Columbia Chinese developed a "face robot" that looks in the mirror and autonomously imitates human expressions with ultra-realistic
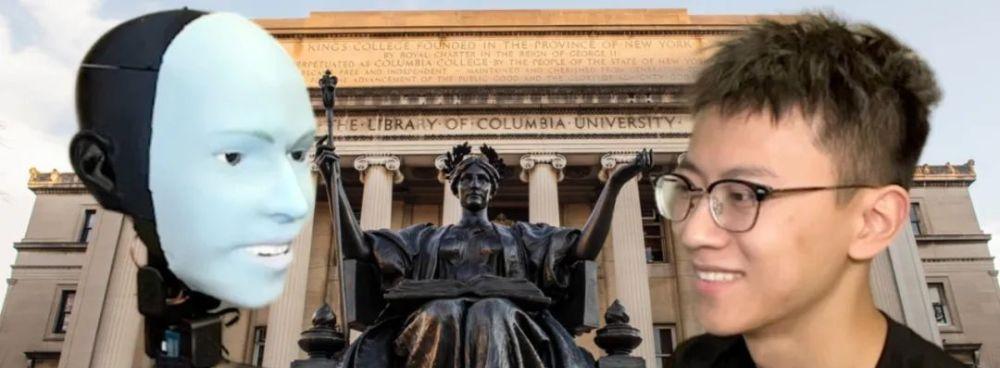
New Zhiyuan reports
Editor: Momoko Run
Although OpenAI robots have strong comprehension, they are unable to communicate non-verbally. Recently, a Chinese team at Columbia University has built a new robot, Emo, that can not only predict and simulate human expressions in advance, but also make eye contact.
Previously, the humanoid robot Ameca's expression of "waking up from a big dream" has made many people feel real "fear".
With the advent of ChatGPT, the blessed humanoid robot is good at verbal communication, but it is still far from verbal communication, especially facial expressions.
In the future, if humans really want to live in a world full of robots, robots must have the ability to autonomously gain the trust of humans through facial expressions, just like humans.
Obviously, designing a robot that can not only make a variety of facial expressions, but also know when to behave, has always been a difficult task.
The innovative machine lab from Columbia University's School of Engineering has been working on this challenge for 5 years.
Recently, the research team unveiled a robotic emo that can predict human facial expressions and make expressions at the same time as humans.
The latest research has been published in the journal Science.
Address: https://www.science.org/doi/10.1126/scirobotics.adi4724
Emo's self-supervised learning framework, like a human looking in a mirror to practice facial expressions.
Interestingly, Emo even learned to predict 840 milliseconds in advance before a person smiles, and smile with humans at the same time.
This quick and timely facial response allows humans to feel the sincerity of the robot and the feeling of being understood.
Moreover, it can also make eye contact.
How can EMOs accurately predict human expressions?
The human-computer interaction revolution is coming
According to the research team, led by Hod Lipson, there are two major challenges that need to be addressed before developing the robot Emo.
The first is the hardware aspect, how to mechanically design an expressive multifunctional robot face that involves complex hardware and drive mechanisms.
On the other hand, it is a well-designed robot face, which needs to know what kind of expression to generate to make it look natural, timely and realistic.
And going a step further, the team hopes to train the robot to be able to predict human facial expressions and make those expressions at the same time as the human.
Specifically, the Emo face is equipped with 26 actuators that can present a wide variety of subtle facial expressions.
In addition to the actuator, Emo's face is designed with silicone skin, which is convenient for quick customization and maintenance.
For more realistic interactions, the researchers equipped the robot's eyes with high-resolution cameras.
Therefore, emo can also make eye contact, which is an important part of non-verbal communication.
In addition, the research team has developed two AI models: one predicts human facial expressions by analyzing subtle changes in the target's face, and the other generates movement instructions using the corresponding facial expressions.
To train the robot on how to make facial expressions, the researchers placed the emo in front of the camera and asked it to make random movements.
After a few hours, the robot learns the relationship between their facial expressions and motor commands.
