The Heart of the Machine is original
Machine Heart Editorial Department
How does a novice driver who has just started on the road grow into an "old driver"? Obviously, he must have experienced enough time and mileage of driving practice to be able to skillfully and calmly deal with various possible road conditions and emergencies. So although the automatic driving system will also undergo a large number of real road tests before being put into use, even if scientific civilization is quite popular today, there are still many people who still cannot "safely hand over the driving matter to AI", after all, what is in front of people is endless controversy and unclear driving accidents, and the occurrence of accidents may be caused by multiple subjective and objective factors such as technology, algorithms, roads, data, transmission, weather, drivers, etc., and the division of rights and responsibilities is very difficult.
Specifically from the algorithm level, because the driving scenario naturally has higher requirements for safety, which requires the automatic driving algorithm to be interpretable; but the decision planning module of the current automatic driving system is mostly based on training data, because the existing data set generally lacks intermediate data or state data, resulting in the algorithm in extreme cases is difficult to make a completely correct decision in time. "The algorithm has not yet reached the level of complete 'credibility', which to a certain extent brings difficulties to the determination of liability after the accident." Chen Junyan, director of the Artificial Intelligence and Big Data Division of the East China Branch of the China Academy of Information and Communications Technology, analyzed it.
Chen Junyan, Director of the Artificial Intelligence and Big Data Division of the East China Branch of the China Academy of Information and Communications Technology
Because it is impossible to explain the boundaries of authority and responsibility in an accident that has occurred through algorithms, humans can only try to rationalize the behavior of autonomous driving, but the black box nature of deep learning models makes this process difficult. He Fengxiang, an algorithm scientist at the Jingdong Exploration Research Institute, believes that many AI algorithms at this stage are still based on a black box model, and if you don't know much about the mechanism behind the algorithm, you don't know where the risk comes from, nor can you identify the risk mechanism and risk scale, let alone manage the risk well. In this case, AI algorithms cannot be applied in key areas, such as medical diagnosis, autonomous driving, etc., where people have high expectations, and more "life-threatening" industries. "Next, we need to have a deep understanding of the algorithm's behavior before we can design an algorithm that can be trusted on this basis."
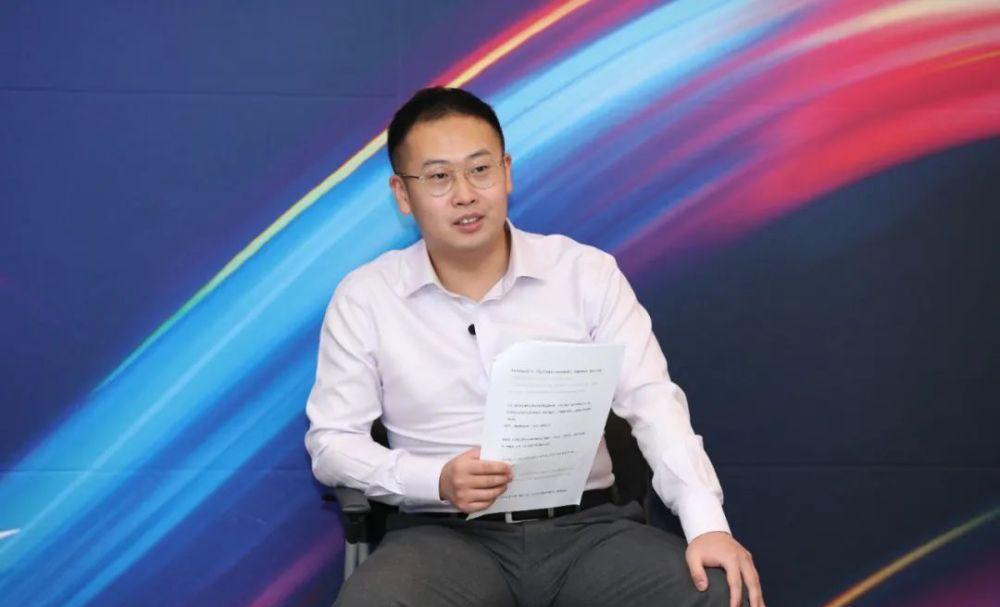
He Fengxiang, algorithm scientist at Jingdong Exploration Research Institute
In addition, it is worrying that whether it is the basic location information such as addresses and routes, or personalized information such as music and chat content, once the important data in it is stolen, tampered with or abused, it will produce serious legal liability incidents, damaging the property safety and even life safety of the data subject. How to protect such a large amount of data and prevent data misuse? It is also a problem that must be solved in the process of automatic driving landing.
