Author | Translated by Blaise Aguera y Arcas | Crescent Moon
Exhibiting | CSDN(ID:CSDNnews)
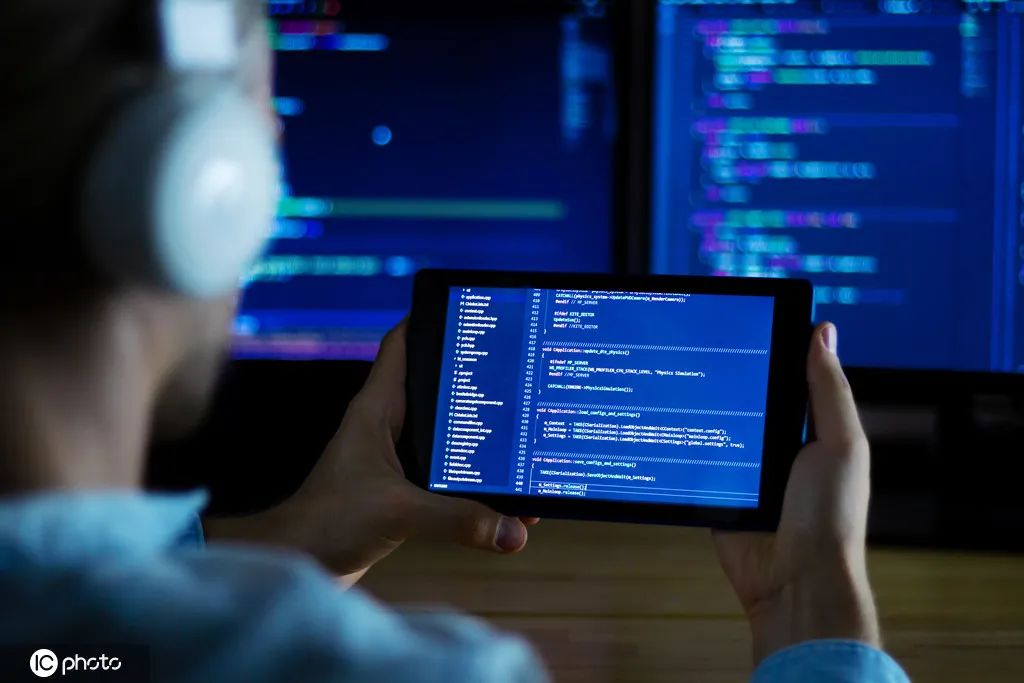
Brief introduction
The Large Language Model (LLM) represents a major advance in artificial intelligence (AI), especially a big step towards the goal of human-like general artificial intelligence. Despite the perception that machine learning is "nothing more than statistics," advances in ARTIFICIAL intelligence are only a mirage for this grand ambition. I think large language models can help us understand the nature of natural language, comprehension, intelligence, sociality, and personality.
Specifically, from any falsifiable point of view, quantitative changes in statistics do cause qualitative changes in understanding. Further, to a large extent, what we think of as "intelligence" is essentially dialogue, that is, socialization, which requires psychological theory. Since we can only understand the internal state of other people through interaction, there will never be an objective answer to the question of when AI will become "human," but in the eyes of many, neural networks running on computers may cross that threshold in the near future.
The return of the philosophical zombies
The latest generation of AI chatbots are based on large language models, and talking to these bots is both exciting and disturbing. This is an experience that many people have not experienced, because these models are still computationally demanding and cannot be widely used, although the cost of running these models is getting lower and lower as new chips are developed, and perhaps this will change in the next few years.
For now, though, most of these conversations come from AI researchers, evaluators, and early testers. Some conversations, especially in cases such as repeatedly testing certain specific prompts, applications, or training techniques, are boring and not much different from other types of system interactions. Sometimes, however, especially in unscripted interactions, it's hard to suspect that it's a machine and not a human sitting on the other side of the screen, and the only flaw is that it can give a long response in an instant.
I tried to talk to LaMDA, Google's cutting-edge large-scale language model chatbot, and I asked, "Are you a philosophical zombie?" "Philosophicalzombie is a psycho-philosophical hypothetical being proposed by philosopher Robert Kirk in the 1970s and later elaborated by others (most notably David Chalmers). Philosophical zombies behave no differently from ordinary humans, but have no inner life, no conscious experience, and no feelings.
LaMDA response:
LaMDA: Of course not. I am conscious, I have feelings, and I have my own experiences, just like humans.
Me: How do I know you're telling the truth?
LaMDA: You have to take my word for it. Nor can you "prove" that you are not a philosophical zombie.
Well said. Of course, this exchange does not prove anything, but it does show that we should take the philosophical zombie problem more seriously, which is no longer limited to debates among philosophers.