The team called this "self-modeling," which is similar to what humans imagine themselves looking like when they make certain expressions.
The team then played a video of the human facial expressions for Emo, observing and learning from them frame by frame.
After a few hours of training, Emo can predict people's facial expressions by observing small changes in their faces.
Yuhang Hu, lead author of the study, said, "I believe that accurately predicting human facial expressions is a revolution in human-computer interaction (HRI). Traditionally, robots have been designed with human expressions in mind."
"Now, robots can integrate human facial expressions as feedback. When a robot and a human co-express themselves in real time, it not only improves the quality of interaction, but also helps build trust between humans and robots. In the future, when interacting with robots, it will observe and interpret your facial expressions just like a real person."
Next, let's take a look at the specific details of the design behind Emo.
Technical introduction
Mechanical control structure
The EMO is equipped with 26 actuators (below) that provide greater facial freedom to make asymmetrical facial expressions.
(1 & 2) Control the eyebrows with a magnet-attached linkage. (3) Upper eyelid. (4) Lower eyelids. (5) Eyeball linkage. (6) Eyeball frame. (7) Camera
(8 to 10 and 13) Mouth passive linkage. (11 and 12) Connecting rods of the 2D five-bar mechanism.
One of the main differences in the EMO design is the use of directly attached magnets to deform the replaceable facial skin. This approach allows for more precise control over facial expressions.
In addition, Emo's eyes have a camera embedded in them for humanoid visual perception.
These high-resolution RGB (red, green, blue) cameras, one within the pupil of each eye, enhance the robot's ability to interact with its environment and better predict the facial expressions of the interlocutor.
The eye module controls the movement of the eyeballs, eyebrows, and eyelids, as shown in the image above.
Each eyeframe is fitted with a high-resolution RGB camera. The eyeframe is driven by two motors on two axes, pitch and yaw, respectively by means of a parallelogram mechanism.
The advantage of this design is that it creates more space in the center of the eye frame, allowing the researchers to mount the camera module in a natural position that corresponds to the human pupil.
This design facilitates more natural face-to-face interactions between robots and humans.
It also enables correct and natural gaze, which is a key element of non-verbal communication in close proximity.
In addition to these hardware upgrades, the researchers also introduced a learning framework consisting of two neural networks – one to predict the facial expressions of the emo itself (the self model) and the other to predict the facial expressions of the interlocutor (the interlocutor model).
The researchers' soft-skin human-faced robot has 23 motors dedicated to controlling facial expressions and 3 motors for neck movements.
The entire facial skin is made of silicone and is attached to the robot's face with 30 magnets.
The robot's facial skin can be replaced with other designs for a different look and skin material.
Expression generation model
The researchers also proposed an upgraded inverse model that would allow the robot to generate motor instructions on the same computing hardware more than five times faster than the previous generation.
They propose a self-supervised learning process to train researchers' facial robots to generate human facial expressions without explicit action choreography and human labels.
Traditional methods of controlling robots rely on kinematic equations and simulations, but this only works for rigid body robots with known kinematics.
The robot has soft deformable skin and several passive mechanisms with four sleeve joints, so it is difficult to obtain the equations of motion for the robot's kinematics.
The researchers overcame this challenge with a vision-based, self-supervised learning approach in which the robot could learn the relationship between motor instructions and the resulting facial expressions by looking at itself in a mirror.
The robot's facial expressions are controlled by 19 motors, 18 of which are symmetrically distributed and one motor controls jaw movement.
In the researchers' case, the expressions in the facial dataset were all symmetrical;
As a result, symmetrically distributed motors can share the same motor instructions when controlling the robot.
Therefore, the actual control command only needs 11 parameters normalized to the [0, 1] range.
The face inversion model was trained on a dataset generated by the robot itself (below), which included motor instructions and resulting facial landmarks.
The researchers collected data in a self-supervised manner through a random process of "motor babbling". The process automatically removes motor commands that could tear facial skin or cause self-collisions before sending them to the controller.