Trusted AI, the security guard of the digital age
Today, artificial intelligence technology has become a resource like hydropower, integrated into all aspects of people's daily lives, from shopping recommendations, medical education, to biometrics and industrial intelligent manufacturing, there are many opportunities. However, while we are immersed in the convenience and efficiency of life brought by AI technology and the rapid development of the industry, problems such as uncontrollable technology, data security, and privacy leakage caused by its "black box mode" occur from time to time.
Is AI trustworthy? How to build mutual trust between people and the system? What is the measuring scale? How to better use AI technology for people... Related issues have gradually become a topic of great concern to academics, industry, governments, and even the general public in recent years.
The concept of trusted AI first appeared at the Xiangshan Science Conference in November 2017, first proposed by Chinese scientist He Jifeng in China, and with the rapid development of artificial intelligence in recent years, people's understanding of trusted AI has become clearer and even deeper.
In October 2019, JD Group proposed for the first time at the Wuzhen World Internet Conference that JD.com practiced the six dimensions of "trustworthy AI". In July 2021, the first "Trusted Artificial Intelligence White Paper" jointly written by the China Academy of Information and Communications Technology and the JD Exploration Research Institute was officially released, which for the first time systematically proposed a panoramic framework for trusted artificial intelligence and comprehensively expounded the characteristic elements of trusted artificial intelligence. "We are in line with the white paper's thinking, and conduct research on trusted AI from four aspects, namely stability, interpretability, privacy protection, and fairness." He Fengxiang, an algorithm scientist at the JD Exploration Research Institute, said.
Throughout the world, the development of trusted AI technology has a similar path to follow: starting from the basic theory, from the theoretical problems, theoretical results are in-depth, designing AI algorithms that can be trusted, and finally trying to apply these AI algorithms to products to complete the technology landing, and the current trusted AI as one of the three major research directions locked by the JD Exploration Research Institute is also the same. "In the process of work, we found that these four aspects are somewhat related to each other, and we hope that in the long-term exploration, we can put forward some unified theories to consistently describe trusted AI, rather than simply mechanically studying its four aspects separately."
Compared with Domestic, foreign companies start earlier in the specific layout of trusted AI technology. Taking privacy protection as an example, in 2006, Dwork et al. proposed a differential privacy model, which quickly replaced the previous privacy model and became the core of privacy research as soon as it appeared. At present, some companies have deployed differential privacy as a standard on a large scale, and for user data, they use differential privacy algorithms to anonymize and disrupt the collected data, so that the data cannot be located to specific users.
Needless to say, trusted AI involves a wide range of directions, each direction will extend a lot of specific tasks, and the realization of trusted AI is not a "day's work". In the view of He Fengxiang, an algorithm scientist at the Jingdong Exploration Research Institute, whether it is possible to establish a theoretical foundation by studying the operating mechanism behind AI and unify the theories in different directions is a difficult problem, of course, this is also a very core problem. If this problem is solved, subsequent algorithm research may be of great help.
"Walk on two legs" to promote the standardization of trusted AI
The standardization of trusted AI should be ahead of the implementation of the practice, which is another major consensus in the industry. The key to achieving the above goals is to learn to "walk on two legs", in addition to policy guidance, but also requires industry self-discipline.
