Reports from the Heart of the Machine
Editors: Zenan, Boat
NLP is pushing AI into an exciting new era.
At present, the hottest direction in the field of artificial intelligence is pre-training large models, and many people believe that this research has begun to show results in the field of general artificial intelligence.
A well-known scholar in the field of natural language processing, Stanford University Professor Christopher M. Christopher Manning recently published an article titled "Human Language Understanding & Reasoning" in the AI & Society special issue of the Journal of the American College of Humanities and Sciences (AAAS), which explores the nature of semantics, language understanding, and looks ahead to the future of big models.
Manning believes that with technological breakthroughs in the field of NLP, we may have taken a firm step in the direction of Artificial General Intelligence (AGI).
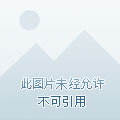
summary
Over the past decade, simple neural network computing methods have made huge and surprising breakthroughs in natural language processing, with people replicating successes at hyperscale and training on large amounts of data. The resulting pre-trained language models, such as BERT and GPT-3, provide a strong foundation for general language understanding and generation that can be easily adapted to many understanding, writing, and reasoning tasks.
These models show the first signs of a more general form of AI that could produce powerful underlying models in the realm of perceptual experience, rather than just language.
Four eras in the NLP space
When scientists think about AI, most of them first think of the ability to model or reconstruct a single person's brain. However, modern human intelligence is far more than the intelligence of a single brain.
Human language is powerful and has had a profound impact on our species because it provides a way for the population as a whole to network the brain. A person may not be much smarter than our chimpanzee or bonobo's close relatives. These apes have been shown to possess many of the hallmark skills of human intelligence, such as using tools and planning. In addition, their short-term memory is even stronger than ours.
The timing of human invention of language may always be a mystery, but it is relatively certain that in the long evolutionary history of life on Earth, humans have only recently developed language. The common ancestors of proto-monkeys, monkeys and apes date back about 65 million years. Humans separated from chimpanzees about 6 million years ago, and the history of human language is generally thought to be only a few hundred thousand years old.
After humans developed language, the power of communication allowed Homo sapiens to quickly surpass other creatures, although we were not as strong as elephants and not as fast as cheetahs. Only recently did humans invent writing (probably only over five thousand years ago) to allow knowledge to communicate across the boundaries of time and space. In just a few thousand years, this information-sharing mechanism has brought us from the Bronze Age to today's smartphones. High-fidelity code that allows for rational discussion and information distribution among humans, allows for the cultural evolution of complex societies, and gives birth to the knowledge behind modern technology. The power of language is the foundation of human social intelligence, and language will continue to play an important role in a future world where ARTIFICIAL tools augment human capabilities.
For these reasons, the field of natural language processing (NLP) emerged in tandem with the earliest developments in artificial intelligence. In fact, preliminary work on the NLP problem of machine translation, including the famous Georgetown-IBM experiment of 1954, enabled the first case of machine translation in history, slightly before the creation of the term "artificial intelligence" in 1956. In this article, I give a brief overview of the historical processing of natural language. I then describe recent dramatic developments in NLP that have come from large artificial neural network models trained on large amounts of data. I traced the tremendous progress made in building effective NLP systems using these techniques and summarized some ideas about what these models achieved and where they would go next.
To date, the history of natural language processing can be roughly divided into four eras.
The first era was from 1950 to 1969. NLP research begins with machine translation research. One imagines that translation can quickly build on the enormous success of computers in deciphering codes during World War II. Researchers on both sides during the Cold War sought to develop systems that could translate the results of scientific research from other countries. At the beginning of this era, however, little was known about the structure of human language, artificial intelligence, or machine learning. In retrospect, the amount of computation and data available was pitifully small. Despite the hype of the original systems, these systems only provided word-level translation lookups and some simple, not-very-principled rules-based mechanisms for dealing with the inflected form (inflection) of words and word order.
In the second era, from 1970 to 1992, we witnessed the development of a series of NLP demonstration systems that exhibited complexity and depth in dealing with phenomena such as syntax and references in human language. These systems include Terry Winograd's SHRDLU, Bill Woods' LUNAR, Roger Schank's, such as SAM, Gary Hendrix's LIFER, and Danny Bobrow's GUS. These are all rules-based systems that people build by hand, but they're starting to model and use some of the complexities of human language understanding. Some systems are even deployed for tasks such as database queries. Linguistics and knowledge-based artificial intelligence are developing rapidly, and in the second decade of this era emerged a new generation of hand-built systems that distinguished it from declarative and linguistic knowledge and its procedural processing and benefited from the development of a range of more modern language theories.
