· PaLM-E is the largest VLM (Visual Language Model) known to date. As a multimodal embodied VLM, it can not only understand images, but also understand, generate language, and execute various complex robot instructions without retraining. It also demonstrates strong emergence (models behave unpredictably).
By integrating PaLM-E into the control loop, it is resistant to interruptions that can occur during missions. In one video example, researchers grab potato chips from a robot and move them, but the robot finds the chips and grabs them again.
"The advent of AGI (General Artificial Intelligence) is not too far away, but there will certainly be many miscalculations in the process. AI is expected to outperform humans in the next five years' jobs that most humans currently do. A month before the launch of ChatGPT, John Schulman, co-founder of OpenAI and lead of the ChatGPT project, said on the reinforcement learning podcast TalkRL.
AGI does not seem to be far away, but researchers are still exploring how to lead to AGI. Just recently, a new research result was released - using visual data to enhance language processing capabilities. Its performance is pleasantly surprising, demonstrating a strong emergence (the model has unpredictable behavior).
On March 7, Beijing time, the team of Google and the Technical University of Berlin launched the largest visual language model in history, PaLM-E, with 562 billion parameters (GPT-3 has 175 billion parameters).
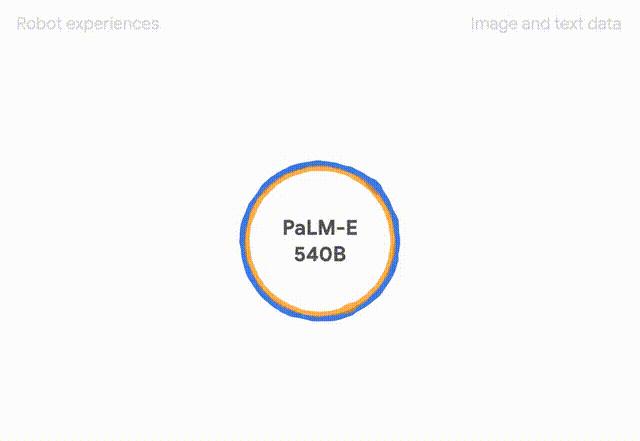
Application of PaLM-E.
"PaLM-E is the largest VLM (Visual Language Model) known to date. We observe emerging capabilities such as multimodal thought chain inference (which allows the model to analyze a range of inputs including verbal and visual information) and multi-image inference (which uses multiple images as inputs to make inferences or predictions) that accept only single-image prompt training. Danny Driess, the paper's first author and a Google AI researcher, said.
A tweet from the paper's first author and Google AI researcher Danny Driess.
In this sense, deep learning models have become more complex over time, and PaLM-E seems to continue the trend of "surprise".
PaLM-E (Pathways Language Model with Embodied) is a combination of the PaLM-540B language model and the ViT-22B visual transformer model. It's called "PaLM-E" because it's based on Google's existing "PaLM" big language model (similar to the technology behind ChatGPT). Google added sensory information and robotic controls to make PaLM "embodiment" (a state closely connected to the body). Since it is based on a language model, PaLM-E takes continuous observations, such as image information or sensor data, and encodes them into a series of vectors of the same size as the language tag. This allows the model to "understand" sensory information in the same way as it does with language. PaLM-E also draws on Google's previous work on the ViT-22B Vision Transformer model, which has been trained on a variety of vision tasks, such as image classification, object detection, semantic segmentation, and image captioning.
Google isn't the only research group working on using neural networks for robot control. This particular work is similar to Microsoft's recent "ChatGPT for Robotics" paper, which attempts to combine visual data and large language models for robot control in a similar way.
As a multimodal embodied visual language model (VLM), PaLM-E can not only understand images, but also understand and generate language, and can execute various complex robot instructions without retraining.
The robot was asked to go to the drawer to get potato chips.
According to Google, when given a high-level command, such as "Bring me the chips in the drawer," PaLM-E can generate an action plan for a mobile robot platform with arms (developed by Google Robotics) and execute its own actions.
PaLM-E achieves this by analyzing data from the robot's camera without preprocessing the scene. This eliminates the need for humans to preprocess or annotate data and allows for more autonomous robot control. It is also resilient and reacts to the environment. For example, the PaLM-E model can guide a robot to pick bags of chips from the kitchen, and, by integrating the PaLM-E into the control loop, it can resist interruptions that can occur during a mission. In one video example, researchers grab potato chips from a robot and move them, but the robot finds the chips and grabs them again.
In another example, the same PaLM-E model autonomously controls the robot through tasks with complex sequences that previously required human guidance. Google's research paper explains how PaLM-E translates instructions into action:
We demonstrate the performance of PaLM-E on challenging and diverse mobile operational tasks. The robot needs to plan a series of navigation and manipulation actions based on human instructions. For example, given the command "I spilled the drink, can you get me something to clean up", the robot needs to plan a message that contains "1. Find the sponge, 2. Pick up the sponge, 3. 4. Put down the sponge" sequence to the user. Inspired by these tasks, we developed 3 use cases to test PaLM-E's concrete reasoning capabilities: availability prediction, failure detection, and long-term planning.
PaLM-E recognizes the basketball star Kobe Bryant in the image and can generate textual information about him, such as how many championships he won.
The researchers write that PaLM-E is also an "effective visual language model." For example, it can recognize basketball star Kobe Bryant in an image and can generate textual information about him, such as how many times he has won championships. In another example, PaLM-E sees a traffic sign and explains the rules associated with it.
PaLM-E sees a traffic sign and explains the rules associated with it.
In addition to robotics, Google researchers have observed some interesting effects that apparently come from the core of PaLM-E – large language models. PaLM-E exhibits "positive migration" capabilities, i.e. it can transfer knowledge and skills learned from one task to another, resulting in "significantly higher performance" compared to single-task robot models.
The larger the language model, the more it can maintain its language capabilities when training on visual language and robot tasks.
In addition, they observed a trend in model scale: "The larger the language model, the more it retains its language capabilities when training on visual language and robotic tasks – quantitatively, the 562B PaLM-E model retains almost all of its language capabilities."
Google researchers plan to explore more applications of PaLM-E in real-world scenarios, such as home automation or industrial robots. They hope that PaLM-E will inspire more research into multimodal reasoning and embodied AI.
"Multimodality" has become a buzzword, and we are likely to hear it more and more. That's because many companies are developing general-purpose artificial intelligence that looks like a human and can perform ordinary tasks.