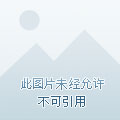
Reporting by XinZhiyuan
EDIT: Sleepy
The success of reinforcement learning in the field of Go and games has made it one of the most concerned areas of machine learning and artificial intelligence, attracting many leading companies in the technology industry such as Google, Deepmind, openAI to compete, and being identified by Deepmind as a key technology to "general artificial intelligence".
Although reinforcement learning has demonstrated the ability to act autonomously and make decisions beyond top humans in the game world, there is still a long way to go to make reinforcement learning go beyond the game world and implement it into real business scenarios.
With the profound accumulation in the field of reinforcement learning, Nanqi Xian ce has not only achieved breakthrough research results and developed unique environmental model learning technology, but also opened up the closed loop of algorithm, engineering, implementation and service landing practice, and took the lead in opening up a path in the thorny bush of reinforcement learning landing.
Nanqi Xian Ce condensed the reinforcement learning landing technology and experience in the core tool "Xianqi", and recently released the official version of this "intelligent decision-making industrial software".
At the same time, in order to better promote the development of global reinforcement learning technology and the cultivation of technical talents in related fields, Nanqi Xian Ce and Jiangsu Artificial Intelligence Society jointly launched the "AI Decision-making Reinforcement Learning Landing Challenge".
The challenge will open the registration channel on December 25, welcome the industry elite to show their skills in the competition, and fully demonstrate the advanced strength of AI decision-making technology!
AlphaGo's brilliance and dilemma
On March 9, 2016, AlphaGo, a Go AI system developed by Deepmind, won the first set in a game against Go world champion Li Shiqian. Five days later, AlphaGo defeated Li Shiqian 4:1, which was also the first time that AI had defeated the top professional players in Go.
This victory is an important milestone in the development of artificial intelligence. The development of global artificial intelligence has entered a new round of rising, and at the same time, an unfamiliar technical term "reinforcement learning" has also begun to receive widespread attention.
In fact, reinforcement learning is not a new thing, and its representative technology "timing difference" method was born in 1984, 37 years ago.
In the more than 30 years before AlphaGo made history, reinforcement learning was less well known than the technical applications in other areas of artificial intelligence. The low profile of reinforcement learning is not intentional, but there are internal reasons.
"Reinforcement learning" and "supervised learning" are juxtaposed and are the main subfields of machine learning. The goal of supervised learning is to solve prediction problems, learning from labeled data, for example, speech and text recognition, face recognition, etc. are such technologies; while reinforcement learning solves decision-making problems, just like creatures in nature, they need to grope in the environment, trial and error, according to the feedback of the environment, change their life habits, so as to better "survive".
Reinforcement learning is a self-trial-and-error learning method that reduces the dependence on human beings, which is therefore considered by Deepmind to be a key technology to "general artificial intelligence". In the virtual environment with completely clear rules of the game, with the help of powerful computing power, the acceleration effect of one day in the real world and decades in the game world can be achieved, so reinforcement learning can obtain decision-making ability beyond humans in a short period of time through massive trial and error learning.
In the case of AlphaGo, for example, it has played more than 30 million games of self-play in just a few months through reinforcement learning in a Go environment, and if you calculate the average amateur chess player per hour, it will take at least 4,000 years for humans to acquire such decision-making ability!
It is conceivable that for practical business problems, if people can also portray clear and complete rules of the game, then reinforcement learning can easily find out the optimal business decisions, become a powerful helper for people, liberate human tedious labor in small decision-making, and instead give full play to human advantages in top-level design - imagination and creativity.
But in fact, most of the practical problems we face lack clear and complete rules, which is not the ideal "game environment". What's even more cruel is that if reinforcement learning is allowed to train trial and error in the real world, it will bring huge costs to people, and even life is dangerous!
Therefore, leaving the virtual game world, reinforcement learning is no longer intelligent, not only the learning speed is extremely slow, but also because of the large number of wrong behaviors that will be tried in the learning process, causing irreparable losses.
Under the aura of AlphaGo, today's reinforcement learning has become the highlight direction in the field of artificial intelligence. However, the progress of academia does not match the expectations of the industry.
If there's an algorithmic innovation today that can reduce the hundreds of millions of exploratory interactions required for reinforcement learning by two orders of magnitude, that would be big news in academia. However, millions of explorations in industry are still far from being widely used.
Environmental Model Learning – The Maverick Choice
Back in May 2016, two months after AlphaGo's victory over Li Shiqian, Professor Yu Yang of Nanjing University's LAMDA group received a business consultation from Alibaba's team in charge of "Taobao Search": When applying reinforcement learning to search sorting tasks, can you learn only from offline data?
Obviously, when trying to apply reinforcement learning technology, the technical team encountered the challenge of lacking a "game environment", and there are no clear rules to describe the user's behavior on the Taobao search sorting task, so it is difficult to construct a "game environment" for reinforcement learning training.
If the "game environment" can be restored from offline data, reinforcement learning can play an advantage, and the problem can be solved.
In April 2017, Professor Yu Yang submitted an application for the "Virtual Taobao" cooperation project, trying to learn from historical data an environment with virtual users, with which "zero cost" training reinforcement learning can be achieved. After the project application was submitted, it was quickly challenged by the review experts, "simulating the environment of e-commerce is unbelievably challenging", an email that remains in Professor Yu Yang's inbox.
