Zhi DongXi (public number: zhidxcom)
Text | origin
| April
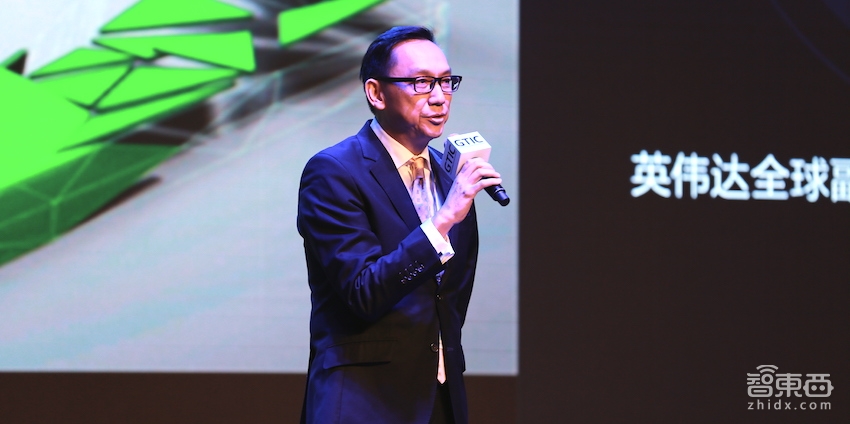
The third wave of artificial intelligence is surging and the industry is in a big wave, feeling the pulse of the times and seeing the future at the "gtic 2017 Global (Smart) Technology Summit".
On March 10th, the "gtic 2017 Global (Smart) Technology Summit" jointly organized by Zhidongxi, AWE and Jiguo was officially opened at the Shanghai Zendai Himalayas Center. Academia, the investment community, the entrepreneurial circle and the industrial chain are fiercely clashing here, and nearly 40 bigwigs such as nvidia, neato robotics, iFLYTEK, SenseTime, Coworth Robot, Ninebot, WM Motor, Singularity Auto, Yushi Technology, Goertek, Horizon Robot and so on take turns on the stage.
As the highest-spec summit in the field of artificial intelligence in the first half of 2017, gtic focused on the fields of "robot industry", "new forces in automobiles" and "home and internet of things", discussed the nuggeting opportunities, consumption upgrades and ecological construction under technological change, and brought the most cutting-edge practical experience and judgment.
In the morning speech session, Li Shenwei, global vice president of NVIDIA and general manager of the enterprise business unit in China, delivered a speech with the theme of "Artificial Intelligence Deep Learning - A New Computing Model", which detailed the process and ideas of NVIDIA to become the industry hegemon by virtue of the wave of artificial intelligence.
Here is a summary of the main points of the speech:
1. The birth of the cuda architecture is a key node in deep learning. The GPU before the NVIDIA CUDA architecture did not have too strong support for deep learning computing capabilities, and after the birth of the CUDA architecture, ordinary scholars and programmers can easily use the GPU for high-performance computing. In 2006, deep learning and NVIDIA GPU were used for the first time in the image recognition database imagenet competition, which increased the recognition rate of imagenet to 85%, and since then, deep learning and GPU have been jointly launched.
In 2012, NVIDIA and Ng explored how GPUs can accelerate deep learning, and now Baidu's speech recognition rate has surpassed that of humans. In 2016, the imagenet recognition rate has reached 96%, surpassing that of humans.
2, whether it is some manufacturing, design, or to the production of movies that we are all familiar with, NVIDIA is the standard of this industry. And in 2006, after we entered the high-performance budget, 70% of us have used Nvidia products as the family of high-performance computing.
In the wave of AI, we now see that basically 100% of the research in the field of artificial intelligence is developed on this platform supported by Nvidia. Nvidia for artificial intelligence and deep learning, we also released the software development platform SDK, that is, the home library of home learning in Kikuda, which currently has more than 200,000 downloads in the world.
