Recently, the team of Professor Liu Haiyan and Associate Professor Chen Quan of the University of Science and Technology of China adopted a data-driven strategy to propose a new protein de novo design route, and the relevant results were published in Nature under the title of "Backbone-centered Neural Network Energy Function for Protein Design".
Proteins are the main performers of life functions, and their structure and function are determined by the amino acid sequence. At present, almost all proteins that can form stable three-dimensional structures are natural proteins, and their amino acid sequences are naturally evolved over a long period of time. When the structure and function of natural proteins do not meet the needs of industrial or medical applications, the structure and sequence of specific functional proteins need to be designed. At present, the internationally reported protein de novo design work mainly uses natural structural fragments as building blocks to splice together to produce artificial structures. However, this method has shortcomings such as single design results and excessive sensitivity to the details of the main chain structure, which limits the diversity and variability of the design backbone structure. The most difficult problem in protein de novo design is how to fully explore the structure space of protein backbones and discover novel, "highly designable" backbone structures, and there is still a lack of related systematic solutions.
The relevant team of the University of Science and Technology of China has been deeply engaged in basic research and applied basic research in the direction of computational structural biology for a long time. The team of Liu Haiyan and Chen Quan has been committed to the development of data-driven protein design methods for more than ten years, and after long-term unremitting efforts, the ABACUS model of the amino acid sequence design of the given main chain structure has been established and experimentally verified, and then the SCUBA model can design a new main chain structure from scratch when the amino acid sequence is to be waited for. SCUBA adopts a new statistical learning strategy, based on kernel density estimation (or neighbor count, NC) and neural network fitting (NN) method, from the original structural data to obtain the analytic energy function in the form of neural network, which can reflect the high-dimensional correlation between different structural variables in the actual protein structure with high fidelity, continuously and extensively search the main chain structure space under the premise of uncertain sequence, and automatically generate a "highly designable" main chain.
Theoretical calculations and experiments have proved that the design of the backbone structure with SCUBA can break through the limitation that only natural fragments can be used to splice to produce a new backbone structure, significantly expand the structural diversity of the de novo design proteins, and then design a novel structure different from the known natural proteins. The "SCUBA Model + ABACUS Model" constitutes a complete tool chain for artificial proteins with a completely new structure and sequence from scratch, and is the only fully experimentally validated protein de novo design method outside of RosettaDesign, and complements it. In the study, the team demonstrated the high-resolution crystal structures of 9 de novo designed protein molecules whose actual structures are consistent with the design model, with 5 proteins having novel topologies that have not yet been observed in native proteins.
According to the Nature reviewers, "Unlike existing methods, existing methods either use parametric equations to describe the space of predefined spiral structures, or methods based on fragment assembly rely on known protein fragments." The SCUBA method in principle allows one to explore arbitrary backbone structures and then fill sequences, allowing one to design a wider range of protein geometries than observed in nature", "Protein de novo design remains challenging, and the high-resolution design of six different proteins in this work is an important achievement that shows that this method works well", "The number of successful designs reported in this study is impressive and provides strong evidence that the underlying technique is robust." The neural network-based energy terms used are novel because they characterize multidimensional features that are unattainable by more traditional statistical methods, which are novel and practical enough."
The research laid the foundation for the design of functional proteins such as industrial enzymes, biomaterials, and biomedical proteins. The research work has been supported by funding from the Ministry of Science and Technology, the National Natural Science Foundation of China and the Chinese Academy of Sciences.
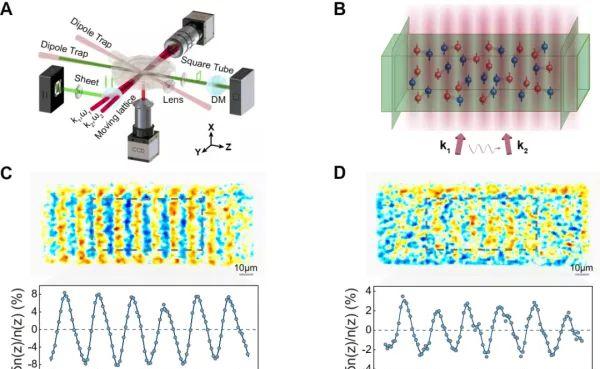
Principles of protein design with SCUBA models. a: The extremely small energy surface of the SCUBA backbone corresponds to the designable backbone structure of the protein, that is, the lowest free energy structure under a specific amino acid sequence; b: the statistical energy term represented by the neural network in SCUBA; c, d: the method framework for learning the analytical energy function from the original data of the protein structure using the near neighbor count (NC)-neural network (NN) method
Comparison of the high-resolution crystal structure (sky blue) of the de novo design protein with the design model (green).
Source: University of Science and Technology of China