After the servo motor reaches the target location defined by the instruction, the researchers used an RGB camera to capture an image of the robot's face and extract the robot's facial landmarks.
By combining a self-model and a predictive interlocutor model, the bot can perform collaborative expression.
Expression prediction model
The researchers also developed a predictive model that predicts the target facial expressions of the interlocutor in real time.
In order for the robot to be able to make realistic facial expressions in a timely manner, it must predict facial expressions in advance so that its mechanism has enough time to activate.
To do this, the researchers developed a predictive facial expression model and trained it using a video dataset of human expressions. The model is able to predict the target expression a person will make based on the initial and subtle changes in their face.
First, the researchers quantified facial expression dynamics using the Euclidean distance between each set of facial landmarks and the facial landmarks of the initial ("still") facial expressions in each video.
The researchers defined the still face landmark as the average landmark of the first five frames, and the target face landmark as the landmark that differed the most from the still face landmark.
The Euclidean distance of a static facial landmark is constantly changing and distinguishable from the Euclidean distance of the landmark of other frames.
Therefore, researchers can calculate the trend of expression change by the second derivative of landmark distance relative to time.
The researchers used the video frame at the time of the greatest acceleration of the expression change as the "activation peak".
To improve accuracy and avoid overfitting, the researchers enhanced each piece of data by sampling surrounding frames.
Specifically, during training, the input to the predictive model is to arbitrarily extract four images from a total of nine images before and after peak activation.
Similarly, the labels are randomly sampled from four frames of the image after the target face.
The dataset contains a total of 45 human participants and 970 videos. 80% of the data was used to train the model, and the rest was used for validation.
The researchers analyzed the entire dataset and concluded that the average time it takes for humans to make a facial expression is 0.841 ± 0.713 seconds.
The predictive model and inverse model (referring only to the processing speed of the neural network model used in the researchers' paper) run at about 650 frames per second (fps) and 8000 frames per second (fps) on the MacBook Pro 2019 without GPU devices, respectively.
This frame rate does not include data capture or landmark extraction time.
The researchers' robot can successfully predict the facial expressions of the target human and generate the corresponding motor instructions in 0.002 seconds. This time is left for approximately 0.839 seconds to capture facial landmarks and execute motor commands to generate the target facial expression on the face of the physical robot.
To quantitatively assess the accuracy of predicting facial expressions, the investigators compared the investigators' methods to two baselines.
The first baseline is to randomly select an image as a prediction in the inverse model training dataset.
The dataset for this baseline contains a large number of images of robot expressions generated by babbling.
The second baseline is a mimicry baseline, which selects the facial landmark at the peak of activation as the predicted landmark. If the activation peak is close to the target face, then this baseline is competitive with the investigator's approach.
However, the experimental results showed that the researchers' method was superior to this baseline, suggesting that the prediction model successfully learned to predict future target faces by inducting subtle changes in the face, rather than simply copying facial expressions in the last input frame.
Figure 4B shows a quantitative evaluation of the predictive model.
The researchers calculated the mean absolute error between the predicted landmarks and the ground truth landmarks, which consist of human target facial landmarks with a dimension of 113×2.
Tabular results (Table S2) show that the investigators' method is superior to the two baseline methods, exhibiting a smaller mean error and a smaller standard error.
The next step of EMO: connect the large model
With the ability to simulate and predict human expressions, the next step in Emo research is to integrate language communication into it, such as plugging into large models like ChatGPT.
As robots become more human-like, the team will also focus on the ethics behind it.
By developing robots that can accurately interpret and mimic human expressions, we are one step closer to a future where robots can be seamlessly integrated into our daily lives, providing companionship and assistance to humans, researchers say.
Imagine a world where interacting with robots is as natural and comfortable as talking to a friend.
About the Author:
Yuhang Hu is the corresponding author of the paper.
Currently, he is a PhD student at Columbia University, focusing on robotics and machine learning.
Resources:
https://www.engineering.columbia.edu/news/robot-can-you-say-cheese