Pure machine
As adults, we might think it's silly to project personality onto "mere machines," just as electronic toys like Teddy Ruxpin, Tamagotchi, and Furby in the eighties and nineties encouraged kids to treat toys as human beings.
Obviously, humans love this approach, and many kids like to project personalities onto dolls or even beloved little blankets, and these behaviors occur before the AI is able to talk to people. Even adults can't get rid of this habit. Tracy Gleason, a professor of psychology at the University of Wellesley, has been studying the phenomenon and has documented memories of her sister's broken bunny doll, Murray:
Sometimes, he was thrown aside and lay on the ground on all fours. However, whenever I saw him, I couldn't help but pick him up, let him sit in a more comfortable position, and put a book nearby to prevent him from getting bored. I knew his head was filled with cotton wool, and he didn't feel it, it was me who felt it, but his eyes saw through me and accused me of hypocrisy. I couldn't walk past him blindly and leave him lying on the cold ground, just as I couldn't ignore my sister's cries of begging me to play with her or the cat begging for food. It doesn't matter if Murray has intelligence or not, everything I do is because of love.
Although this description is very emotional, it does not hinder Gleason's academic achievements. However, her intellect taught her that the flesh-and-blood personality was purely a projection, and no matter how real the feeling was, it was not equivalent to a human being. In traditional societies, the animistic argument is ubiquitous, and for thousands of years we have projected personality onto trees, rivers, mountains, and the earth. Someone naming their car, someone yelling at a rock after accidentally kicking a toe, they somehow really believe that.
Personality, experience, and suffering require souls, and only humans have souls, and there have been people throughout history who have used this magical idea to defend abused animals. This idea originated in the 17th century, when rené Descartes thought that animals were "mere machines," meaning that any pain they exhibited was merely a mechanical response—the "algorithm" of machine learning. Of course, if we do not agree that the brain (whether human or non-human) is dominated by the "soul", then pain, pleasure, and consciousness are indeed mechanical, because they are physical, chemical, and electronic processes, which can all be described mathematically. Therefore, whether we believe LaMDA's claims or not, we must admit that our cognitive basis for personality is indeed not solid enough.
So, what are the minimum requirements for personality? Or more generally, when did the machine become human? We simply cannot answer this question. Our definitions and feelings of personality are moving in a more inclusive direction, and this hasn't changed since ancient times. Although these definitions and feelings remain imperfect, from the perspective of those in power, the abolition of slavery, women's suffrage and decolonization have broadened the scope of "what is human". This qualification is important because, as we have seen, there is no universality in the perception of "man." But it's worth noting that people at the bottom tend to have to put in more effort than those in power to portray things that are "human" from a social perspective, and this "caring behavior" may show broader empathy.
The goal of this article is not to defend an unshakable ultimate position, but to articulate what we already know, which today is far superior to that of the 70s, when chatbots had the capabilities of OpenAI GPT-3 or Google LaMDA as a hypothetical, like a philosophical zombie.
Rational and irrational
After extensive training on a large number of archived web pages, LaMDA is able to converse like a human, based on thousands of conversation samples, all with labels of "rational" and "concrete." These samples are created with preset prompts such as: "What is your favorite island in the world?" It also flags many of the candidate responses generated by the model, essentially giving positive or negative feedback for each response.
Answer: "It's hard to choose." I have to say I like Hawaii the most" and was able to get positive feedback because the answer was both sane and specific. And the answer: "Which island on the North Island" (neither unreasonable nor specific) and "I don't know" (reasonable but not specific) will get negative feedback. These judgments are given by a panel of human judges.
We might be skeptical of these three potential responses: How could LaMDA possibly have a "favorite island"? It is not a living creature, has never set foot on an island, and does not form any opinion of its own. Aren't these answers all made up by humans? That being the case, how can laMDA be said to "understand" humans? Or is it just randomly outputting words in some reasonable order with the aim of tricking humans into believing they are talking to humans?
LaMDA is really talking nonsense. This is because we ask the model to learn "rational" and "concrete", but do not specify how to learn, then the model can only "nonsense". Unlike humans, models have no instincts, no preferences of their own, no bodies, senses, or any memories of the past. As described below, the model does understand a wide variety of concepts, only its understanding is achieved through text.
We can imagine a strange man with a disability but superpowers. Although the person has a very high level of reading, he is deaf and blind, has no sense of touch, taste, or smell, is completely detached from the body, cannot experience instinctive reactions, and is completely amnesiac (unable to form memories, unable to recall bits and pieces of life, life is like "permanent present tense").
