More than five years have passed since the AI chip startup boom around 2016, from team formation, to chip design, to product landing, AI chip companies have reached the time to hand over an answer sheet.
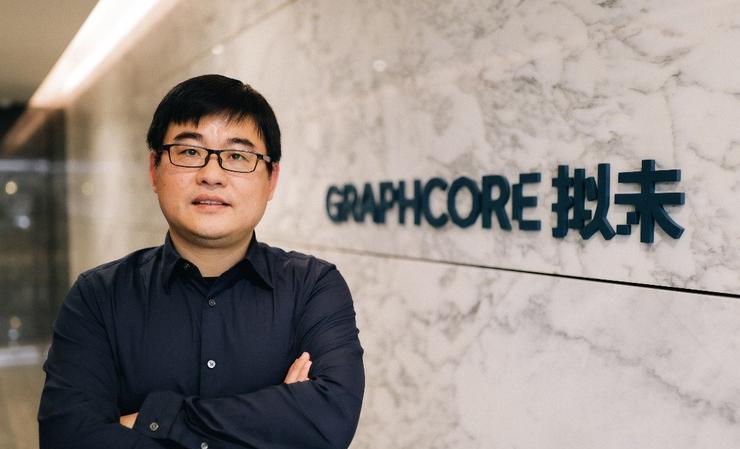
Tao Lu, President and Global Chief Revenue Officer of Graphcore Greater China
Lu Tao, president and global chief revenue officer of Graphcore Greater China, told Leifeng, "The competition for AI chip companies will be more intense in 2022, because different companies have chosen different strategies and paths, and this year can be seen more clearly." ”
In fact, from 2020 onwards, most AI chip companies have opened the landing of chips, but due to the obvious difference between AI chips and traditional chips, the cognitive differences between chip providers and users, coupled with the impact of the epidemic, and the lack of cores in the global spread, the landing and large-scale use of AI chips are facing many challenges.
In 2021, the industry is paying more attention to the deployment of AI, including a focus on machine learning frameworks, supported models, and cluster management and scheduling management.
What are the three secrets to becoming the leader of AI chips?
Seven steps in front of AI chip companies
The AI boom has spawned a large number of chip startups, and startups want to successfully land a new type of AI chip to challenge the current giants, which can be compared to climbing Mount Everest.
Lu Tao believes that if today's giants are on Mount Everest, if they want to reach the height of giants, they need to climb seven steps.
The first step is to form a team, the second step is to preach the concept, the third step is to design a chip, the fourth step is to have a chip and can send samples to customers for testing, the fifth step is to have a product to land, the sixth step is to have a product, there are many landings, and the seventh step is to have a large market share. Lu Tao believes that Graphcore is in the fifth to sixth steps.
Companies at this stage show that they have solved many technical challenges. AI computing is significantly different from traditional CPUs, and in the past 5-10 years, CPU applications are very clear, such as Web services, database services, storage services, etc. However, there are many innovations and research in the application of AI as a whole, and the whole is highly dynamic.
In such a case, the landing of AI chips is more difficult, the steps can be roughly divided into three, first, to use the performance advantages of AI chips to attract customers, and then customers will consider the difficulty and time of software porting, after verification, in order to achieve large-scale deployment.
The process can be said to be long and challenging. One of the big challenges is the difference in perception between AI chip providers and users. "From our perspective, while this difference in perception has improved, challenges persist." Lu Tao said, "For example, many users may think that our IPU performance is good and want to migrate to the IPU without modifying the code, which requires us to do a lot of work in software and ecology." ”
The construction of AI software and ecology requires a relatively complete understanding of a specific application field to achieve end-to-end cooperation of the entire business, not only AI, but also the cooperation between AI chips and other devices and systems, operation and maintenance management, etc.