From an international point of view, on the one hand, the credibility of AI is regulated in the form of guidelines and bills, such as the Draft Ethical Guidelines for Trusted Artificial Intelligence and the Code of Ethics for Trustworthy Artificial Intelligence issued by the European Commission's Advanced Expert Group on Artificial Intelligence (AI HLEG). This year, the EU Artificial Intelligence Act has also been officially released.
On the other hand, it is to exert force on the development of standards. For example, the National Institute of Standards and Technology (NIST) issued a Standard Proposal on Identifying and Managing AI Discrimination in June, followed by an AI Risk Management Framework in July.
In contrast, the domestic standardization development follows the same idea, including the Academy of Information and Communications Technology, including the Academy of Information and Communications Technology, as well as representatives of the business community like JD.com, are working hard to promote industry initiatives and standard development.
In July 2020, the five ministries and commissions issued the "Guidelines for the Construction of the National New Generation Artificial Intelligence Standard System"; at this year's World Artificial Intelligence Conference Trusted AI Forum, the "Initiative to Promote the Development of Trusted Artificial Intelligence" was officially released; at the same time, the Artificial Intelligence Governance and Trust Committee of the Chinese Intelligent Industry Development Alliance was also announced.
"We also hope to be able to assist in the formulation of standards from our own research results, and with standards, some research results can be better quantified." For example, provide some quantitative indicators to measure the trustworthiness of the algorithm, and set some thresholds as technical standards. In He Fengxiang's view, this is equivalent to "first making a well-formulated ruler, and then doing the measurement." ”
Where will the future path of trusted AI point?
In the process of trusted AI technology practice, enterprises will inevitably play the role of backbone, which can make trusted AI better land and go further. Especially in the links of data screening, algorithm optimization, and model design, to find the optimal solution to the problems of privacy leakage, algorithm bias, and content review, we must rely on the continuous exploration of enterprises.
In August this year, the launch of the enterprise-level multi-party security computing platform of Everbright Bank undertaken by Huakong Qingjiao is a visual case of enterprises helping to implement trusted AI. This is the first enterprise-level data circulation infrastructure platform officially put into production in the financial industry, marking that multi-party secure computing has truly opened up the last link of "industry-university-research" and stepped onto a key stage towards large-scale application.
It is reported that multi-party secure computing can ensure the privacy of input and the correctness of calculation at the same time, so as to realize the "available invisible, controllable and measurable" data. "Under the premise of no trusted third party, multi-party secure computing can not only ensure that the input information of the members of the parties involved in the calculation is not exposed through mathematical theory, but also obtain accurate calculation results." On this basis, the amount of data use can be controlled through the calculation contract mechanism, and the data abuse can be effectively prevented in combination with the blockchain depository. Under the premise of realizing data privacy protection and effective control of usage, there will be more opportunities for AI research and landing at the level of interpretability and fairness. Wang Yunhe, head of Huakong Clearance Standards and director of strategy, said.
Wang Yunhe, head of Huakong Clearance Standards and director of strategy
"Trusted AI involves a wide range of tasks, and the tasks involved are very complex. In terms of technology landing, we believe that we can establish an open system, and different manufacturers can cooperate to develop a unified standard, and eventually become a complete ecosystem. He Fengxiang suggested. It is understood that at present, the privacy computing, multi-party computing, federal learning and other technologies of the Jingdong Exploration Research Institute have been used in the output of external technology, and the relevant technologies for interpretability and stability have also been explored at the forefront, and related products will also be landed as soon as possible.
Since the academic community first proposed to actively study from all walks of life, and then to the industry to start the practice, the connotation of trusted AI is gradually enriched and improved, but the landing of trusted AI not only requires the support of advanced technology, but also needs to reach a consensus on concepts. The future development of trusted artificial intelligence will require all walks of life to work together to build a safe, fair and controllable future intelligent world.