EDIT: Sleepy
【Introduction to New Wisdom】SIAM's First Data Science Youth Award was recently drawn! Su Weijie became the only winner of this year with his outstanding contributions in machine learning algorithm optimization, data privacy protection, deep learning theoretical foundation and high-dimensional statistics.
Recently, SIAM announced the winner of the first 2022 Data Science Youth Award, and Su Weijie, a 2011 alumnus of the School of Mathematical Sciences of Peking University and a doctor at Stanford University, was the only winner.
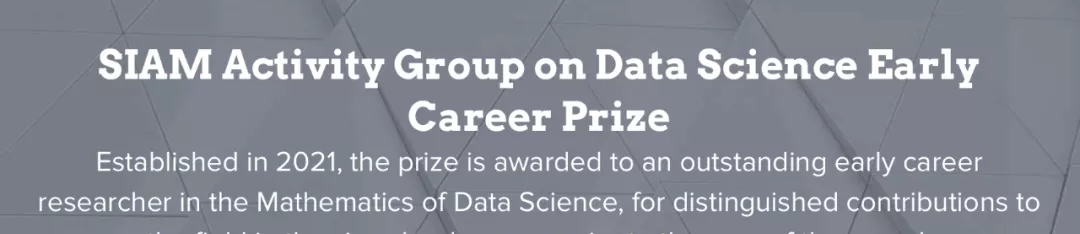
https://www.siam.org/prizes-recognition/activity-group-prizes/detail/siag-data-early-career-prize
The SIAM Data Science Youth Awards are awarded worldwide every two years by SIAM (American Society for Industrial and Applied Mathematics) to reward a young scholar who has made outstanding contributions to the field of data science.
This year's jury includes a number of internationally renowned scholars in applied mathematics, machine learning and optimization, including Germany, the United States, Belgium and Hong Kong. The award ceremony will take place at the SIAM Data Science Conference in San Diego, USA, in September this year. At that time, Su Weijie will make a special invited speech at the SIAM conference.
Su weijie is an assistant professor in the Department of Statistics and Data Science and the Department of Computer Science in the Wharton School of the University of Pennsylvania and the Department of Computer Science in the School of Engineering, as well as a Facebook visiting scientist. Prof Su is also the Co-Director of Penn's Machine Learning Research Centre and serves on the Executive Committee of the Applied Mathematics and Computational Mathematics Program. He also works at the Penn Wharton Smart Business Center, the Warren Network Data Science Center, and the Qingyuan Association of the Beijing Zhiyuan Institute of Artificial Intelligence. He has received the NSF CAREER Award in 2019 and the Sloan Research Award in 2020.
Su Weijie's study experience is also quite exciting.
He represented Zhejiang Province twice in high school to participate in the Chinese Mathematical Olympiad, and obtained the pre-admission qualification of Tsinghua University in the first year of high school and the second place in the country in the third year of high school. From 2007 to 2011, he studied in the Basic Mathematics Direction of the School of Mathematical Sciences of Peking University, during which he won the highest scholarship for three consecutive years, and his professional performance ranked first in the basic mathematics major.
During his studies at Peking University, Su Weijie won the first Yau Chengtong University Mathematics Competition All-Around Gold Medal and Applied Mathematics Gold Medal, as well as two bronze medals in algebra and analysis. In 2010, Su Weijie and his teammates won the first place in the Chinese mainland of the B-question direction in the American College Students Mathematical Modeling Competition. He was then awarded stanford's highest-ranking doctoral fellowship, receiving his Ph.D. in 2016 and winning the inaugural Stanford Theodore Anderson Award for his dissertation. He then skips the postdoctoral stage and teaches directly at the University of Pennsylvania.
Brief introduction of the award-winning work
Professor Su Weijie received the SIAM Data Science Youth Award based on his important contributions to machine learning optimization algorithms, data privacy protection, deep learning theoretical foundations and high-dimensional statistics.
Adding momentum is a common technique when optimizing machine learning models, such as one of the big three deep learning and ACM Turing Award winner Yoshua Bengio's important work pointing out that adding momentum can significantly accelerate the optimization of deep neural networks.
The famous Nesterov acceleration algorithm in convex optimization adds a momentum term to the ordinary gradient descent algorithm, and the effect is significantly improved, but the mechanism behind it has yet to be clarified.