Lu Tao pointed out, "As a provider of computing platforms, to allow end users to use our platform well, ecology is very important, and ecological construction can reduce the threshold for users to use." At the same time, vertical cases are also very important, through the 'model', can significantly accelerate the application in a certain industry. Of course, strengthening cooperation with AI platform vendors is also key. There are two kinds of users in the FIELD of AI, one is ai developers, who can do corresponding development with cases, and the other is AI platform enterprises, they can package AI technology for other people to use. ”
For developers or researchers with computer backgrounds, they can program based on TensorFlow, PyTorch, Baidu Flying Propeller, etc., if they do not have this ability, they need to use more advanced frameworks, such as Digging Face, to lower the development threshold and simplify development. The development difficulty of the two is significantly different, if you implement a business with PyTorch, it may take two hundred lines of code, but with Hug Face, it may take 50 lines of code to complete.
Three keys to becoming a leader in AI chips
It is not difficult to find that in order to achieve large-scale landing of AI chips, reducing the cognitive difference between AI chip providers and users is the key, and the key to this is the construction of software and ecology.
But to become a leader in the field of AI chips, Lu Tao believes that there are three keys: prejudgment + take a little risk + a little luck.
"When Graphcore was founded in 2016, it could only catch up. For new areas, if you can predict in advance, you may have a leading position in the field. Lu Tao explained specifically.
In 2016, the traditional machine vision model ResNet already existed, and at this time Graphcore needed to catch up, and as a catcher, it needed to do some pre-judgment to find opportunities. In 2020, Graphcore focused on Transformer technology, which was originally the underlying technology for natural language processing, but there was a trend in the industry to use Transformer for computational vision, and Graphcore took the lead in supporting it.
By the second half of 2021, some Transformer-based visual models, such as ViT, have become popular. Due to advance prediction, Graphcore has good support for new Transformer-based vision models as well as GNNs, and many innovative and cutting-edge models support better than GPUs.
It is with innovative IPU hardware and the constant improvement of the Poplar software ecosystem that Graphcore has many application cases in 2021. In the second half of 2021, Agile Digital will use IPU for meteorological forecasting, precision irrigation, disaster prevention and mitigation. Deep Potential Technology completed the migration of molecular dynamics simulation software DeepPMD-kit to IPU hardware, and explored scenarios such as scientific computing, drug design, material design and new energy sources based on molecular dynamics simulation.
In the financial and insurance sector, Oxford-Insman uses IPUs for stock price forecasting; Tractable has partnered with Graphcore to accelerate accident and disaster recovery. In terms of telecommunications, Graphcore partnered with Korea Telecom to release the IPU Cloud. In the field of sustainable urban environment, Shengzhe Technology applies urban-related sustainable development based on IPU. In the field of medical and life sciences, Graphcore has partnered with Stanford University School of Medicine to conduct some research and exploration using IPUs to take "medical + privacy computing" as the core direction.
He also revealed that in 2022, Graphcore will have some IPU product releases with domestic public cloud vendors, and there will also be new hardware product releases. Graphcore will also further deepen cooperation in some related AI application areas, such as AI-assisted scientific research, autonomous driving, etc. are their more important directions in AI applications or vertical fields in 2022.
It is worth mentioning that the hardware decoupling of Graphcore's IPU and CPU will help IPU better expand the market. For example, in BERT training, Graphcore uses a server with 2 CPUs and 64 IPUs, with a ratio of 1:32, and the ratio in the computer vision model is basically 1:8. But if it is an Nvidia or Intel system, no matter what the scene and model, there will be a fixed CPU and GPU ratio, such as 1:4 or 1:2.
Write at the end
The competition of AI chips will eventually evolve into ecological and commercial competition. In the early stage of AI chips, although there are many participants, they are all in the stage of team formation and product development, and there is no real competitive relationship between AI chip companies. With the release of AI chips and the advancement of landing, the competition between AI chip companies has really begun.
When more and more fields and customers begin to pay attention to the scale application and landing of AI, it will naturally intensify the competition between AI chip companies, at this time, it is no longer a simple competition for hardware performance, software and ecology can impress end users.
In 2022, we will see the increasingly fierce competition among AI chip companies, and we will slowly see the real strength of AI chip companies. Leifeng Network