Reports from the Heart of the Machine
Editor: Du Wei
When a field gets bigger and bigger, with more papers published each year, is that good or bad thing for the field?
A week ago, the number of citations for He Kaiming's ResNet paper, a classic in the field of computer vision, exceeded 100,000, and this is only six years since he submitted the paper. The popularity of this work is so high, which not only shows the provenness of ResNet itself, but also confirms the popularity of the AI field, especially computer vision.
However, behind The resNet's high citation also gives us a question, that is, the field of computer vision produces so many new papers every year, why do researchers often choose it as a citation? Will the tendency toward highly cited classical papers bring progress or stagnation to the field? Is it possible for a newly published paper to become the next classic?
In a paper recently published in the SCI journal PNAS, "Slowed Canonical Progress in Large Fields of Science," two researchers from Northwestern University and the University of Chicago answered these questions and delved into the intricate correlation between the number, quality, and citations published in the scientific field.
There is a very direct view of scientific progress, that is, the more the merrier. The more papers published in a field, the faster the speed of scientific progress; the greater the number of researchers, the wider the coverage. Even though not every paper has a significant impact, they all become grains of sand that accumulate into sand piles, increasing the likelihood of qualitative change. In the process, the scientific landscape has been reconfigured and new paradigms have emerged in structural inquiry.
The publication of more papers also increases the likelihood that "at least one of them contains significant innovations". A disruptive new idea can shake the status quo, draw attention from previous work, and get plenty of new citations.
The prevailing policies in the field are a good reflection of this view that the more the merrier. Scholars are evaluated and rewarded based on their productivity, and publishing more papers over a period of time is the surest path to tenure and promotion. The number remains a benchmark for universities and companies to compare, with the total amount of published works, patents, scientists and funding remaining a top priority.
Quality is also judged primarily by quantity. Citations are used to measure the importance of individuals, teams, and academic journals in a field. At the dissertation level, it is often assumed that the best and best value papers will attract more attention, thus shaping the research trajectory in the field.
In the paper, they predict that when the number of papers published each year is very large, the rapid flow of new papers will force the academic community to focus on widely cited papers, thereby reducing the focus on less mature papers, even if some of them come up with novel, useful, and potentially transformative ideas. The advent of a large number of newly published papers has not caused a faster change of field paradigm, but has consolidated those highly cited papers, preventing new work from becoming the most cited and widely known field classic.
Researchers have validated these ideas through experimental analysis, showing that the focus on quantity in scientific research units may hinder fundamental progress. This adverse effect will be exacerbated as the number of works published each year in each field continues to grow. And, given the deep-seated, intricate structure that drives awareness of the "number of publications first" field, this situation will be inevitable. Policy measures to reconstruct the value chain of scientific productivity need to be adapted to refocus the public on new ideas with potential.
What is this article about?
This paper focuses on the impact of field size, i.e. the number of papers published in a given field in a given year. Previous research has found that inequality in citations across many disciplines is increasing, at least in part by preference. However, a paper often fails to maintain their citation levels and rankings over the past few years. Disruptive papers can replace previous jobs, and natural fluctuations in the number of citations can also affect paper rankings.
As a result, the researchers predict that when the field is large enough, the momentum for change will change. The most cited papers will be entrenched and will receive disproportionate citations in the future. New papers cannot accumulate citations through preference dependency, and therefore cannot become classics. Few newly published papers have an impact on established academic shackles.
They give two mechanisms that underpin the above predictions. On the one hand, when a field publishes many papers in a short period of time, scholars have to resort to heuristic methods to develop a sustained understanding of the field. Cognitively overloaded reviewers and readers don't think about the new ideas in new papers when they read them, only linking them to existing paradigm papers. There is a high chance that new ideas that do not conform to existing patterns will not be published, read, or cited.
Faced with this dynamic of change, paper authors have had to firmly link their work to well-known papers. These well-known papers act as "badges of knowledge", defining how to understand new work, discouraging them from researching ideas that are too novel and not easily associated with existing classics. As a result, the probability of breakthrough new ideas being generated and published and widely read decreases, and the publication of each new paper will also disproportionately increase the number of citations for highly cited papers.
On the other hand, if new ideas arrive too quickly, competition between them can prevent any new ideas from being widely known and widely accepted within the field. As for why this is the case? The researchers use the sand pile model of disseminating ideas in a certain field as an example.