However, the direction of our work changed significantly in the third era between 1993 and 2012. During this period, digital text became richer, and the most applicable direction was to develop algorithms that could achieve some degree of linguistic understanding on a large amount of natural language content, and to use the presence of text to help obtain this ability. This led to a fundamental repositioning of empirical machine learning models around NLP in the field, a direction that still dominates today.
At the beginning of this period, our main method was to grasp a reasonable amount of online text —the collection of texts at that time was generally under tens of millions of words— and extract some kind of model data from it, mainly by calculating specific facts. For example, you may find that the types of things that people recognize are fairly balanced between a person's position (such as a city, town, or fortress) and a metaphorical concept (such as imagination, attention, or essence). But counting words only provides a language understanding device, and early empirical attempts to learn language structures from text collections were rather unsuccessful. This has led much of the field to focus on building annotated language resources, such as tagging words, instances of people's names or company names in text, or the grammatical structure of sentences in tree libraries, and then using supervised machine learning techniques to build models that can generate similar labels on new pieces of text at runtime.
Since 2013, we have expanded the empirical direction of the third era, but the work has changed dramatically due to the introduction of deep learning/artificial neural network methods.
In the new method, words and sentences are represented by positions in a space of real vectors (tens or thousands of dimensions), and similarities in meaning or syntax are represented by proximity in that space. From 2013 to 2018, deep learning provided a more powerful way to build high-performance models that made it easier to model contexts at greater distances, and models could be better generalized to words or phrases with similar meanings because they could take advantage of proximity in vector spaces rather than relying on the identity of symbols (such as morphology or part of speech). However, the approach has not changed in building supervised machine learning models to perform specific analytical tasks.
In 2018, everything changed, and the first major success of ultra-large-scale self-supervised neural network learning was in NLP. In this approach, the system can learn a lot of language and world knowledge by touching large amounts of text (now usually billions of words). Self-supervision of this is achieved by letting the AI system create its own prediction challenges from the text, such as continuously recognizing each "next word" in the text given a previous word, or filling in the text with a masked word or phrase. By repeating such predictive tasks billions of times and learning from mistakes, the model will do better the next time it is given a similar text context, accumulating a general knowledge of the language and the world that can then be deployed to tasks that are of interest to more people, such as Q&A or text classification.
Why big models are breakthroughs
In hindsight, the development of large-scale self-supervised learning methods is likely to be seen as a revolution, with a third era likely to extend into 2017. The impact of the pre-trained self-supervised approach is a breakthrough: now we can train on a large number of unlabeled human language materials, generating a large pre-trained model that can be easily adjusted by fine-tuning or hints, providing powerful results on a variety of natural language understanding and generation tasks. Now, there is an explosion of progress and attention about NLP. There is a sense of optimism, and we are beginning to see the emergence of knowledge instilling systems with some degree of general intelligence.
I can't fully describe the currently dominant neural network model of human language here. In general, these models represent everything through real vectors and are able to learn to represent a piece of text well by backpropagation from certain prediction tasks to errors in word representation (which boil down to calculus) after being exposed to a lot of data.
Since 2018, the primary neural network model for NLP applications has been the Transformer architecture neural network. Transformer is a more complex model than the simple neural network for sequences of words explored decades ago, and one of the main ideas is the attention mechanism — through which the representation of one location is computed as a weighted combination of representations from other locations. A common self-supervising goal in the Transformer model is to mask the occasional word in the text, which calculates the words that once existed on the vacancies. It does this by calculating the vector of queries, keys, and values representing that position from each word position, including the mask position. Comparing a query for a location with the value of each location, the algorithm calculates the attention of each location. Based on this, a weighted average of the values for all locations is calculated.
This operation is repeated several times at each layer of the Transformer neural network, and the resulting values are further manipulated through a fully connected neural network layer and generate a new vector for each word by using a normalization layer and residual connections. The whole process is repeated several times, providing an additional layer of depth for the Transformer neural network. Finally, the representation above the mask position should capture the words in the original text: for example, the committee shown in Figure 1.
What can be achieved or learned through simple computations of a Transformer neural network is not obvious, and at first it is more like some kind of complex statistical association learner. However, using very powerful and flexible hyperparameter models like Transformer and vast amounts of data to practice prediction, the model discovers and characterizes much of the structure of human language. Studies have shown that these models learn and characterize the syntactic structure of sentences and learn to memorize many facts that help models successfully predict masked words in natural language.