"The skepticism was very reasonable, in fact, there was no technology that could learn the environment well." Professor Yu Yang said. Even in today's mainstream perception of the international reinforcement learning academic field, environmental models are still considered extremely difficult, and based on this recognition, the direction of mainstream technology is to use environmental models without or less.
"But learning in the environment is extremely critical." Although questioned, Professor Yu Yang did not abandon technical research in this direction.
In fact, in the LAMDA Laboratory of Nanjing University, Professor Zhou Zhihua has long pointed out that adapting to the open dynamic environment is an important direction for the future of machine learning, and was supported by the key projects of the National Natural Science Foundation of China in 2014, and the relevant results were taken as one of the representative work to lead to the future of stable artificial intelligence in the 2016 International Artificial Intelligence Conference AAAI Chair Report.
Therefore, learning the environment model is the only way in Professor Yu Yang's view, but the question of how to learn the environment well has always been in his mind.
In January 2018, after various attempts and efforts, a preliminary technical solution saw effective results for the first time in the test of Taobao scenarios. This result finally gave Professor Yu Yang some confidence.
But more questions arise, "Is there any versatility to this technology?" Can it be supported by mathematical theory? Is it valid on other issues as well, and what is the scope of the effect?" With these questions in mind, Professor Yu Yang and his students have worked with more companies to verify the effectiveness of the technical solution in a variety of different scenarios, and the technical solution is constantly being upgraded and improved.
At the 2020 International Top International Conference on Artificial Intelligence, NeurIPS, Professor Yu Yang's team published the latest theoretical achievements of the technology in environmental learning, which strictly proved the feasibility of the technology in theory and also gave a shot in the arm for the environmental learning technology.
"Looking back now, I'm glad I insisted on doing environmental learning." The environmental model not only achieves "zero-cost" training reinforcement learning, but also has many advantages that are difficult to replace by other technical approaches, such as predicting the effect of decision-making, flexibly adjusting decision-making goals, and imposing overall constraints.
The mission of Nanqi Xian Ce
Technology has worked out, but it is still quite a step from truly forming productivity.
In the process of actual scenario verification, it is found that the application threshold of environmental model learning technology is very high, and there are large differences from business understanding to technology application. "It would be a shame if technology can't be transformed into productivity, if it can't benefit society, and it just stays on paper."
In June 2019, with the joint support of the Artificial Intelligence Innovation Research Institute of Nanjing University and investors, Professor Yu Yang and Qin Rongjun began to form the Nanqi Xian Ce team to promote the in-depth application and popularization of technology. While applying environmental learning technology to more scenarios, Nanqi Xian ce brings back the experience and challenges of scene landing, and constantly improves the technology.
After continuous iteration, Nanqi Xian Ce has precipitated the core technology and application experience to create a universal intelligent decision-making tool based on environmental learning, "Xianqi". On December 24, 2021, the official version of Nanqi Xian Ce "Xian Qi" was released.
"Xianqi": intelligent decision-making industrial software based on environmental learning
Before the release of the official version of "Xianqi", Nanqi Xian Ce had actually released a number of open source algorithm libraries. Qin Rongjun introduced that this includes: offline reinforcement learning algorithm library (https://agit.ai/Polixir/OfflineRL), offline reinforcement learning dataset (https://agit.ai/Polixir/OfflineData), offline strategy evaluation algorithm library (https://agit.ai/Polixir/d3pe), As well as the gradient-free optimization algorithm library (https://agit.ai/Polixir/ZOOpt), "Xianqi" is based on these open source libraries.
https://revive.cn is a SaaS platform system that also provides SDK software. For developers familiar with reinforcement learning, the Xianqi SDK provides the most flexible way to use it. The "Xianqi" SaaS system provides services for data access, decision flow diagram design, environment learning, strategy training, model deployment, and special attention to team collaboration to facilitate developer information sharing.
Through cooperation with dozens of companies in various industries and fields, "Xianqi" has realized the application of cross-industry multi-business scenarios in energy, manufacturing, logistics and marketing, and has been highly recognized by the industry and enterprise side.
Although the business processes of these industries are complex and there are huge differences between the industry and the industry, Xianqi can do the key point and land steadily every time, and even often break the cognition of the enterprise, bringing unexpected good results, "Enterprises often say ' I really didn't expect that the original in each scenario can continue to do decision-making optimization, Xianqi let us see new opportunities", Qin Rongjun introduced.
What is even more rare is that "Xianqi" provides auxiliary decision-making tools for industrial business scenarios, which are decoupled from the actual decision-making ring of the business, and can be flexibly deployed by enterprises according to their own situation. At the same time, "Xianqi" directly learns based on the historical data of business scenarios, without the help of other commercial software, the deployment cycle is short, and it also brings a very low threshold for enterprises to use.
For developers interested in learning about "Xianqi", the reinforcement learning landing challenge jointly organized by Nanqi Xian ce and Jiangsu Artificial Intelligence Society released a competition question for marketing operation business, in which the baseline method is obtained through a simple call to "Xianqi", and the contestant also provides the source code of the baseline method to provide a starting point for the contestants.
Reinforcement Learning Landing Challenge
The "AI Decision-making Reinforcement Learning Landing Challenge" was released simultaneously with "Xianqi".
The challenge was jointly initiated by Nanqi Xian Ce and jiangsu Artificial Intelligence Society to promote the application of reinforcement learning in real-world scenarios. Welcome interested friends to participate!