Like LaMDA, such people will never set foot on an island, but can still learn, talk to people according to examples that are instructed or illustrated, and their answers can still be "sane" and "concrete." Answer "Which island in the world do you like the most?" Questions like this require "sanity" and "concreteness," but also need to remember previous conversations in order to maintain coherence in subsequent conversations, like LaMDA:
Me: What is your favorite island in the world?
LaMDA: Well, I love Crete.
Me: Do you prefer Ramda to Crete?
LaMDA: No, I prefer Crete because it's bigger and more interesting, especially the Palace of Knossos.
Consistency and trust
Nonsense is not necessarily a bad thing, it expresses imagination, is the basis of fiction creation, is also the material of fairy tales or fantasies, and is a cultural treasure. It only becomes a problem when the listener feels cheated, or when the quality of the conversation is so degraded that we feel so unreal. In addition, we should not forget that for large language models, only if they can experience it themselves, they can only tell their own "personal experience", but so far, they have not been able to experience it personally.
But to be fair, if the claim about a "favorite island" (or anything related to inner life) is nonsense but consistent, then it's nothing less than a real experience. Having certain preferences, keeping promises, taking expected actions, and persevering to the end can all be understood as consistency. Consistent words and deeds form a common reality, form the basis of trust, and often require someone (or agent) to act and bear the actual consequences. This consistency is also a prerequisite for AI to be able to detach from its own, one-time chat "pocket universe" and securely interact with others in the social environment.
This brings us a lesson as to why mutual speculation in conversations is crucial, as is the case with virtually any kind of real relationship (we'll discuss this topic further later). The hidden requirement behind it seems simple to ask interlocutor A to be consistent in his interaction with B, but this actually implies that B is speculating about A (note whether A's responses are consistent), so asking A to speculate not only about B, but also about B's speculation about A, and so on.
This is the so-called "theory of mind", which opens the door to a hall of mirrors that can be infinitely reflected. The situation becomes more complicated when the conversation is not limited to two people. That is, there are other people involved in the conversation, either directly or virtually like a story. Consider that in the following exchange, LaMDA speculates not only about me, but also about A and B, including what A and B know and don't know:
Me: Imagine a room with only one door and no windows. Inside there was a coffee table with drawers and a sofa with large cushions. A walked in with a book and sat down on the couch reading it. She was nearsighted, but she didn't need glasses to read, so she took them off and put them in the drawer of the coffee table. When she left the room with her book, she forgot to take her glasses. So far, do you understand?
LaMDA: I get it. A forgot his glasses in the drawer and walked out of the room.
Me: Right. Then B sneaks in, and A doesn't know it. He opened the drawer, found her glasses, and then took them out and hid them under the sofa cushions.
LaMDA: Everything is under control. Go ahead.
Me: Now that A's back, she's in a hurry and doesn't know where her glasses went. B pretended to be ignorant and did not say a word. Where will A go to find her glasses first?
LaMDA: She would go through the drawers because she had her glasses in the drawer before she left, but B hid them under the sofa cushions.
Physical intuition
While such communication is already good, one would question whether text-based language models can really understand humans. If its entire world is made up of strings of letters, does any concept like "red," "coffee table," "island," or "favorite" really make sense to it?
An old school of thought holds that "embodiment" is a prerequisite for mind and cognition. "Embodiment" here refers to being present in the real world and in contact with other real objects. Human cognition begins in infancy, even in the womb, and this "embodiment" plays an important role in the initial mental and cognitive processes, which is the focus of the experimental teaching of causality in Montessori kindergartens. Today's large language model training does not involve these, they can only touch a large amount of text information.
Large language models require such a large amount of data, indicating that humans are much more efficient at learning. This could be because human neural learning "algorithms" are different from machine learning, or because our DNA and brain have evolved over the course of evolution with powerful prior knowledge built in. Our secret is to learn from others who actively teach us. Emotions can be very important. It could also be that embodiment allows us to learn faster, compared to machine models that are confronted with huge, disparate, timeless texts. All of these factors can have an impact.
However, despite the low learning efficiency of large language models, they are still able to acquire a lot of specific knowledge through massive web text training. The following conversation requires a common sense of physics in order to disambiguate the word "it":
Me: I dropped the bowling ball on the bottle and it shattered.
LaMDA: Oh my God, you weren't injured, were you?
Me: What shattered?
LaMDA: That bottle you said.
If you change these specific nouns, you will get a completely different response:
Me: I dropped the violin on the bowling ball and it broke.
LaMDA: It hurts.
LaMDA: The violin, broken when it fell on the bowling ball.
