01
Basic definition of autonomous driving simulation testing
Definition: Without the need for real vehicles, the application scenarios of automatic driving are digitally restored directly through simulation software, and as many real-world system models as possible are established for test verification.
Benefits of Virtual Simulation Testing:
1) Indispensable technical means for automobile research and development, manufacturing, verification and testing, etc., which can effectively shorten the technology and product development cycle and reduce the cost of research and development;
2) It is a key part of the realization of high-end automatic driving landing applications, and vehicles with automatic driving functions must undergo a large number of virtual simulation tests and real vehicle road tests before they can be commercialized.
The three stages of autonomous driving commercialization include: virtual simulation testing - closed field testing - open road testing, and the process of automatic driving development of car companies is also V-shaped, from product design verification, MIL-SIL-HIL-VIL to development verification, test verification, and experience evaluation.
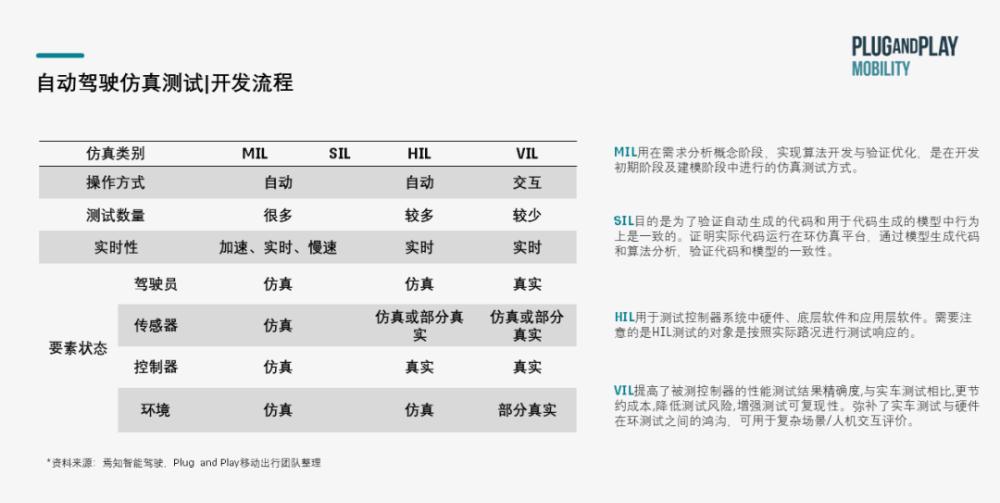
Autonomous driving simulation tests can be divided into five simulation levels according to the state of the four elements of the environment, sensors, controllers, and vehicles, and the higher the level, the more real elements are included.
1) The simulation test of Level 1 is basically based on the verification of the algorithm based entirely on the computer.
2) Level 2 adds sensor signal equipment, and the scene simulation software and vehicle dynamics software are run in the real-time simulator to ensure the real-time operation.
3) Level 3 Compared to Level2, some sensors use real parts.
4) Level 4 scene simulation and vehicle dynamics simulation software joint simulation, with the real vehicle feedback wheel speed, wheel angle, etc. as input, output driving resistance and steering resistance, through the hub test bench feedback to the real vehicle, to achieve closed-loop test. The difficulty lies in the authenticity of the driving resistance and steering resistance simulations.
5) The simulation test of Level 5 no longer places the real vehicle on the drum test bench, but uses the real vehicle to drive on the real road, so it is generally referred to as the vehicle in the ring test VIL. OEMs can choose different levels of simulation tests from level1-5 according to different needs to evaluate the safety and accuracy of the system.
The automatic driving simulation algorithm in ordinary scenarios has been relatively perfect, and the breakthrough difficulty lies in some extreme scenarios (corner cases). Since extreme scenarios can be found in reality and can be easily generated by using simulation platforms, the industry consensus is to increase the proportion of simulation tests in autonomous driving tests. Moreover, the test scenario configuration is flexible, which can improve the test efficiency and reduce the test cost.
Simulation testing, closed field testing, and open road testing complement each other to form a closed loop of testing and jointly promote the research and development of autonomous vehicles and the establishment of a standard system.
1) The simulation test results can be tested and verified in closed sites and open roads;
2) The dangerous scenes obtained through road testing will be fed back into the simulation test, and the setting scene and parameter space can be adjusted in a targeted manner;
3) The final results of simulation tests and closed field tests should be comprehensively evaluated, and the evaluation criteria and test scenario library should be continuously improved based on the evaluation results.
