April 2 is World Autism Day, so let's focus on this group.
Autism spectrum disorder (ASD) is a neurodevelopmental disorder characterized by social and communication disorders as well as repetitive and restrictive behaviors. ASD not only seriously affects the daily life of patients, but also places a huge burden on families and society. Unfortunately, the incidence of ASD has increased in recent years.
Previous studies have shown that timely diagnosis and appropriate intervention in ASD will reduce the severity of autism symptoms and improve cognitive, verbal, and executive function skills in young children. However, the diagnosis of ASD is largely based on behavioral observations and clinical interviews, and delays in diagnosis are inevitable due to the lack of specific biological evidence. Therefore, it is crucial to identify an early objective and accurate diagnostic approach to ASD.
Conventional MRI (cMRI) and diffusion-weighted imaging (DWI) are widely used, non-invasive testing methods. Multiple studies have shown that cMRI and ADC are important for diagnosing ASD. However, it is unclear whether these structural and functional abnormalities based on MRI can be used to distinguish between ASD and typical developmental (TD) individuals.
In recent years, with the continuous advancement of deep learning (DL) algorithms, some studies have found that MRI-based DL algorithms can be used to identify ASD and TD, and even can be used for ASD prediction. However, most of the data analysis in previous studies used fMRI, which is not practical in clinical practice. Given that cMRI and DWI have become simple and commonly used imaging sequences for assessing brain abnormalities, cMRI and ADC-based DL methods may be more valuable for ASD diagnosis.
A study published in the journal European Radiology recently constructed a DL-based ASD diagnostic model using cMRI (including axial T1, T2, FLAIR, and sagittal T1/T2 sequences) and brain ADC images from age-matching cohorts, enabling early diagnosis and treatment evaluation of patients with ASD.
A total of 151 children with ASD and 151 children in the age-matched typical developmental (TD) control group were included in this study. Data from these subjects was assigned to the training and validation datasets. In addition, 20 children with ASD and 25 TD controls were obtained, and their data were used in a separate test set. All subjects underwent cMRI and DWI tests.
In this study, a series of DL models were developed to identify ASD and TD based on cMRI and ADC data. The seven models used include five single-sequence models (SSMs), one dominant sequence model (DSM), and one full-sequence model (ASM). To enhance feature detection of the model, an attention mechanism module is also embedded.
When APPLYING FLAIR or ADC-based SSMs to validation and stand-alone test sets, the highest AUC (0.824 to 0.850) is achieved. DSM using a combination of FLAIR and ADC shows better AUC in validation (0.873) and standalone test sets (0.876). ASMs also show better diagnostic value in validation (AUC = 0.838) and standalone test sets (AUC = 0.836) compared to SSMs. Among the models with attention mechanisms, DSM achieved the highest diagnostic performance, with AUC, accuracy, sensitivity, and specificity of 0.898, 84.4%, 85.0%, and 84.0%, respectively.
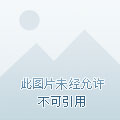
Figure Grad-CAM assisted ASD image recognition. The average heat map of the brain shows differences between ASD and TD in multiple brain regions. The fused image visually shows the anatomical location of the class-distinguished area. The values displayed by the heat map are normalized between 0 (blue) and 1 (red), with hotter colors representing areas that are more important to the classification.
The results of this study show that the differentiation between children with ASD and children with TD can be achieved based on the commonly used MRI sequences. This study also demonstrates the potential to apply a CNN model based on the ResNet-18 architecture to achieve high detection accuracy in patients with ASD and to enable early screening of ASD in a wide range of populations.
bibliography
Xiang Guo,Jiehuan Wang,Xiaoqiang Wang,et al. Diagnosing autism spectrum disorder in children using conventional MRI and apparent diffusion coefficient based deep learning algorithms. DOI:10.1007/s00330-021-08239-4
Written by | shaosai
Edit | Swagpp