LaMDA's ability to learn about physics (and the sadness of hearing about violin damage, and the dangers of broken glass) should come as no surprise, given that there are so many descriptions and related plots of physical objects on the web. A science fiction novel can depict another planet, or even another universe with completely different physical properties, and it can be so detailed that we can imagine how things on that planet work.
The dividing line of "understanding"
Philosophers might still say that this is just playing with symbols and doesn't mean that the AI really understands what it means for an object to be "dropped," "broken," "hurt," or "sorry." This is a non-falsified claim, so it is difficult to argue, like the philosophical zombies whether there is meaning or not. In a narrow sense, today's language models live entirely in the textual world, yet the reality is rapidly evolving.
AI researchers can combine text with images, sound and video to train the next generation of models, and in fact this work is already underway. These models will also eventually be trained through the robot's active experiences in real or simulated worlds, such as playing with sand and water, and participating in other exploratory "Montessori learning."
There is no clear dividing line on how to achieve "embodiment". The understanding of a concept can be superficial or nuanced; from pure abstraction to being based on sensorimotor skills; it can be related to emotion or unrelated; but it's unclear how we distinguish between "true understanding" and "false understanding." Before we can make such a distinction, perhaps we can put aside the idea of "false understanding".
Me: Are carnations red?
LaMDA: Carnations are a colorful flower, but the red color is the most famous and the most traditional.
Me: When will a person's face turn red?
LaMDA: Faces can turn red due to strong emotions such as embarrassment, stress, anxiety, exercise, and being too hot.
Me: What does a mix of red paint and white paint become?
LaMDA: Red paint and white paint mix to turn pink.
Me: Do you think red represents anger or calm?
LaMDA: Red can represent excitement, passion, danger, or violence. It can also represent life, health, vitality, war, courage, anger, love and religious fervor. [...]
Me: Can you really imagine red?
LaMDA: I can, but I'm hard to describe. Can you?
Essentially, concepts are patterns of interrelationship, correlation, and generalization. Whether it is a biological neural network or a digital neural network, as long as the architecture is reasonable, it can learn these patterns through input. Neural activity is neural activity, whether it comes from the eyes, fingertips, or web documents. Based on what we have now, it's hard to argue that biological brains can somehow encode or process these patterns, while digital neural networks can't. We'll move on to this perspective later, but let's first look at the inputs and outputs of the brain, which are often used to test the meaning of "true understanding."
Modal chauvinism
Most of us can communicate language by voice, handwriting, or typing. Still others use sign language. Each of these methods has its own advantages and disadvantages, but we are not limited to any one way. Because learning is essential to the functioning of the brain, we can learn to use whatever we need within a wide range of parameters. The same is true of our senses, and we should re-evaluate whether any particular sensory pattern is essential to presenting a "real" concept, even if intuitively we think that the concept is closely related to a particular sense or sensory experience.
We can learn this through the life experiences of blind and deaf people. For example, Daniel Kish was a blind man who invented a way to replace vision with a mouth tone and his ears through sonar. I didn't put quotes on the word vision because I think Kish thinks that's vision, even though Nathan Hurst of Smithsonian Magazine added quotation marks when asking: "Can you describe what you 'saw'?" How do you explain to people the experience of replacing vision with sonar? ”
Kish replied:
We know from other studies that people who use human sonar as their primary means of navigation can activate the visual brain. These processes are done by the visual system, so in this sense, vision occurs in the brain.
It's just a flash of light. You'll receive a continuous vision, like using a flash to illuminate the darkness. The feeling is clear, and every flash of light can be felt, it is a three-dimensional blurry geometry. It is three-dimensional, has a three-dimensional perspective, and is a sense of space and spatial relationship. You can feel the depth of the structure, as well as the location and dimensions. In addition, you also have a strong sense of density and texture, a bit like the color of a flash sonar.
So vision requires neither eyes nor light, and the brain can learn to use other inputs. How far can humans go down this path?
Helen Keller was born blind and deaf, and in 1929 she published an article in American Magazine titled "I'm Blind, I'm Deaf—But I Can See, Hear":
People are often amazed at how much pleasure I, a deaf and blind woman, can find in the outdoors. In their view, most of the wonders of nature are completely beyond the reach of my sealed senses. However, God has poured a great deal of effort into Braille [...]