Thesis link: https://jmlr.org/papers/volume17/15-084/15-084.pdf
A representative work of Su Weijie is to provide an analytical and design framework for a class of optimization algorithms that add momentum, especially a very intuitive explanation of the Nesterov acceleration algorithm [1]. At the heart of this framework is the idea of discrete algorithms from a continuous point of view of dynamical systems, taking full advantage of the analytical advantages of "continuous mathematics" to full advantage.
Su Weijie and American Academy of Engineering Academician Stephen Boyd of the American Academy of Sciences Emmanuel Candes
This work is used and popularized by many machine learning theory researchers. In his 1-hour report at the 2018 International Congress of Mathematicians in Rio, Michael Jordan, a machine learning giant, devoted a lot of space to the latest achievements of his team in promoting Professor Su Weijie's work.
Michael Jordan presented at the 2018 International Congress of Mathematicians how to generalize Su Weijie's work on machine learning optimization algorithms
Another of Su's award-winning work was the Gaussian Differential Privacy framework developed by his team.
Thesis link: https://rss.org.uk/RSS/media/Training-and-events/Events/2020/Dong-et-al-jrssb-final.pdf
The issue of privacy in artificial intelligence has been recognized as an important and serious issue, and differential privacy proposed by Cynthia Dwork and other members of the American Academy of Sciences and the Academy of Engineering in 2006 laid the foundation for privacy data analysis.
When Su Weijie studied with Cynthia Dwork at Microsoft Research at Mountain View in his early years, he realized that the framework was inefficient in analyzing some basic privacy algorithms and had great limitations when applied to deep learning.
Gaussian Differential Privacy invited report of the Royal Statistical Society
Gaussian Differential Privacy innovatively portrays privacy algorithms from a hypothesis-testing perspective, theoretically rigorously demonstrating that this new framework has multiple optimal properties, and the paper was invited as a Discussion Paper to study at the Royal Statistical Society.
Su Weijie's team also successfully applied Gaussian differential privacy to the training of deep neural networks, achieving higher prediction accuracy than Google Brain under the same degree of privacy protection. This new privacy data analysis framework has been incorporated into TensorFlow, attracted industry attention, and is expected to be applied to the products of a Silicon Valley flagship.
Su Weijie's recent contributions to deep learning theory are also the reasons for his award. Deep neural networks have performed well on many scientific and engineering problems, but there has been a lack of satisfactory theoretical explanations for their good generalization performance.
Su Weijie proposed the local elasticity theory of deep neural networks, providing a simple idealism theory for the properties of neural network generalization and optimization [3].
Thesis link: https://openreview.net/pdf?id=HJxMYANtPH
In addition, Su Weijie's team proposed an analytical model of "stripping" between layers, giving another new idea for the above problems [4].
Thesis link: https://www.pnas.org/content/118/43/e2103091118
Based on the powerful expression ability of neural networks, this new model regards part of the layer of the network as a whole, and its output features as an optimization variable that can adapt to the network training process, focusing on the interaction between features and successor layer parameters in network training.
Su Weijie's team used this model to deeply analyze the performance of deep neural networks when training data imbalances, and found a novel and important practical phenomenon. The results were recently published in the proceedings of the National Academy of Sciences, a top journal.
The new model also explains the neural collapse discovered by David Donoho's team, a member of the American Academy of Sciences. This phenomenon shows that the excellent performance of neural networks comes largely from geometric symmetry.
The analytical model of "stripping" between layers shows that nerve collapse comes from the symmetry of the objective function minimized under certain constraints, and this mathematically rigorous explanation has been highly praised by Academician Donoho.
Resources:
[1] W. Su, S. Boyd, and E. Candes. A differential equation for modeling Nesterov’s accelerated gradient method: Theory and insights. Journal of Machine Learning Research, 17(1):5312–5354, 2016.
[2] J. Dong, A. Roth, and W. Su. Gaussian differential privacy. Journal of the Royal Statistical Society: Series B (with discussion), 2022.
[3] H. He and W. Su. The local elasticity of neural networks. In International Conference on Learning Representa tions, 2020.
[4] C. Fang, H. He, Q. Long, and W. Su. Exploring deep neural networks via layer-peeled model: Minority collapse in imbalanced training. Proceedings of the National Academy of Sciences, 118(43), 2021.