local windows that do not overlap while allowing cross-window connections leads to greater efficiency
The new paper by He Kaiming's team is trying to find a new breakthrough direction.
At its core, it is to abandon the FPN design.
Specifically, the researchers obtained a multiscale feature map by convolving or deconvolving the last layer of feature maps of ViT, thereby reconstructing a simple FPN.
Compared with the standard feature pyramid through the bottom-up, top-down and local connection method of feature fusion, it can be said to be simple and crude.
In addition, when extracting features from high-resolution images, the researchers also employed a window attention mechanism, but did not choose to do shifts like Swin Transformer.
When it comes to information exchange, they divide the block into four sections and explore two strategies: global propagation and convolutional propagation.
As can be seen from the table, the use of 4 conv blocks works best.
This new method is named ViTDet.
The paper also mentions that pre-training combined with the MAE method is more effective.
Judging from the experimental results, the method of using ViT as the backbone network shows better performance than the method of using hierarchical strategies such as Swin and MVITv2 when the model is larger.
The researchers said:
Using ordinary ViT as the backbone network, pre-training based on the MAE method, the resulting ViTDet can compete with all previous advanced methods based on hierarchical backbone networks.
About the author
Yanghao Li, a graduate of Peking University, now works as a research engineer at the Facebook AI Research Institute.
Hanzi Mao, a graduate of Huazhong University of Science and Technology, received his Ph.D. from Texas A&M University in 2020 and is now a senior research scientist at Facebook AI Research Institute.
In addition, in addition to He Kaiming, Ross Girshick also sat on this paper.
Address of thesis:
https://arxiv.org/abs/2203.16527