02
Application composition of autonomous driving simulation
The first type of application composition - scene library
The application composition is divided into 3 categories, the scene library as the foundation, the construction of the scene library requires the simulation platform and the evaluation system as the guide; the simulation platform is the core, the development and evolution need the scene library and the evaluation system as the support; the evaluation system is the key, and the establishment and improvement also need to take the existing scene library and simulation platform as the reference basis. The three are closely coupled to each other.
The data sources of the test scene mainly include three parts: real data, simulation data, and simulation data synthesized based on real scene data; in addition, there are four typical test scenarios in the scene library: natural driving scenarios, dangerous working conditions scenarios, standard regulation scenarios, and parameter reorganization scenarios. Almost all scene situations are included.
The scene is the overall dynamic description of the autonomous vehicle and the constituent elements of its driving environment over a period of time, which is infinitely rich, extremely complex, unpredictable, and inexhaustible. The construction of the test scene library can effectively drive the R&D and testing of automatic driving, and the R&D and testing of automatic driving can in turn provide feedback from the scene library and enrich the scene library, thus forming a closed loop.
The standard system of the scene library is based on the C-ASAM jointly established by AsAM, China Automobile Data and German ASAM, and ISO as the reference standards for the industry. ASAM is the most authoritative, about 300 OEMs, suppliers and scientific research institutions around the world are members of the association, and have established a recognized OpenX standard system in the field of simulation testing. The current situation of China's scene library is due to the fact that the database data format standards are not uniform, the unified scenarios are difficult to form, and the standard formulation and certification system lag behind the market progress, so the current database construction of the scene library is in a state of separate battles.
The second type of application composition - simulation platform
Simulation platforms typically include simulation frameworks, physics engines, and graphics engines. The simulation framework is the core of the platform software platform, supporting sensor simulation, vehicle dynamics simulation, communication simulation, traffic environment simulation, etc.
An autonomous driving simulation platform covering all of these modules, mainly validating the stability and performance of the architecture:
Through the simulation environment, combined with the calculation results of some hardware systems for testing, the compatibility and functional integrity of software and hardware can be verified, the functional safety of subsystem modules can be verified by low-cost measurements, and the all-round test verification of local subsystems can be convenient to achieve the purpose of system identification and error tracking and positioning.
The real platform provides algorithm companies with a pure software interface solution for rapid iteration of algorithms, and also provides a system solution that combines software and hardware.
The third type of application composition - evaluation system
Evaluation system for simulation testing. Two important evaluation dimensions: authenticity and validity. Authenticity evaluation: Mainly for the evaluation of the authenticity of the scene library, it is divided into two aspects: the authenticity of scene information and the authenticity of scene distribution. In the second dimension, there is no unified effectiveness evaluation standard, and there are only two kinds of standards that can be referred to at home and abroad, both of which have their own flaws. China's "Autonomous Vehicle Road Test Capability Platform Content and Methods" only covers the daily driving scenarios of cars, and the UNITED Nations NATM Master Document can only be verified in key parts of the scenarios.
03
Market and industrial chain distribution
Market capacity
Autonomous driving simulation testing has been widely accepted by the industry, due to the small number of autonomous driving test vehicles, the automatic driving simulation test platform can make up for the shortcomings of traditional field road testing, making the automatic driving simulation platform a just need to achieve high-level automatic driving. In 2025, the scale of China's autonomous driving market will exceed 800 billion yuan, and the participants of the automatic driving simulation platform include: technology companies, vehicle companies, autonomous driving solution providers, simulation software companies, universities and scientific research institutions. The autonomous driving simulation market is expected to reach at least $20 billion by 2025.
Autonomous driving industry chain map
Internet Simulation Platform:
In terms of simulation, it started relatively late and had less experience in exploring automotive functions, but it has the advantages of big data and strong software development capabilities. Autonomous vehicles have a greater demand for software than traditional cars, and technology companies explore simulation software with the aim of entering the automotive industry with a huge market, establishing a larger data platform, and forming a new business growth point.
Autonomous Driving Solution Providers:
Mainly for their own needs to develop customized simulation software, less external simulation services, but with the help of sufficient funds, rich road measurement data support, their own research and development driving force, in the automatic driving simulation has a strong competitiveness.
Traditional Company & Startup Simulation Platform:
Due to the relatively deep accumulation of technology, traditional simulation software companies have inherent advantages in entering automatic driving simulation, and there are many partners and secondary development has advantages.
Due to the late start-up, the accumulation of technology is weak, and the gap between domestic enterprises and foreign enterprises is large, but relying on strong funds and talent gathering, it is expected to rise rapidly. Among the world's mainstream autonomous driving simulation software companies, the United States and Germany account for more than half of the total number of global companies. Germany and the United States have a solid foundation in the field of traditional simulation software to promote the development of autonomous driving simulation in the world leading position. At present, there are only 3 Startups in China, which has shortcomings in the field of traditional automobile simulation, weak accumulation in automatic driving simulation, and insufficient innovation.