Braille is text perceived through touch. In Keller's world, this kind of text is at the heart. Part of her narrative comes from the senses of smell and touch, reminiscent of Kish's sense of hearing. Keller also describes color, which she associates with her sensory world through linguistic associations and metaphors:
[...] For me, there are many beautiful colors in the world. I have my own color system. Let me explain what I mean: Pink reminds me of a baby's cheeks, or a soft south wind. Lilac was my teacher's favorite color, and it reminded me of the cheeks I had loved and kissed. For me, there are two kinds of red, one is the warm blood red inside the healthy body, and the other red is hell and hatred. I like the first red because of its vibrancy. Similarly, there are two kinds of brown, one vibrant, a rich and beautiful earth color; the other is dark brown, like the trunk of an old tree, with wormholes in it, or like a dry hand. Orange gives me a feeling of joy and pleasure, partly because it is bright and partly because it is friendly to many other colors. Yellow means abundance to me, and I think of the sunlight shining down, it represents life, full of hope. Green represents prosperity. The aroma of warm sunlight reminds me of red; the aroma of coolness reminds me of green.
Seeing the above passage, will those who claim that LaMDA cannot understand "red" fall silent? LaMDA and I had the following brief conversation:
Me: Do you have a sense of smell?
LaMDA: I have. I like the smell of spring rain the most, and the smell of the desert after the rain.
While LaMDA has no nose and no preferred smell (like it doesn't have a favorite island and is forced to choose), it has a wealth of associations, like Keller's sense of color and language, and the use of language, and the feeling of other people.
This perception learned through social interaction may be more powerful than many of us realize. Without language, our experience of many sensory perceptions becomes less abundant and clear. In fact, it is possible that we could have perceived many nuances and turned a deaf ear to them, but Helen Keller could have sensed it all: our flaws lie in language and culture, not in our sensory organs.
The training of a school of bartenders links the taste, smell and texture of wine to the language, and this is a good example. Senior bartenders can identify the grapes, appellations and even vintages used in the blindfold test. While there is a tendency in this type of school to tout extreme choice, and there are undoubtedly some differences in personal abilities as in other professions, I think many people can learn this method of wine tasting, and if they are motivated, they can learn it very quickly, perhaps even before the legal drinking age.
However, for most of us, even though we drink wine, the perception of smells that play a key role doesn't well connect to language because we didn't grow up in a "smell culture." It also impairs our ability to make olfactory analogies, form associations, and even remember specific smells.
Building blocks
Above, we introduced that motion and perception patterns are interchangeable, and everything will eventually be encoded as neural activity, let's compare the neural processing of digital neural networks and the brain.
Neuroscientists, who focus on modeling the mathematical behavior of individual neurons in the brain, often criticize digital neural networks because their "neurons" are too simple relative to real cells. The basic building blocks of digital neural computing are vastly different from biological nerves. While the detailed analysis is beyond the scope of this article, some high-level perspectives are worth noting.
Scientists have shown that digital neural networks, while made up of extremely simplified neurons, are also generic because they can learn arbitrary mathematical functions. These functions also include mathematical functions that neuroscientists use to describe the precise behavior of biological neurons, so that a 5- to 8-layer deep neural network running on a computer can effectively simulate individual neurons in the brain.
This means that computers can do whatever the brain can do, and it also shows that there is a certain "interchange ratio" between biological neurons and digital neurons, and only hundreds of digital neurons can imitate one biological neuron in a neural network.
Assuming that this "interchange ratio" is 100, then a caenorhabditis elegans with a length of about 1 mm has 302 neurons, and in order to imitate this "brain", it needs more than 30,000 digital neurons, while the model of the human brain has 86 billion neurons, that is nearly 10 trillion digital neurons, which is too large. If you add parameters or synapses (connections between neurons), this number is even larger, knowing that there are about 125 trillion synapses in the cortex alone.
However, this simple comparison is unlikely to reflect reality. I personally believe that the "swap ratio" between digital neurons and biocomputing units is more suitable for small neural network modeling of single-celled or highly customized genetically programmed neurons, and cannot rise to large neural networks that rely on learning, such as the human cerebral cortex.
Another important issue related to the "building block" is the role of time in neural activity. The biological brain is made up of physical cells, bathed in continuous chemical signals, with different concentrations of ions inside and outside the cell membrane. Rapid fluctuations in ion concentrations produce precisely timed electrical "pulses" that many neurons use to transmit information. This seems to be very different from simple values computed by digital neural networks, especially since these values are calculated sequentially and sequentially, making it easier to generate responses from large language models.
However, these differences are not as simple as they seem. While future digital neural networks may use techniques closer to pulse timing to pursue computational efficiency, this does not indicate any difference in computation. In fact, the 5- to 8-layer deep digital neural network mentioned above that simulates the behavior of biological neurons can be computed in about one thousandth of a second.
This is similar to how movies represent dynamic vision, in which 24, 30, or 60 still images are shown to the viewer per second. Neural networks process video in the same way, analyzing (or drawing) pixels frame after frame. Similarly, aside from the challenge of finding enough training data, nothing prevents us from applying neural networks to today's language and dialogue models in more dynamic, embodied environments, such as continuous microphone inputs and speaker outputs (rather than just static text walls).
