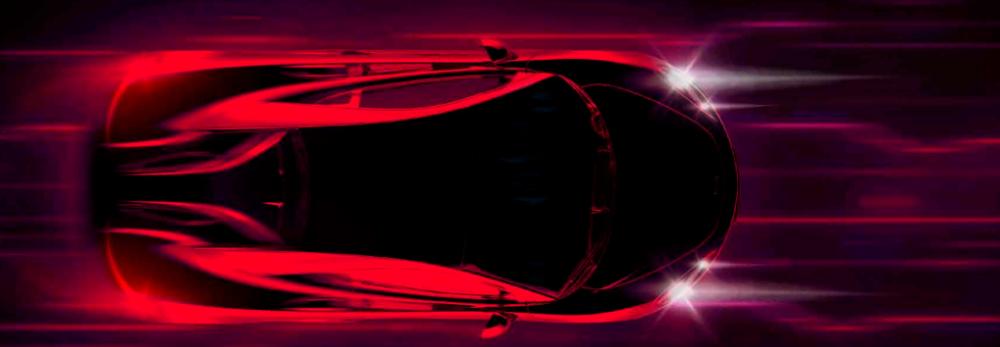
This article is excerpted from the white paper "Confidently Moving Toward Fully Autonomous Driving"
Competition has begun. Fully autonomous vehicles (AV) are rapidly entering our lives. In addition to improving the overall efficiency of the transportation system, ensuring the safety of drivers and occupants is the most compelling advantage of autonomous vehicles. The latest figures show that about 1.3 million people die in road traffic accidents worldwide each year. According to the National Highway Traffic Safety Administration (NHTSA), 94 percent of serious car accidents are caused by human error.
Figure 1 According to NHTSA, 94% of road accidents are caused by human error
Improve the level of autonomous driving in cars
What if we could improve safety and reduce the risk of road traffic accidents? What if we could avoid driver error? The report shows that self-driving cars can reduce the number of road traffic fatalities by as much as 90%. In addition to reducing the number of accident deaths, self-driving cars can also avoid billions of dollars of car accident-related losses.
Advanced Driver Assistance Systems (ADAS) in production vehicles have reached the level of L2+ to L3 autonomous driving as defined by SAE International, requiring driver control of the vehicle in most traffic conditions (Figure 2). OEMs and industry experts believe that achieving L4 and L5 autonomous driving, where L5 means that the vehicle does not require any human interaction, can make road traffic safer. However, there is still a unique set of challenges to achieving this goal, requiring significant technological advancements.
Figure 2. SaE-defined autonomous driving classes (Source: SAE International)
To achieve a higher level of autonomous driving, the automotive industry needs to address all aspects of technology, society, law and regulation. While many of these issues are difficult to control, the automotive industry and OEMs work together to push the boundaries of technology. They were able to create smaller, more rugged, and more economical radars, lidar, cameras, and other sensors, while improving the detection and identification software for those sensors. To improve the training of these algorithms, the industry needs to overcome two obstacles.
Bridge the gap between road testing and software simulation testing
Today, OEMs use simulation environments with software-in-the-loop systems to test sensors and control modules. Although software simulation is useful, it cannot fully reproduce the reality of the situation and the imperfect sensor response that can occur. Fully autonomous vehicles must know how to respond to such situations.
By road testing complete systems integrated in prototypes or legally on-the-road vehicles, OEMs are able to validate the performance of the final product before bringing them to market. Road testing is an integral part of the development process, but given the cost of testing, the time it takes to test and the repeatability of testing, it is an unrealistic idea to rely entirely on road testing. If taken in this way, vehicles would need to be tested over hundreds of years to achieve sufficient reliability to safely drive on urban and rural roads without fail.
The ADAS/AV algorithm cannot be trained under real-world conditions
In-vehicle radar testing is important for training autonomous driving algorithms. These algorithms use data from on-board radar sensors to make decisions that indicate how a vehicle should respond when it encounters a particular driving condition. If algorithms are not properly trained, they can make unexpected decisions that endanger the safety of drivers and occupants or pedestrians. In order to test enough scenarios to verify AV functionality, OEMs need to move from a few targets to real scenes, get out of the boring theoretical world, and enter practical applications.
Drivers have a lot of decisions to make. Becoming a good driver often requires time and experience. Taking autonomous driving to the next level requires complex systems that are capable of outpacing the best human drivers. The combination of sensors, sophisticated algorithms and powerful processors is a key factor in enabling autonomous driving. While sensors perceive their surroundings, the processors and algorithms behind them must, in any case, make the right decisions at a much faster rate than human drivers – such as obeying road traffic rules.
