Recently, researchers at the University of Science and Technology of China used machine learning methods to show the similarity and diversity of global earthquake rupture processes in a panoramic manner, which has enlightening significance for earthquake early warning.
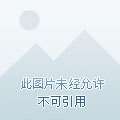
Image courtesy of India's Asia News Agency (IANS)
Earthquakes are one of the important natural disasters facing human society. In the past 20 years, the world's largest earthquakes have caused nearly 1 million casualties and countless economic losses. Earthquake rupture processes are diverse, and objectively measuring their similarities and differences will help people understand the physical processes of earthquakes and make early predictions of earthquake magnitude.
But previously, studies of the average rupture process of multiple earthquakes overlays were unable to measure the range of differences in global earthquakes. At the same time, statistical studies based on certain rupture characteristics cannot make a systematic comparison of the entire rupture process.
This time, Li Zefeng, a researcher at the University of Science and Technology of China, used machine learning methods to summarize the time function characteristics of more than 3,000 earthquakes above magnitude 5.5 around the world, showing the similarity and diversity of global earthquake rupture processes in a panoramic manner, deepening people's understanding of earthquake energy release patterns, and having enlightening significance for early earthquake early warning. The results were published in geophysical research letters, a journal of the American Geophysical Society (AGU).
Image courtesy of Geophysical Research Letters, a journal of the American Geophysical Society (AGU)
The researchers used the Variational Autoencoder in deep learning to compress and reconstruct the source time function of more than 3,000 medium and large earthquakes around the world in two dimensions, realizing a universal model of the global source time function, and showing the global seismic moment release pattern and quantity distribution in a panoramic manner.
The model shows that medium and large earthquakes are mainly simple and uniform ruptures, and there are fewer complex and irregular ruptures. The model also reveals the distribution of two types of special types of earthquakes, namely escape patterns in which energy release is concentrated in the late rupture period, and complex earthquakes that are released in multiple parts. The study found that the energy release mode of large earthquakes has a weak magnitude dependence, which provides useful enlightenment for the predictability of the final magnitude in the early warning of earthquakes.
a is the distribution of the global seismic source time function in the implicit space of the variational autoencoder, and b is the reconstructed global seismic rupture pattern manifold(manifold), picture from the University of Science and Technology of China
The above research is based on the development of the source time function clustering method jointly studied by Li Zefeng's team and Harvard University in 2021, and is also one of the series of research results that the team has committed to applying artificial intelligence to scientific discovery (AI for Science) in recent years.