Edit/Green Lotus
Scientists and institutions devote a lot of resources every year to discovering new materials in the hope of providing catalysts for fuels. As natural resources dwindle and demand for higher value and advanced performance products grows, researchers are increasingly focusing on nanomaterials. But continuous experimental methods for identifying new materials impose insurmountable limits on material discovery.
Recently, researchers at Northwestern University and the Toyota Research Institute (TRI) applied machine learning to guide the synthesis of new nanomaterials, removing barriers related to material discovery. This trained algorithm can define datasets to accurately predict important catalysts that could be used for fuels in the clean energy, chemical, and automotive industries.
The study, titled "Machine learning–accelerated design and synthesis of polyelemental heterostructures," was published in the journal Science Advances on December 22, 2021.
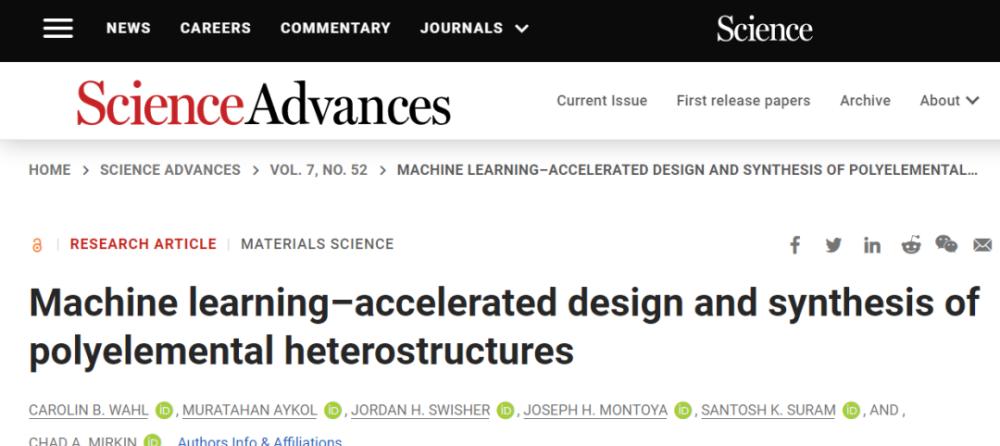
Chad Mirkin, an expert in nanotechnology at Northwestern University and the paper's corresponding author, said: "We asked this model to tell us that a mixture of up to seven elements could make something that hadn't been done before. The machine predicted 19 possibilities, and after experimentally testing each possibility, we found that 18 of them were correct."
Plot the material genome
Mirkin's invention of a data generation tool called "Megalibrary" has greatly expanded the researchers' horizons. Each Megalibrary contains millions or even billions of nanostructures, each with a slightly different shape, structure, and composition, all of which are position-coded on a 2×2 square centimeter chip. To date, each chip has contained more new inorganic material than scientists have collected and classified.
Mirkin's team developed Megalibraries using a technique called scanning probe block copolymer lithography (SPBCL), a massively parallel nanolithography tool capable of depositing hundreds of thousands of features per second for specific locations.
NP datasets and statistics for simulated optimization activities.
When mapping the human genome, the scientists were tasked with identifying combinations of four bases. But the "material genome" includes nanoparticle combinations of any 118 elements available in the periodic table, as well as parameters such as shape, size, phase morphology, crystal structure, and so on. Building smaller subsets of nanoparticles in the form of Megalibraries will bring researchers closer to completing a complete map of the material's genome.
"Even if we can make materials faster than anyone else on the planet, it's still a drop in the ocean of possibilities." Mirkin said. "We want to define and mine the material genome, and our approach is through artificial intelligence."
Machine learning applications are well suited to address the complexities of defining and mining the genome of materials, but are limited by the ability to create datasets to train algorithms in space. Mirkin says the combination of Megalibraries and machine learning may eventually solve this problem by understanding which parameters drive certain material properties.
Materials that chemists cannot predict
If Megalibraries provides a map, machine learning provides a legend.
Mirkin said using Megalibraries as a source of high-quality and large-scale materials data for training AI algorithms allows researchers to get rid of the "keen chemical intuition" and continuous experiments that often accompany the material discovery process.
In this study, the team compiled previously generated Megalibrary structural data consisting of nanoparticles with complex composition, structure, size, and morphology. They used this data to train the model and asked it to predict the composition of the four, five, and six elements that would produce a certain structural feature. Of the 19 predictions, the machine learning model correctly predicted the new material 18 times—with an accuracy of about 95 percent.
Closed-loop optimization for discovering quaternary metal SINPs.
With little knowledge of chemistry or physics, using only the training data, the model was able to accurately predict complex structures that never existed on Earth.
"As these data suggest, the application of machine learning, combined with Megalibrary technology, could be the way to ultimately define the genome of materials." Joseph Montoya, a senior research scientist at TRI, said.
Metal nanoparticles show promise for catalyzing key industrial reactions such as hydrogen evolution, carbon dioxide (CO2) reduction, and oxygen reduction and precipitation. The model was trained on a large dataset built by Northwestern University to look for polymetallic nanoparticles with parameters set around phase, size, and other structural features that alter the properties and functions of the nanoparticles.
Megalibrary technology could also drive discoveries in many areas that are critical to the future, including plastic upcycling, solar cells, superconductors and qubits.
The team is now using the method to find catalysts that are critical to fuel processes in the clean energy, automotive and chemical industries. Identifying new green catalysts will convert waste and large quantities of feedstock into useful substances. Production catalysts can also be used to replace expensive and rare materials.
Thesis link: https://www.science.org/doi/10.1126/sciadv.abj5505
Related: https://phys.org/news/2021-12-machine-synthesis-complex-materials.html
Artificial Intelligence × [ Biological Neuroscience Mathematics Physics Materials ]
"ScienceAI" focuses on the intersection and integration of artificial intelligence with other cutting-edge technologies and basic sciences.
Welcome to follow the stars and click Likes and Likes and Are Watching in the bottom right corner.