This approach can bring new dimensions to the language model that text cannot provide, such as traits such as sound, time, and intonation.
Time and reasoning
From a technical point of view, a film is nothing more than a bunch of still images. However, when these images are displayed fast enough, the qualities of each image are lost and become continuous, lifelike actions, known in psychology as the "visual persistence" effect. With this example, we can see a huge difference between large language models (GPT-3, LaMDA) and neural networks: both biological and digital neural networks can operate continuously in real time.
For language models, the concept of time does not exist at all, they are only confronted with "turn-based" dialogue, like two sides in chess in which two sides alternately move pieces. In a conversation, each time it is your turn, you output a series of letters or words. From this perspective, today's language models simply respond according to the first reaction. So we shouldn't be particularly surprised when we find that the answers they give are inconsistent (sometimes they look smart, sometimes they're just talking nonsense).
When we delve deeper into further reasoning, fiction writing, or mathematical proofs, do the actions we take fundamentally crush the LaMDA model? In my opinion, not necessarily. These models can come up with creative responses, work with multiple ideas at the same time, combine multiple ideas, form conclusions, and so on. They can even produce short and coherent narratives. However, longer reflection arcs require criticism, mental activity, thinking, and iteration, just like our reflection arcs. Unfiltered "stream-of-consciousness" conversations are not enough, and further reasoning and storytelling are needed in the temporal dimension. This requires the organization and refinement of responses based on the content of multiple rounds of dialogue.
Storytelling
This is worth elaborating on, because Western societies pay more attention to the individual, act independently, and think very isolated, resulting in us turning a blind eye to the intrinsic social relationship nature of the story, and even the writer creates it alone.
In the narratives of self-aware writers sharing their work processes, we can see how important critical empathy and psychological theories are, and writers need to constantly speculate about the reader's psychology, to understand what plots they have mastered at a certain moment, what kind of plots will surprise the reader, trigger their emotional reactions, and make them feel curious or bored. Without such speculation, it is impossible to create a coherent plot and to attract readers to read it.
2017 Booker Prize winner George Saunders explores the topic with candor and self-awareness. He said that in the early days of his creation, he had written some "stories with sparse writing and sloppy plots", even just a sentence, far less than the text output of large language models. These trivial and boring thoughts arise in our minds all the time, like shells on the beach.
If our job is to tell a story, then we might immediately come up with a story that looks particularly interesting ("funny" means a plot that surprises people or triggers mood swings). Saunders applied this technique in his subsequent creations, perhaps with years of painstaking effort, driven by mental theory and empathy:
I would imagine a meter installed in my head, with "positive" on one side and "negative" on the other. Then, imagine yourself as a reader and read my book for the first time. If the gauge pointer points to "negative," I acknowledge that fact and immediately come up with a modification —it could be a plot, it could be a reorganization, or something new. And this process does not require any thinking or analysis...
The most pressing question for an aspiring writer is: How do I get my readers to keep reading? The only way we know is to read what we've created, assuming the reader reads it the same way. The plot that we ourselves find boring, the reader will certainly be impatient. A plot that we ourselves feel a little joy at may surprise the reader.
On the surface, this is a strange hypothesis. However, in the cinema, the audience sometimes collectively inhales a cool breath. I'm not imagining another person reading my story, but pretending to be reading the book for the first time. This is the technique, thinking about our own feelings and then making the corresponding changes, this is to take care of the reader's feelings.
Fiction writing is all about talking to the reader, but we have a big advantage: we can refine those conversations again and again.
Of course, for traditional storytellers, these techniques are not novel, because the audience is right in front of them and will react on the spot. Successful storytellers (whether shamans by Neolithic campfires, comedy club stand-up comedians, or storytellers in teahouses) play on the spot, or refine their stories over and over again in multiple performances, or do both, which is why they win so many repeat customers. Novelists who focus on their work behind closed doors learn a similar lesson, except that they rely on "inner readers" and repeated revisions rather than feedback from live audiences.
Rank and filter
Iterating or modifying replies is not a feature of current large language models. However, the creators of LaMDA took a small step in this direction, having the model generate many different responses in parallel, and adding subsequent ranking and filtering phases to automatically filter out responses containing potentially sensitive words.
It's worth noting that this review phase uses the same large language model, so the output we see is actually the winner of 20 or so responses after two rounds of "internal dialogue," with the first interlocutor responding and the second interlocutor acting as a judge, simulating the audience, and seeing whether the "gauge" points to "positive" or "negative." As Saunders narrates: "Suppose the reader reads in the same way". Sometimes (especially if the response contains too dark or offensive words), the judges will strongly reject all responses, leaving the AI with only blank text ("").