Moreover, while predicting a masked word may initially seem like a fairly simple and low-level task, the outcome of this task has a powerful and universal effect. These models bring together the languages they are exposed to and a wide range of real-world knowledge.
With just one further instruction, such a large pre-trained model (LPLM) can be deployed for many specific NLP tasks. From 2018 to 2020, the standard approach within the field is to fine-tune the model with a small amount of additional supervised learning, training it on the exact task of interest. But recently, researchers were surprised to find that the largest of these models, such as GPT-3 (Generative Pre-Trained Transformer), performs new tasks well with a prompt. Give the model a human language description or a few examples of what people want the model to do, and the model can perform many tasks that they have never been trained for.
The new paradigm of NLP brought about by the big model
Traditional natural language processing models are typically composed of several independently developed components, usually built into a pipeline in which first attempts to capture the sentence structure and low-level entities of the text, followed by vocabulary with high-level meanings, which are also actors fed into some specific domain. Over the past few years, the industry has replaced this traditional NLP solution with LPLM, often fine-tuned to perform specific tasks. We can expect what LPLM can accomplish in the 2020s.
Early machine translation systems covered finite language constructs in a finite domain. Building large statistical models from a wide range of parallel corpora of translated texts that cover machine translation led to the first launch of Google Translate in 2006.
Ten years later, at the end of 2016, when people switched to neural machine translation, Google's machine translation performance improved significantly. But new systems are being replaced faster and faster, and in 2020 Transformer-based neural translation systems were improved with different neural architectures and methods.
Instead of being a large system that translates between two languages, the new system leverages a giant neural network while being trained on all the languages covered by Google Translate, tagging different languages with only a simple token. While this system can still go wrong, machine translation is constantly evolving, and the quality of today's automated translations is already excellent.
For example, to translate French into English:
He had been nicknamed, in the mid-1930s, the Singing Fool, while making his debut as a solo artist after having created, in 1933, a successful duet with the pianist Johnny Hess.
For his dynamism on stage, agile silhouette, his wide and laughing eyes, his hair in battle, especially for the rhythm he gave to words in his interpretations and the writing of his texts.
He was nicknamed the Singing Madman in the mid-1930s when he was making his debut as a solo artist after creating a successful duet with pianist Johnny Hess in 1933.
For his dynamism on stage, his agile figure, his wide, laughing eyes, his messy hair, especially for the rhythm he gave to the words in his interpretations and the writing of his texts.
In a Q&A system, the system looks for relevant information in a set of text and then provides an answer to a specific question (rather than returning only a page that suggests relevant information, as was the case with earlier web searches). The Q&A system has many straightforward commercial applications, including pre-sales and post-sales customer consultation. Modern neural network question answering systems are highly accurate at extracting answers that exist in text, and are even good at finding out answers that don't exist.
For example, find the answer to the question from the following English text:
Samsung saved its best features for the Galaxy Note 20 Ultra, including a more refined design than the Galaxy S20 Ultra–a phone I don’t recommend. You’ll find an exceptional 6.9-inch screen, sharp 5x optical zoom camera and a swifter stylus for annotating screenshots and taking notes.
The Note 20 Ultra also makes small but significant enhancements over the Note 10 Plus, especially in the camera realm. Do these features justify the Note 20 Ultra’s price? It begins at $1,300 for the 128GB version.
The retail price is a steep ask, especially when you combine a climate of deep global recession and mounting unemployment.
How much does the Samsung Galaxy Note 20 Ultra cost?
128GB version $1300
Does the Galaxy Note 20 Ultra have a 20x optical zoom?
No
What is the optical zoom of the Galaxy Note 20 Ultra?
5x
How big is the screen of the Galaxy Note 20 Ultra?
6.9 inches
For common traditional NLP tasks, such as tagging people or organization names in a piece of text or categorizing text with emotional tendencies (positive or negative), the best current system is LPLM-based, fine-tuning specific tasks by providing a set of samples labeled in the desired way. Although these tasks were well done before large language models appeared, the breadth of language and world knowledge in large models further improves performance on these tasks.
Finally, LPLM sparked a revolution in the ability to generate fluid and continuous text. In addition to many creative uses, such systems have utilitarian uses, such as writing formulaic news articles and automatically generating summaries. In addition, such a system can help radiologists diagnose the condition by proposing (or summarizing) key points based on the radiologist's findings.