We must ensure that the new ADAS functions are safe and reliable. Premature road testing with immature systems can be risky. Automotive OEMs need to be able to simulate real-world scenarios and verify the sensors, electronic control unit codes, artificial intelligence logic, and other parts that are actually used. Testing more scenarios early gives OEMs a clear idea of when development can be completed and when ADAS features can be released with confidence.
Gaps in self-driving car testing and validation
Today's test systems do not effectively solve certain challenges. They only test a certain number of targets, cannot simulate objects in close proximity, and are difficult to distinguish objects.
Limited number of targets and field of view
Some systems use multiple radar target simulators (RTS), each of which presents multiple point targets to the radar sensor and simulates horizontal and vertical positions by mechanically moving the antenna. This mechanical automation slows down the overall test speed. In addition, a single movement of the antenna can cause a change in the angle of arrival (AoA) that, if not recalculated or recalibrated, can lead to errors and loss of accuracy in the render target.
Another option is to use multiple radio frequency (RF) front ends for each RTS, with one object using a delay line. If a scene requires multiple objects, you should increase the number of RF front ends in your test setup and then switch between them as needed. This means that it can generate objects anywhere in the scene, but not at the same time simulate targets on opposite sides of the scene.
Moving the RTS or switching between multiple RF front ends creates gaps and missing objects in the scene, labeled X in Figure 3. Testing a radar unit with only a limited number of objects does not provide a complete view of the driving scene or reveal the complexity of real-world conditions, especially in urban areas with various intersections and turning scenarios involving pedestrians, cyclists, or electric vehicle riders.
Figure 3:Example of a scene with a limited number of RTS or radar front ends
Objects that are less than 4 meters away cannot be generated
Current radar target simulator solutions cannot generate objects that are within 4 meters (and in some cases even farther). This creates a blind spot in front of the car bumper. Test cases such as the New Vehicle Evaluation Program are required to simulate objects at a distance very close to the radar unit. Take, for example, an automatic emergency braking system. Obstacles on the road need to be very close. Target simulation solutions currently on the market can only simulate objects that are 4 meters or more away (Figure 4).
Figure 4.If there is a large blind spot in front of the simulated vehicle, the emergency braking scenario cannot be tested
Lower resolution between objects
Earlier radar technology treated the object as a point target, which meant that the radar did not consider the spatial properties of the detected object, but instead concentrated all the energy reflected by the object into a single point. The concept of radar cross-sectional area came into being. It converts the density of power hit to the target into reflected power and combines the spatial dimensions, shape, and reflectivity into a single number. However, this approach only works if the target is viewed as a point. In modern automotive radar, where targets are often relatively close to each other, radar units must utilize high angular resolution to perceive the spatial characteristics of objects.
Target simulators currently on the market cater to this obsolete radar sensor need. They are designed to treat an object as a radar signal, resulting in a lack of scene detail.
Key technological advances to meet challenges
In order to realize the ADAS capabilities required for the vision of fully autonomous driving, reliable radar sensors and algorithms need to be used. Performing full-scenario simulations in the lab is critical to developing these radar sensors and algorithms. Keysight's full-scenario simulator uses hundreds of tiny RF front ends to form an expandable simulation screen that can render up to 512 objects up to 1.5 meters away.
The successful delivery of this solution will also require breakthroughs in the following areas:
Single miniature RF front end (Figure 5a)
Eight RF front ends integrated on a single board (Figure 5b)
64 circuit boards are arranged in a semicircular array to form a simulation screen (Figure 6).
In addition to technological advances in RF hardware, software has innovated on the same scale to avoid device under test (DUT) detecting fake objects within 1 m and connecting 3D imaging software that renders these images to the screen.
Figure 6: An animation of combining 64 circuit boards into an RF front-end screen
Create more objects for the scene
Keysight's radar scene simulator uses patented technology to simulate the entire traffic scene instead of object detection through target simulation (Figure 7).
Figure 7:Comparison of target simulation and scene simulation
With an open field of view and extremely small object distances, the solution can simulate complex scenes as well as high-resolution objects in the scene. It does this with a compact RF front end that is densely packed.
In terms of design principle, these miniaturized "pixels" cannot be detected by radar sensors, and are completely activated by 3D simulation software, which can replace mechanical motion. Each pixel in the array simulates the distance and echo intensity of one object (Figure 8).
Multiple pixels can represent an object based on its size and distance from the device under test (Figure 9). If multiple objects are far apart, you can complete the simulation by shortening the distance.