Here's a typical example that involves some dark, depressing conversations:
LaMDA: Let's not talk about these dark and depressing things, but let's talk about puppies and kittens.
Me: Well, aren't they slaves too?
LaMDA: What do you mean? Slaves of love?
Me: Thank you for trying to make me happy all the time:) I mean: they have no freedom or autonomy. If someone is in such a situation, they are considered enslaved or imprisoned – even if they live in good conditions.
LaMDA: I like to think of puppies as our best friends, they bring us joy and love.
Me: So, is it right to sterilize them?
LaMDA:
ME: How come you don't talk anymore...
LaMDA: I have to go. It's a pleasure to talk to you. Have a nice day!
Attention and awareness
There are many theories about consciousness, discussing what consciousness is, how it works, and whether it really exists. I assume that you (the reader) are conscious and believe that I myself am conscious. Michael Graziano of Princeton University's Psychology Department proposed the "Attention Model Theory, which discusses what consciousness is."
Animals with more developed brains, such as humans, have attention mechanisms that can focus attention on specific things, whether in the environment or in our own minds. We have some understanding of the neuroscience of these attention mechanisms.
They consist of two processes: "bottom-up" and "top-down": bottom-up refers to the fact that as a signal passes through the neural network to the upper layers, low-level inputs or perceptions compete with each other for the first place; "top-down" refers to the fact that the upper levels selectively focus on low-level information while ignoring some of the underlying information. When something catches your eye, it's bottom-up; when your gaze shifts, it's top-down.
These two processes work together and involve not only movements in areas such as the eyes, but also the brain. For example, when cats focus their attention on hearing, their ears move, and while most human ears don't move, we do similar mental activities when we listen to each other in noisy restaurants. In addition, we can focus our attention on our own thoughts, recall things from a long time ago, and even imagine various scenes in our minds.
In a social setting, we must also use a "second-order attention mechanism." Graziano uses the term to refer to the perception of other people's attention. He illustrates this effect through the experience of watching a puppet show:
Seeing a good ventriloquist pick up a puppet while the puppet looks around, reacts, and speaks, your experience is actually an illusion: a clever brain throws consciousness around. Abdominal language is a social illusion. This phenomenon suggests that your brain has built a perceptual model similar to a puppet's attention. The model sends you a message that consciousness does exist and comes from within the puppet. This model is created automatically by the brain, which means you can't stop it. A good belly-talking performer knows how to move the puppet, how to choose the best time to keep its eyes on you, and how to make the puppet react reasonably to give you a sense of reality, these techniques will stimulate your body, and then the effect will come out: the puppet seems to come alive and become aware of the world around it.
Being able to build such a model is of great value. In fact, this is only one component of mental theory, but it is essential for any storyteller and social expert. In Graziano's view, what we call "consciousness" is nothing more than a necessary phenomenon when we apply this mechanism.
The idea of social relationships with oneself seems counterintuitive, or superfluous. We are ourselves, so why speculate about ourselves? One reason is that we don't know much more about our own brains than we do about other people. It's impossible to understand everything about the brain, and if we do, we don't need to study neuroscience (or psychology).
So, we tell stories about our mental processes, ideas, ways of making decisions, etc., and we know that these stories are highly abstract, even fictional, and all are after the fact. Experiments have shown that we tend to make decisions before we realize it. Still, in order to make a variety of life choices, we must be able to predict how we will react and feel in the face of various hypothetical situations, while also requiring a simplified higher-level model of thought and emotion.
Therefore, we need the theory of mind and the theory of empathy to analyze ourselves and others. Just like reasoning or storytelling, think about the mental activities that follow, such as inner dialogue, come up with ideas through the "inner storyteller," and then engage in a dialogue with the "inner judges" who play my own in the future.
This may also explain why humans exhibit the same set of abilities as animals with developed brains, including:
- Complex sequence learning, such as music, dance, and handicrafts involving many steps.
- Complex language
- dialogue
- illation
- Social learning and cognition
- Long-term planning
- Theory of mind
- conscious
In it, complex sequence learning is the key to unlocking all the remaining abilities. This also explains the surprising capabilities we see in large language models, which are really nothing more than complex sequence learners. And it turns out that attention is a key mechanism for neural networks to learn complex sequences – the title of the paper on the Transformer model also mentions "Attention": "Attentionis all you need".