When the sand slowly falls on the sand pile, one grain at a time, and then one grain at a time when the sand pile movement stops. Over time, the sand pile reaches a scale-free critical state, and one grain of sand can cause the entire sand pile area to collapse. But when the sand falls at a very fast rate, adjacent small collapses interfere with each other, causing no grain of sand to trigger displacement within the sand pile. This means that the faster the sand falls, the smaller the area that each new grain of sand can affect. The same is true of papers, if the paper appears too quickly, no new paper can become a classic through local diffusion and preference attachment.
These two arguments have spawned six predictions, two of which are the long-term dominance of the highest-cited papers and the futility of newly published papers and their own subversive decline.
In short, compared to a field that publishes very few papers per year, when the field produces a lot of papers per year, it will face the following six situations:
New papers will be more likely to cite the highest cited paper than the low-cited one;
The list of highest-cited papers each year hardly changes, resulting in classic papers always being those;
The probability of a new paper becoming a classic will decline;
New papers entering the list of highly cited papers are not achieved through a cumulative dissemination method;
The proportion of newly published papers developing existing scientific ideas increased, while the proportion of subverting existing ideas decreased;
A new paper is less likely to become disruptive work.
What data and methods are used?
Using the Web of Science dataset, the researchers analyzed papers published between 1960 and 2014, totaling 90,637,277 papers and 1,821,810,360 citations. The Web of Science divides academic fields, or in some cases large subfields, into different disciplines. As a result, there are a total of 241 disciplines in the investigator's classification and use them as the basis for domain-level analysis. Among them, the number of citations received by a focus paper from newly published papers on the same topic each year constitutes the main interest variable of the researcher.
To calculate the 1-decay rate (λ) of the 10 largest non-multidisciplinary disciplines, for each discipline, the researchers divided the years by 10 log of the number of papers published, with cut-off points of 1, 1.5, 2, 2.5, 3, 3.5, 4, 4.5, 5, and 5.5, and divided the paper years by the most cited percentiles in field-year, with cut-off points of 1, 2, 3, ..., 100, respectively. For each (number of records published) × (citations in hundredths), they returned the number of citations for a paper in the second year to the number of citations in the focus year. The coefficient of this regression yields 1-λ.
In addition, to calculate 1-λ for all disciplines (shown in Figure 2D below), the researchers selected the top 100 most cited papers in the 1st, 2nd, 5th, 10th, and 25th percentiles. They classified subject-years by base 10 log of the number of papers published (cut-off points 1, 1.5, 2, 2.5, 3, 3.5, 4, 4.5, 5, and 5.5, respectively). For each bin× selected percentile, the researchers returned the number of citations for a paper in the second year to the number of citations in the focus year. The coefficient of this regression yields 1–λ.
Have these predictions been confirmed?
All of the researchers' predictions were confirmed in the citation patterns of the Web of Science dataset, as shown in Figures 1 through 4 below. As the field becomes more and more numerous, the most cited papers always become dominant, occupying an absolute advantage in the distribution of citations. In contrast, new papers are less likely to become high citations and cannot gradually accumulate attention over time. Publishing papers often develops existing ideas without being subversive, and rarely produces new research trends that are groundbreaking.
Specifically, the most cited papers received a disproportionately higher share of citations in larger fields. The Gini coefficient for the largest domain reference share is about 0.5, as shown in Figure 1A below. The disproportionate number of citations of high-cited papers has led to increased attention to inequality.
For example, when around 10,000 papers are published annually in the field of electrical and electronic engineering, the top 0.1% and top 1% of highly cited papers account for 1.5% and 8.6% of the total citations. When the field publishes 50,000 papers per year, the top 0.1% and top 1% highly cited papers account for 3.5% and 11.9% of the total citations. When the field is larger, publishing 100,000 papers per year, the top 0.1% and top 1% highly cited papers account for 5.7% and 16.7% of the total citations.
In contrast, the share of the bottom 50 percent of least cited papers decreased in total citations, accounting for 43.7 percent of 10,000 papers published each year, and only slightly more than 20 percent when 50,000 and 100,000 papers were published each year.
