Reports from the Heart of the Machine
Machine Heart Editorial Department
Are you on the list?
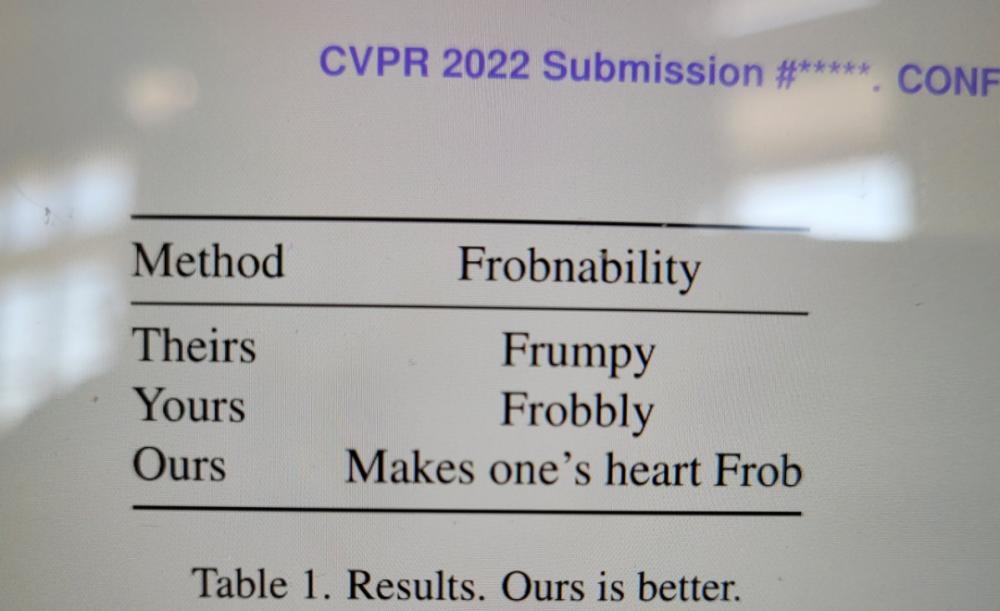
It's on the list!
In just a few minutes, the circle of friends has been swept away by the message of the CVPR 2022 paper reception result announcement.
As the top conference in the field of artificial intelligence, CVPR attracts a large number of research institutions and universities every year, and the number of submissions quickly exceeded 10,000 as it approached the deadline last November – the number of valid submissions for the last CVPR 2021 was "only" 7500. The record number of submissions has put pressure on review, with a total of 6,427 reviewers having 25,801 comments as of January 25, including 3,066 from 2,104 emergency reviewers.
This morning, CVPR officially released the ID of the paper received at this year's conference. According to the Heart of The Machine, 2067 papers were accepted.
List of thesis receiving IDs: https://drive.google.com/file/d/15JFhfPboKdUcIH9LdbCMUFmGq_JhaxhC/view
After the meta review, the final paper acceptance results for this session will be announced in one to two days.
Congratulations to the students on the list!
While cheering and starting to promote themselves, some people also conducted a statistical analysis of the papers received this year for the first time, and it seems that the sooner the paper is submitted, the better:
Obviously, the earlier the students submit, the higher the degree of research completion, not the rush to rush the deadline.
This year's submissions also offer some lessons.
As early as last October, CVPR officially issued an important notice: according to the plan, from October 19, 2021 to March 2, 2022, it will be a social media silence period. During this period, any social media publicity for the paper initiated by the author is considered a violation of the policy.
Today's results are sent down, and there are indeed cases of rejection because of self-promotion submissions. Some authors of the paper said on social software that before the work of the students in his group made a flyer page and uploaded the demo to YouTube, a rejection letter was sent:
The purpose of this regulation is to address the bias problems that arise after the author's institution is exposed, and although some people (such as AI pioneer Yann LeCun) oppose it, believing that it will affect the speed of dissemination of cutting-edge technology, it seems that the CVPR will be implemented very strictly.
However, it should be noted that sending the paper to the preprinted paper platform arXiv, and then using Twitter to forward the link, or others are discussing, is not against the rules.
Biggest suspense: Can Masked Autoencoders win the best paper?
In November 2021, He Kaiming released a paper "Masked Autoencoders Are Scalable Vision Learners" after two years, which was uploaded to arXiv for less than half a day and was on the Zhihu hot list.
At the time, there was some discussion on social networks that the paper might "pre-order" the best CVPR 2022. Partly because MAE research is indeed uploaded in CVPR format, but also because Masked Autoencoders is a very high-quality work. The paper was also uploaded prior to the November 16 CVPR 2022 paper submission deadline.
Thesis link: https://arxiv.org/abs/2111.06377
The paper shows a new approach called masked autoencoders (MAE) that can be used as an extensible self-supervised learner for computer vision. MAE is based on two core ideas: the researchers developed an asymmetric encoder-decoder architecture in which one encoder operates only on the visible subset of patches (without masking tokens), and another simple decoder can reconstruct the original image from potentially characterizing and masking tokens.
The researchers further found that masking most of the input images (e.g., 75 percent) yielded important and meaningful self-supervising tasks. Combining these two designs, we can train large models efficiently: training speeds up to 3x or more, and accuracy.
The authors argue that this scalable approach allows learning from high-volume models that are well generalized: for example, in a method that uses only ImageNet-1K data, the vanilla ViT-Huge model achieves the best accuracy (87.8%). Transmission performance in downstream tasks outperforms supervised pre-training and shows considerable scalability.
Masked Autoencoders proposed a computer vision recognition model with good generalization performance, which is expected to bring new directions to the large model of CV, and it is reasonable to have high hopes of "getting the best paper". But winning the award should not be the most important thing for He Kaiming, after all, he has won CVPR awards many times: including the Best Paper of CVPR in 2009, and the Best Paper in 2016.
After six years, will Kaiming Daishin win the Best Paper Award again? Everything is suspense.