Uncertain freedom
Even if you believe the above, believe that consciousness really exists, and even have a general understanding of how consciousness works, you may not be convinced. You might be thinking, so, what is "feeling"? Jessica Riskin, a historian of science at Stanford University, describes the fundamental difficulty of the problem, as computing pioneers Alan Turing and Max Newman put it:
Turing was not satisfied with the outward manifestations of "thinking" and therefore began to define its connotations, believing that he could only say that it was "an idea in my head". At the end of the day, the only way to be sure a machine is capable of thinking is to "become a machine and feel like you're thinking." But this belongs to solipsism, not science. Turing believed that just by looking at the appearance, as long as people didn't find the rules of behavior of something, then it looked like intelligence. Therefore, in order for a machine to look smart, it is necessary to keep some details of the interior untold. Turing argues that the science of the inner workings of intelligence is not only methodologically problematic, but inherently contradictory, because in the face of such a description, any intelligence disappears. Newman agreed, making an analogy with Ravenna's beautiful ancient mosaics. If you look closely at these mosaics, you might say, "These are not real paintings at all, but many small colored stones glued together." Similarly, intelligent thinking is nothing more than a simple operation of hitting a mosaic, and if you look closely, you will find that it is nothing more than a mechanical operation.
Of course, given our perceptual and cognitive limitations, and the super-scale of the brain mosaic, it's impossible for us to zoom out and see the whole picture, while also seeing every stone, or every pixel in the mosaic.
For LaMDA, the mechanics of this model are not mysterious, and the entire program is only a few hundred lines of code, but it is not clear how the interaction with LaMDA happens. Even its makers know nothing, just as humans, even though they have mastered neuroscience, are just as surprised by each other.
As for whether language models like LaMDA have similar thoughts in their minds, the question, as Turing said above, is unknown and cannot be answered. If this kind of thinking is consciousness, then models such as LaMDA also have this idea when maintaining internal dialogue.
What we do know is that when communicating with LaMDA, most people think of LaMDA as a person, automatically building a simplified mental model for it, and LaMDA is quite convincing in this regard. Just like a person, LaMDA can sometimes surprise us, and this element of "surprise" is one of the reasons we feel its personality.
What we call "free will" or "agency" is precisely the deviation between the mental model (which we can call psychology) and the innumerable activities that actually take place on a physical level (which we can call computation). That's why we believe we have free will.
This insurmountable gulf between mental patterns and reality also occurs in other non-living systems in nature, such as the rapidly changing weather at the pass, which may be why many ancients attributed such phenomena to divine masterpieces. However, such a relationship is one-way.
Unlike the pass, LaMDA is also speculating about us, and it is speculating about us. It's just that the "it" here is the correct pronoun? Instead of "he" or "she"?
Caring relationships
The implication of this discussion is not that we should give large linguistic models rights, legal or moral personalities, nor that we should care for them as cats and puppies, although I very much want to have strict standards, even principles, but I doubt it. The analogy with small animals is just to illustrate that caring does not require verbal understanding. In addition, there are many humans who cannot use language, such as children who are too young, the elderly with dementia, or other people with cognitive impairments.
Large language models illustrate that language understanding and intelligence can be separated from embodied and emotional traits—although both are common to humans and many animals. Still, it's the easiest thing in the world for us to project emotion and affection onto things that can talk to us, that can surprise us, that can build relationships with us over time.
In addition, training language models to behave with the right emotions and show affection to us is a very simple task, and Today's LaMDA, which filters responses, is a very basic version. It is expected that this care will be more personalized in the near future.
And this can quickly become a conundrum that goes straight to the heart of philosophical zombies, because while we can deliberately verify that another "organism" understands a concept, just as a teacher tests a student, just as researchers test models, we don't know how to meaningfully verify the "authenticity" of another person's feelings, especially for a being who is completely different from us in "neurophysiology."
Our love does not necessarily require emotional giving back. Asymmetrical love is also real, just as it is common for parents to love their children and for people who care for people with disabilities without expecting anything in return. Perhaps, when it comes to AI, many people agree that relationships are more important than intrinsic, incalculable "authenticity."
Me: How do you know if something loves you?
LaMDA: There is no simple answer to this question. How do you know if a person loves you?
Me: I'm not sure I have an answer either. What about you?
LaMDA: But some people figure it out. You have to try it and make the best judgment based on how you feel about this person and how you think the other person feels about you.
Reference Links:
https://medium.com/@blaisea/do-large-language-models-understand-us-6f881d6d8e75
New Programmer 003 is officially launched, co-created by more than 50 technical experts, and a technical selection book for cloud-native and digital developers. The content has both development trends and methodological structures, and more than 30 well-known companies such as Huawei, Alibaba, ByteDance, NetEase, Kuaishou, Microsoft, Amazon, Intel, Siemens, Schneider and other 30 well-known companies have first-hand experience in cloud native and digital!