Transient Electromagnetics (TEM) is a geophysical method based on the laws of electromagnetic induction. Traditional TEM observation devices are all laid on the surface, and the work efficiency is relatively low, especially in deserts, Gobi, mountains, wetlands, and areas with dense water networks. To this end, the detection equipment was mounted on the flight platform, resulting in the Airborne TEM (ATEM). However, the useful signal in the ATEM data is relatively weak and the noise conditions are harsher due to the observation location being farther from the ground and the observation process always in motion, as opposed to static observations on the ground. At present, the general processing strategy is to establish a processing process containing multiple steps, and deal with a certain kind of noise step by step, the whole process is very demanding on personnel, and it is difficult to evaluate whether the final processing result still contains "residual noise". Even so, as the downstream end of data processing, the inversion method does not have a specific mechanism to determine whether the remaining noise is still contained in the input data. Therefore, once there is a defect in the data processing process, it will inevitably have a serious impact on the reliability of the resistivity model estimated by the inversion. The reason for this problem is that signal-to-noise separation (denoising) and signal-mode mapping (inversion) are isolated from each other within the existing framework. If the barrier between the two can be broken, it is expected to improve the reliability of the model estimate.
The transient electromagnetic research team of the Key Laboratory of Mineral Resources Research, Institute of Geology and Geophysics, Chinese Academy of Sciences, analyzed the internal logic between signal-to-noise separation and letter-mode mapping, and proposed a mathematical hypothesis, that is, the model and signal are high-dimensional structures with different dimensions of the same low-dimensional manifold. This mathematical hypothesis becomes the theoretical starting point for the barrier between communication-noise separation and signal-mode mapping, on the basis of which a deep learning method (Figure 1) is used to simultaneously optimize the networks used for signal-to-noise separation and resistivity model estimation, so that the entire neural network can simultaneously perform denoising and inversion in the traditional sense, so that a more objective and reliable geodetic resistivity model estimation result can be obtained from the noise-containing data in one stop.
Figure 1 A network architecture based on a manifold hypothesis design in which dn, C, d, and m represent noisy input data, intermediate results, noise-free data, and theoretical models, respectively. The network structure consists of two paths, and the node C (intermediate result) shared by the two paths represents the common low-dimensional manifold of the model and data
At present, there are various network forms that can realize the above architecture design, and the research team uses stacked Auto-Encoder (SAE) neural networks to carry out follow-up research. The SAE consists of three subnets, a denoising autoencoder for sub-network 1, a sub-network 2 for an autoencoder, and a sub-network 3 for a fully connected network. During the training process, the three sub-networks are trained one by one, and after convergence is reached, the entire SAE network is trained (Figure 2).
After completing the network training, the network processing effect is first tested by using simulation data (forward response data + measured ATEM noise data) (Figure 3). The comparison shows that the blue curve has a higher sensitivity to model resistivity discontinuous interface pickup, and each discontinuous interface is accurately picked up, which is the advantage of this method; in contrast, although the traditional method has insufficient resolution, especially for small gradient discontinuous interfaces (Figure 3b), the entire curve is smoother due to the smooth and stable function. To do this, the research team added a smoothing processor after the subnet 3, which was able to obtain a smoother output result.
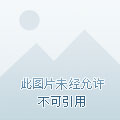
Figure 2 Basic design of SAE neural network. Subnet 1 is a DAE that enables primary processing of noisy input data; subnet 2 is an AE that further extracts the deep features of the input signal; and subnet 3 converts deep features into resistivity sequences
Figure 3 Results of typical model experiments, where the black line is the design model and the blue curve is the output result of the SAE network, which is the output result of the traditional data processing-inversion process. (a) Three-tier model;
The above network is used to process the same section of the line measurement data of Inner Mongolia Taohemu Sumu (Figure 4), which can be seen through comparison, and the two methods obtain basically the same resistivity distribution state, due to the increase of the smoothing period after the sub-network 3, the overall smoothness of the results of the new method has also been significantly improved. On the other hand, the new method has stronger resolution ability for depicting the low-resistance layer near the surface and its spatial spread form, as well as the edge of the underground high-resistance layer, which is the same as the conclusion obtained by the simulation data test, reflecting the advantages of the new method in terms of resolution ability.
Figure 4 Comparison of measured data processing results. (a) Results of traditional methods;
Editor: Fu Shixu
Proofreader: Qin Huaqing Jiang Shumin