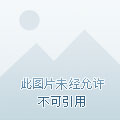
The Achilles heel of artificial intelligence
Deep learning is an artificial intelligence technology for pattern recognition, a successful technology that is fully entering the field of scientific computing. We often see it in many eye-catching news headlines, such as its ability to diagnose diseases more accurately than doctors, and its ability to prevent traffic accidents through autonomous driving. However, many deep learning systems are untrustworthy, and they can easily be fooled.
This makes AI systems like some overconfident humans, often with self-confidence far beyond their actual capabilities. Humans are still relatively good at discovering their mistakes, but many AI have no way of knowing when they made mistakes. For AI systems, sometimes it's even harder to realize they've made a mistake than to produce a correct result.
This instability is the Achilles heel of modern artificial intelligence and a paradox. This paradox can be traced back to two mathematical giants of the 20th century, Alan Turing and Kurt G del. At the beginning of the 20th century, mathematicians were trying to prove that mathematics was the ultimate language of unified science. However, Turing and Gödel discovered a paradox at the heart of mathematics: the truth or falsity of certain mathematical propositions is impossible to prove, and some computational problems cannot be solved by algorithms.
By the end of the 20th century, the mathematician Steve Smale had come up with a list of 18 unsolved mathematical problems at the time, the last of which explored the limits of intelligence between humans and machines. This problem has not been solved so far, but it brings turing and Gödel's first paradox into the world of artificial intelligence: mathematics has inherent fundamental limits, and similarly, artificial intelligence algorithms have problems that cannot be solved.
The inherent limits of artificial intelligence
A new study suggests that ai-powered people have inherent limits that can be attributed to this century-long mathematical paradox. By extending the method proposed by Gödel and Turing, the researchers showed the limits of the algorithms that compute neural networks exist. They propose a classification theory that describes a situation in which, under certain conditions, neural networks can be trained to provide trusted AI systems.
The findings were published in the recent Proceedings of the National Academy of Sciences. The new study points out that there are problems with stable, precise neural networks, and that no algorithm can produce such networks. Only in specific cases can the algorithm compute a stable, precise neural network.
Neural networks are the most advanced tools in the field of artificial intelligence, and they are called "neural networks" because they are a rough simulation of the connections between neurons in the brain. In the new study, the researchers say that while in some cases, good neural networks can exist, we can't create an inherently reliable neural network because of this paradox. In other words, no matter how accurate the data we use to build a neural network, we will never get the perfect information we need to build that neural network.
At the same time, no matter how much data is trained, it is impossible to calculate a good existing neural network. No matter how much data an algorithm can access, it won't generate the network it needs. This is similar to Turing's view: regardless of computing power and runtime, there are unsolvable computational problems.
Researchers say not all AI is inherently flawed. In some cases, AI makes mistakes without problems at all, but it needs to be honest about facing these problems. However, this is not what we see in many systems.
Understand the basics of artificial intelligence
When we try something and find that it doesn't work, we may add something else in the hope that it will work, but if we still can't get what we want when we add it to a certain level, we choose to try a different approach. It is important to understand that different approaches have their own limits. Now, AI is at a stage where its actual success is far ahead of its theory and understanding of it, so there is an urgent need to be able to understand the computational underpinnings of AI to bridge this gap.
When 20th-century mathematicians discovered different paradoxes, they didn't stop studying mathematics. They must find new paths because they understand the limits. Correspondingly, in the field of artificial intelligence, this may mean changing paths or developing new paths to build systems that can solve problems in a reliable and transparent way, while understanding their limits.
The next stage for researchers is to combine approximation theory, numerical analysis, and computational foundations to determine which neural networks can be computed algorithmically and which can become stable and trustworthy. Just as Gödel and Turing have proposed a wealth of fundamental theories about the paradoxes of mathematical and computer limits, perhaps similar basic theories may blossom in artificial intelligence.
#创作团队:
Author: Light rain
Typography: Wenwen
#参考来源:
https://www.cam.ac.uk/research/news/mathematical-paradox-demonstrates-the-limits-of-ai
#图片来源:
Cover image: julientromeur / Pixabay
Top image: Chenspec/Pixabay