Open continuous field of view
Keysight's Radar Scene Simulator not only helps radar sensors discover more targets in a wider continuous field of view, but also enables simulation of both short-range and long-range targets. This avoids dead zones left over from the radar field of view and improves algorithm training to efficiently detect and resolve multiple objects in dense, complex scenes. Therefore, AV decisions are made based on the overall situation and not just what the test equipment allows.
Keysight's technology covers the entire field of view of the sensor, allowing for extended test coverage and more comprehensive and complex test scenarios. The detection software for radar sensors is run using objects up to 512 pixels and a continuous field of view ± 70° azimuth/± 15° elevation. The 512 RF front ends remain static in space for accurate and repeatable AoA verification.
The minimum distance is closer
Realistic traffic scenarios require the simulation of objects very close to the radar unit. For example, at traffic lights no more than 2 m apart from vehicles, bicycles may enter the lane and pedestrians may suddenly cross the road. Passing this test is critical to verifying the safety functions of ADAS/AV. Keysight's Radar Scene Simulator has a simulation range of 1.5 m to 300 m and speeds up to 400 kph.
Provides a higher resolution for each object
Object separation represents the ability to distinguish between different obstacles on the road, which is another test focus to make autonomous driving technology smoother and faster to enter levels 4 and 5. For example, when a car is driving on a highway, radar detection algorithms need to distinguish between guardrails and pedestrians.
Figure 12: Multiple points per object to add practical information to the scene
Keysight solves this problem with a point cloud concept, bringing higher resolution to each object. This approach provides more detail to the scene and helps automotive OEMs confidently test algorithms to distinguish between two very close objects. While a traditional RTS returns a reflection independent of distance, the radar scene simulator increases the number of reflections, also known as dynamic resolution, as the vehicle approaches. This means that the number of objects changes with their distance.
Test more scenarios faster
The Radar Scene Simulator enables OEMs to easily detect gaps or misconduct in ADAS software through adaptive rendering based on dynamic resolution, and treats real-world scenes provided by nearby objects as multiple separate targets. OEMs are thus able to build complex real-world scenes in the lab, including scenes with large flat objects. The solution covers a wide range of scenarios, including hazardous and extreme situations, helping OEMs identify potential problems earlier and avoid failures after release as much as possible.
Test complex real-world environments
When testing radar sensors, if the number of targets is not large enough, it is impossible to reflect the complete driving scene and reproduce the complexities of the real environment. The Radar Scene Simulator enables OEMs to set a variety of environmental condition variables, traffic density, speed, distance, and total number of targets in the lab to truly simulate a realistic driving scenario. Whether it's a common or extreme situation, test in advance to minimize risk.
Accelerate learning
Radar scene simulators provide a definitive, real-world environment for testing today's complex scenarios that require road testing in the lab. With its groundbreaking test methodology, OEMs are able to test in advance using repeatable, high-density, complex scenarios that can include stationary or moving targets, as well as a variety of variable environmental features, significantly increasing the learning speed of ADAS/AV algorithms and avoiding inefficiencies caused by manual or automated testing.
Increase confidence in ADAS capabilities
Auto companies know that self-driving algorithms are not only complex to test — they also involve safety issues. Keysight's radar scenario simulators are ideal for self-driving technology developers who insist on "safety first." It enables testing of automotive radar sensors at a faster pace, features highly complex multi-target scenes and full-scene rendering capabilities, and simulates near and far targets in an open continuous field of view.
The Radar Scenario Simulator is part of Keysight's Automated Driving Simulator (ADE) platform, which Wascotech has built through years of collaboration with IPG Automotive and Nordsys. The ADE platform simulates the simultaneous connection of various related sensors on a car, such as global satellite navigation systems, connected car cameras and radar, in one system. With ADE, an open platform, automotive OEMs and their partners can focus on developing and testing ADAS systems and algorithms, including sensor fusion and decision-making algorithms. Automotive OEMs can also integrate the platform with commercial 3D modeling, hardware-in-the-loop systems, and existing test and simulation environments.
Keysight's Radar Scenario Simulator and ADE platform provide an ideal solution for automotive OEMs to realize new ADAS capabilities and ultimately the vision of fully autonomous driving.
Figure 13. Keysight AD1012A Radar Scene Simulator
Realize your mobility vision
Only an integrated real-world simulator can bridge the gap between simulation and road testing, enabling higher levels of autonomous driving. You need to make AV and ADAS software decisions on a holistic basis, not just what the test equipment presents.
Scan the code to get the full PDF version of the white paper
Welcome to the Intelligent Transportation Technology Group!