Algorithms have also been exposed to discriminating against black people. Recently, after watching a video with a black protagonist on Facebook, some users were asked whether they were "willing to continue watching primate videos." In response, Facebook apologized for saying it was an "unacceptable mistake" and is investigating its algorithmic capabilities.
Previously, Twitter and Google have been found to discriminate against black people. It is reported that algorithm bias is related to the technical link of machine learning, one of the core technologies of AI. For example, if a dataset used by machine learning carries biases from real society, algorithms will learn those biases. In other words, if AI discriminates against black people and women, a large part of the reason is that there is a phenomenon of discrimination against black people and women in real life.
1 This is not the first time blacks have encountered algorithmic bias
Recently, after watching a video of a black man arguing with white civilians and police on Facebook, a user received a Facebook inquiry "whether they would like to continue watching primate videos." It is reported that the video was released by the British "Daily Mail" on June 27 last year, which did not contain content related to primates.
Facebook apologized on Friday for saying it was an "unacceptable mistake" and said it was investigating its algorithmic recommendation feature to prevent it from happening again, the New York Times reported. Facebook spokesperson Dani Lever said in a statement: "Although we have made improvements to ARTIFICIAL intelligence, we know it is not perfect and there are still many areas that need to be improved. We apologize to anyone who might have seen these offensive referral messages. ”
Darci Groves, a former Facebook employee, tweeted a screenshot of the recommendation tip. Some netizens left messages expressing anger at discrimination, and some people questioned whether there were both blacks and whites in the video, "it may also be that white people are identified as 'primates'." ”
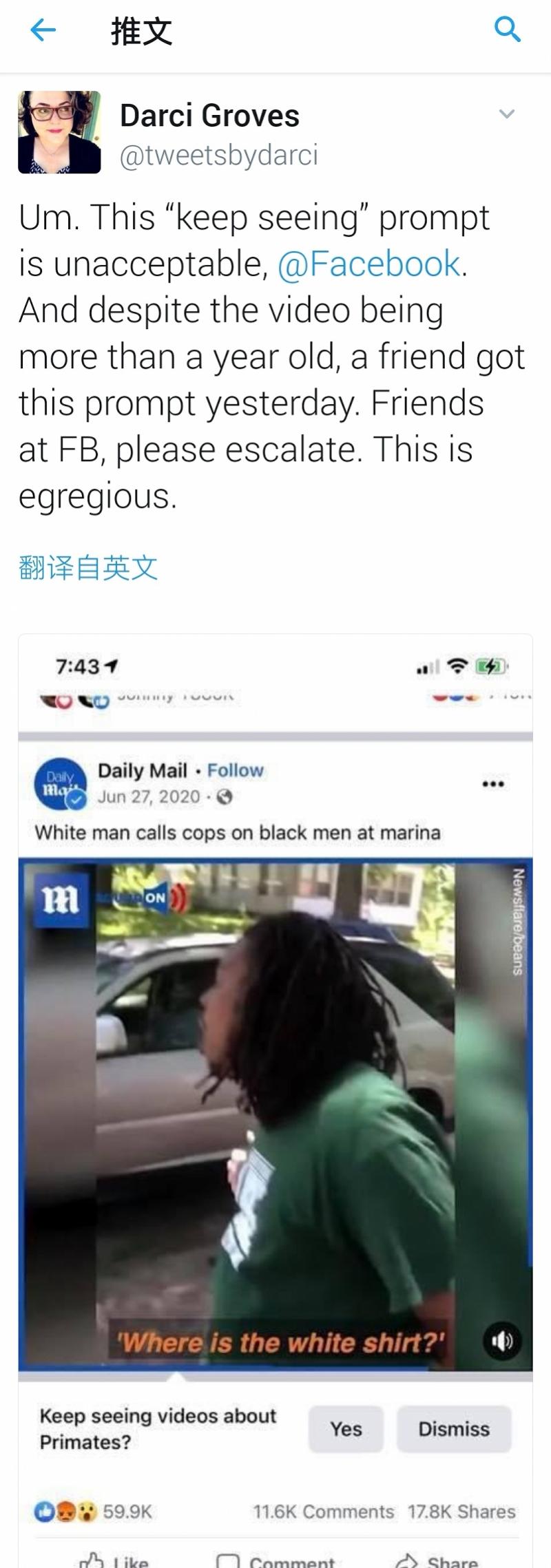
Former Facebook employee Darci Groves tweeted.
This is not the first time blacks have encountered algorithmic bias, though. In May, Twitter's research team published a paper that experimentally confirmed that Twitter's thumbnail algorithm is more white and female when cropping pictures, and more black people when cropping photos of multiple people. Subsequently, Twitter eliminated the ability to automatically crop photos on mobile apps and launched an algorithmic bias hacking contest to look for possible biases in the code.
In 2015, Google Photos also labeled photos of two black people as "gorillas." To fix the bug, Google removed the tag for the term directly from search results, which also led to no images being labeled as gorillas, chimpanzees, or monkeys.
A paper published by OpenAI in February quantified the possible algorithmic biases of AI systems with data. They found that some AI systems had the highest probability of identifying blacks as non-human categories, at 14.4 percent, nearly twice as likely as Indians in second place.
The paper's data shows that some AI systems have a 24.9 percent chance of identifying whites as crime-related categories and a 14.4 percent chance of identifying blacks as non-human.
2 Algorithms learn pre-existing biases in real-world society
In general, developers of AI systems do not deliberately inject bias into algorithms. So, where does algorithmic bias come from?
Tencent Research Institute published an article in 2019 analyzing this problem. They believe that this is related to the core technology of artificial intelligence, machine learning. There are three main links to inject bias into the algorithm: data set construction, goal setting and feature selection (engineer), and data annotation (labeler).
In the process of data set construction, on the one hand, some minority data is more difficult to obtain, the amount of data is smaller, so AI gets less training, so that minorities are further marginalized in the algorithm. On the other hand, the dataset comes from the real world, and there are biases that exist in the real society, and the algorithm will also learn these biases. In other words, if AI discriminates against black people and women, a large part of the reason is that there is a phenomenon of discrimination against black people and women in real life.
In addition, developers may also have personal biases when setting goals or choosing labels. When data labelers mark data, they not only face the problem of "cat or dog" that is easy to judge, but also face the situation of value judgment such as "beauty or ugliness". This can also be a big source of algorithmic bias.
Algorithmic bias is unknowable and untraceable, which poses a challenge for developers. Twitter said in a statement released this year that "it is difficult to find biases in machine learning models." Many times, when unexpected moral hazards are discovered, technology has reached the public. ”
However, the dangers of algorithmic bias are emerging. In April, the U.S. Federal Trade Commission warned that ai-intelligence tools with racial and gender biases could violate consumer protection laws if they were used for credit, housing, or employment decisions.
On August 27, the Cyberspace Administration of China (CAC) issued the Provisions on the Administration of Recommendation of Internet Information Service Algorithms (Draft for Solicitation of Comments) to explore the bias of regulatory algorithms. It is mentioned that algorithm recommendation service providers providing algorithm recommendation services shall comply with laws and regulations and respect social morality and ethics; they shall strengthen the management of user models and user tags, and must not set discriminatory or biased user tags.
Written by: Nandu reporter Ma Jialu