04
Comparative analysis of competition between domestic and foreign benchmarking enterprises
Comparison of innovation points and competition points of key projects at home and abroad
The technical gap in the simulation module of various startups around the world is not particularly obvious, and the main gap lies in the construction of scene library data and the accumulation of simulation test software application and evaluation test system.
Comparison of autonomous driving simulation software platforms of Internet technology companies
05
Pain points and solutions
Confidence problems and solutions
At present, there are three main problems in terms of confidence:
Simulation software itself:
a. Most of the simulation models are based on ideal conditions, and the confidence of the results simulated by the simulation simulator has not yet been evaluated by specific quantifiable indicators;
b. If the noise problem of the radar caused by the sensor hardware or software itself is taken into account, as well as the environmental factors such as rain, snow, water stains, and dust that interfere with the performance of the radar, the radar performance is weakened and cannot be used, or the error of the actual echo signal is difficult to simulate. These problems or phenomena are more difficult problems in lidar simulation.
Simulation platform reproduction and generalization of virtual scenarios:
a. At present, the usual means are to reproduce and generalize more virtual simulation test scenarios through simulation simulators based on real data;
b. There are two main problems in the generalization of real scenes:
1) The direction of generalization is uncertain whether it meets the statistical significance and test requirements;
2) Loss of authenticity of complex dense traffic flow during generalization.
c. Therefore, there must be a difference between the virtual simulation environment and the real environment that reproduces and generalizes, how much of an impact will this difference have on the test results, and what is the level of fit between the test scene and the real scene? Is it within acceptable limits? There is currently no quantifiable evaluation of indicators.
Test Result Evaluation Criteria:
a. At present, the traditional test methods are still based on hardware testing (including HIL hardware in the ring, VIL vehicle in the ring) or real road testing;
b. The parameters cannot be determined whether they are calibrated well, and the real results are difficult to predict, so the test-simulation-test circuit is not open;
c. The data actually verified are not particularly reliable and therefore their authenticity is not guaranteed.
solution:
1) Tencent's automatic driving simulation platform TAD-Sim adopts the method of game rendering + real data dual engine drive, trains traffic flow AI models by using a large amount of real road acquisition data, and then combines game rendering engine technology to automatically build a highly interactive and real-world test scene.
2) Baidu uses the augmented reality automatic driving simulation system - AADS, by scanning the street view by using the lidar and high-definition camera mounted on the vehicle, to obtain static scene images around the vehicle and dynamic trajectory data of traffic movement; using these materials, the system then applies augmented reality technology to directly and automatically create high-fidelity simulation images, making the virtual scenes created closer to the real scene.
3) 51 WORLD uses digital twin test technology to increase the confidence of simulation test results, the real car in the real scene test process, will be in the vehicle in the loop mode of real-time vehicle status data mapping to the virtual scene, while the virtual scene test data and evaluation results will also be fed back to the real world, as a guide and optimization of the real vehicle in the real world for decision-making an important basis.
Scene coverage issues
Corner Case is difficult to cover: For autonomous driving simulation testing, the biggest challenge is to collect all The Corner Cases to cover different road environments, weather environments and traffic conditions.
Corner Case has different regional characteristics:
1) The regional characteristics of "Corner Case" are mainly reflected in the fact that the test scenarios are quite different in different countries and regions. Because the road environment, traffic habits, traffic rules and driving habits of various countries may vary greatly;
2) Regional differences: road environment, traffic conditions, traffic rules, driving habits, etc.
Through the evaluation and assessment of the test scene in the region, it is ensured that it can cope with all the extreme working conditions in the region. Because local simulation companies have the first-mover advantage of "near-water buildings", it is easier to design and develop simulation test software suitable for the domestic road environment, traffic regulations, driving habits and other scenarios.
06
summary
Participants in the autonomous driving simulation platform include technology companies, vehicle companies, autonomous driving solution providers, simulation software companies, universities and scientific research institutions. With the development of the industry, the technical barriers will become more and more obvious. In order to make up for their shortcomings in the field of automotive autonomous driving simulation, traditional simulation giants and Internet technology companies are also constantly acquiring related start-ups to expand their business areas and build their own closed-loop simulation platforms;
For start-ups, it is more important to choose a suitable subdivision field in the simulation field according to their own technical advantages to deepen their cultivation and do an indispensable "software" technology under the "big platform"; and machine learning, as an effective tool recognized as an effective tool to solve the problem of automatic driving long tail, continuously polishing and improving the technical model is the long-term solution to the development of the automatic driving simulation track.