When we look at domain data over time, we see a pattern in which the ranking correlation between the most cited top-50 papers increases when a large number of papers are published each year (Figure. 1B)。 In subsequent years, the Top-50 list, which was most cited in a field, increased from 0.25 at 1,000 papers to 0.74 at 100,000 papers.
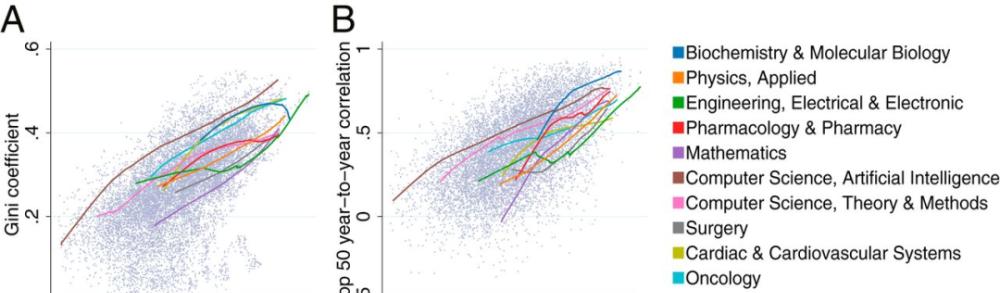
Figure 1
When the field is widespread, the number of citations of the most cited papers continues to increase year by year, while the number of citations of all other papers decreases. Figure 2 below shows the predicted ratio of the number of citations in the current year to the previous year. In years with few publications, the rate of the most cited papers is significantly lower than 1, not much different from that of the less cited papers. However, in years with a large number of publications, the rate of the most cited papers is close to 1, which is significantly higher than that of the papers with fewer citations.
In very large fields, about 100,000 papers were published in the middle of the year, and on average, the number of citations of the most cited papers did not decrease year by year. In contrast, papers ranked outside the top 1% lose an average of about 17% of citations per year, while papers in the top 5% and below tend to lose 25% of citations per year.
Figure 2
When many papers are published in the same field at the same time, the probability of citations reaching top 0.1% of a single paper decreases, a phenomenon that applies in different fields in the same year or in the same field in different years, as shown in Figure 3A. In general, papers in larger fields are cited the most, and are rarely done through processes such as local diffusion.
Figure 3B shows the average time (in years) for an article to enter a related field, provided that the paper becomes one of the most cited papers in the field. When a field is small, papers slowly rise to the top 0.1% of the most cited topics over time. If we publish 1000 papers in a small field (regression prediction) in 1980, it takes an average of 9 years to become the most cited paper if 1000 papers are published in the same field. In contrast, classic papers in the largest fields quickly top citation lists, which is inconsistent with the cumulative process by which scholars discover new works by reading references cited in other people's works. The same regression predicts that in a large field of 100,000 papers published each year, the time for papers to reach the top 0.1% of citations averages less than a year.
Figure 3
Most papers published in the same year build on existing literature rather than disrupting existing literature (Figure 4A). Logical fit predictions show that when 1,000 papers are published in the field a year, 49% of papers have a disruption measure D > 0 (conversely, when 51% D0, the interrupt metric for newly published papers weakens in the larger field). Figure 4B shows the proportion of new papers by domain year, ranked in the top-5 percentile of the interrupt measure. Lowess estimates show that the proportion of new papers with a top-5 percentile interrupt metric has decreased from 8.8 percent at 1,000 papers published annually in the field to 3.6 percent at 10,000 papers per year and 0.6 percent at 100,000 papers.
Figure 4
Quickly build an enterprise-grade ASR speech recognition assistant with NVIDIA Riva
NVIDIA Riva is an SDK that uses GPU acceleration to rapidly deploy high-performance conversational AI services for rapid development of speech AI applications. Riva is designed to help developers easily and quickly access sessionAL AI capabilities, out of the box, and quickly build high-level speech recognition services with a few simple commands and API operations. The service can process hundreds to thousands of audio streams as input and return text with minimal latency.
On December 29, 19:30-21:00, the main introduction of this online sharing is:
Introduction to Automatic Speech Recognition
Introduction and features of NVIDIA Riva
Rapid deployment of NVIDIA Riva
Launch the NVIDIA Riva client to quickly implement speech-to-text transcription
Use Python to quickly build an NVIDIA Riva-based automatic speech recognition service application