These NLP systems perform very well on many tasks. In fact, given a specific task, they can often be trained to perform those tasks like humans. Still, there's reason to wonder if these systems really understand what they're doing, or whether they're just simply repeating some of the operations that don't make sense.
Take the more complex understanding of programming languages, for example, the meaning of words described in programming languages is mainly through denotational semantics: the meaning of a word, phrase, or sentence is a collection of objects or situations in this way, in which the world or its mathematical abstraction is described. This is in stark contrast to the simple distribution semantics (or use of meaning theory) in modern experimental studies in NLP, where the meaning of words is no longer just a description of context.
Do big models really understand human language?
I think the meaning of language comes from understanding the network of connections between the form of language and other things. If we have a dense network of associations, then we can understand the meaning of the linguistic form very well. For example, if I knew that "shehnai" was an Hindi word, then I would have a reasonable idea of what the word meant, it was Hindu, and if I could hear the sound of the instrument playing, then I would have a richer understanding of the word shehnai.
Conversely, if I have never seen or heard the sound of shehnai, but someone has told me that it is like a traditional Indian oboe, then the word also has some meaning for me: it is related to India, related to wind instruments, and used to play music.
If someone adds that shehnai has holes, multiple reeds, and horn-shaped ends like an oboe, then I have more of a network of properties connected to the shehnai object. Instead, I may not have this information, only a few paragraphs of context for using the word, for example:
Since a week ago, someone was sitting in the bamboo forest at the entrance to the house playing shehnai; Bikash Babu did not like shehnai's wailing, but was determined to meet all the traditional expectations of the groom's family.
Although in some ways I would have less understanding of the meaning of the word shehnai, I still know that it is a pipe instrument, which is also based on my knowledge of some additional cultural connections.
Thus, understanding the meaning of language includes understanding the associated network of language forms, and pre-trained language models are able to learn the meaning of language. In addition to the meaning of the vocabulary itself, the pre-trained language model also has a lot of practical knowledge. Many models have been trained in encyclopedias, and they know that Abraham Lincoln was born in Kentucky in 1809; he knew destiny's Child's lead singer was Beyoncé.
Just like humans, machines can benefit greatly from human knowledge repositories. However, models' understanding of word meanings and world knowledge is often very incomplete and needs to be enhanced with other sensory data and knowledge. Large amounts of text data first provide a very accessible way to explore and build these models, but it is also necessary to extend to other types of data.
The success of LPLM in language understanding tasks and the exciting prospect of extending large-scale self-supervised learning to other data patterns such as vision, robotics, knowledge graphs, bioinformatics, and multimodal data indicate the promise of a more general direction. We propose the terminology base model of a generic class model that trains millions of parameters on large amounts of data through self-supervision and can then be easily adapted to perform a wide range of downstream tasks. Examples such as BERT (bidirectional encoder representation from Transformers) and GPT-3 are early examples of such a foundational model, but more extensive work is now underway.
One direction is to connect language models with more structured repositories of knowledge represented as knowledge graph neural networks or large amounts of text to be consulted at runtime. But the most exciting and promising direction is to build a foundation model that can also absorb data from other senses from the world for integrated multimodal learning.
An example of this is the recent DALL-E model, which, after self-supervised learning of the corpus of paired images and text, can express the meaning of the new text by generating corresponding pictures.
We're still in the early days of the basic model era, but here I'll outline a possible future: Most information processing and analysis tasks, and possibly even things like robot control, will be taken over by a specialized version of one of a handful of base models. These models are expensive and time-consuming to train, but it will be very easy to adapt them to different tasks. In fact, one might be able to do this simply using natural language instructions.
This convergence on a few models carries several risks: the institutions that can build these models may have too much power and influence, and many end users may be affected by the biases in these models, and it is difficult to judge whether the models are correct. In addition, the security of use in a particular environment is also questionable, because the model and its training data are very large.
Either way, the ability of these models to deploy the knowledge gained from large amounts of training data to many different tasks will make them very powerful, and they will also become the first artificial intelligence to perform many specific tasks, only needing human instructions to tell it how to do it. While these models may end up knowing only a vague amount of knowledge, their possibilities may still be limited and lack the ability to fine logic or causal reasoning at the human level. But the universal validity of the underlying models means they will be very widely deployed, and they will allow people to see a more general form of AI for the first time in the next decade.
https://www.amacad.org/publication/human-language-understanding-reasoning