3, GPU has become the optimal platform for high-performance computing, CUDA has 300,000 developers, and occupies the vast majority of high-performance computing applications. In terms of solutions, NVIDIA offers the best computing platform in the industry, tesla p40.
For customers who lack big data centers, such as university research, as well as startups and research institutes, the molded supercomputer dgx-1 is a good choice. It integrates the most advanced GPUs, as well as integrates and optimizes the familiar deep learning platform, dgx-1 is not only very common in foreign countries. Its entire computing power, although it is only a small machine, but its entire computing power is like a single chassis put 250 server computing power, you do not need a large data center, you can have a device equivalent to 250 server computing power to engage in AI and deep learning research.
4, all this is from the end of 2012, Nvidia noticed this wave of deep learning after the wave, we have made a lot of investment, in just 3 years, GPU hardware has developed three generations, the overall performance has been 65 times improved, this is NVIDIA's commitment to deep learning and AI industry.
The following is the full text of Shen Wei's speech at the "gtic 2017 Global (Smart) Technology Summit":
Today, I am particularly honored to report to you on NVIDIA's journey into high-performance budgeting in recent years, as well as all aspects of deep learning and artificial intelligence.
Allow me to take a few minutes to introduce you to NVIDIA. Nvidia was founded in 1993, in 1999 we invented the GPU, we are the world's largest GPU company, you guys on Nvidia before entering deep learning, Nvidia is the world's largest game computing platform provider, the world has more than 100 million users in the use of Nvidia game computing platform.
NVIDIA is also a professional provider of graphics work displays, display computing platforms, and is also the standard in this industry. We now see that whether it is some manufacturing, design, or the production of movies that we are all familiar with, NVIDIA is the standard of this industry. And in 2006, after we entered the high-performance budget, 70% of us have used Nvidia products as the family of high-performance computing.
In the wave of AI, we now see that basically 100% of the research on AI in the field of artificial intelligence is developed on this platform supported by NVIDIA. Nvidia for artificial intelligence and deep learning, we also released the software development platform SDK, that is, the home library of home learning in Kikuda, which currently has more than 200,000 downloads in the world.
Where does it all start? 2012 is the first year of AI, or the year of the explosion of deep learning. We Chinese Light Li Feifei hosted a competition at Stanford University, and a student who used deep learning methods for the first time, plus the participation of NVIDIA's GPUs, not only to get the first place in this competition in one go. At the same time, it also changed the competition from the original competition that only did computer vision algorithms, from the previous highest recognition rate, only 74%, in 2012 after using deep learning, and NVIDIA's GPU, suddenly not only won the first place, but also pulled the entire recognition rate to 85%. Since then, the world of deep learning and GPU has begun.
Since the 2012 competition, the methods of deep learning have been out of control. In 2012, Nvidia was honored to join us, now Chief Scientist of Baidu, to publish how to accelerate the development of deep learning through GPU's high-performance budgeting capabilities. At the same time, from 2012 to the present, after the competition increased from 2012 to 85%, there were no algorithms participating in the competition, and by 2016, the entire recognition rate of deep learning was more than 96%. This deep learning recognition rate has exceeded the human recognition rate of images. Of course, not to mention that in 2015, Baidu's breakthrough in deep learning, especially in deep speech recognition, has surpassed the recognition ability of human speech recognition. Also, last year, Alpha Dog, in the progress of Go, is the use of a large number of massive deep learning CPU, which in the past completely could not be achieved.
Speaking of this place, you may have a lot of doubts. Is this happening suddenly, or is there a deeper context? Allow me to report to you on the evolution of history.
Talking about the process of NVIDIA from the invention of the GPU in 1999 to the process of just learning, we have to talk about our important breakthrough in 2006, and it was also the first time in 2006 that Kuda released such an architecture. Before 2006, 90% of the image operations of the image GPU were for image operations, which is very closed and provided for game developers and developers. For general researchers, or academics and researchers who have requirements for high-performance budgets, this is a very hard thing. Before 2006, if you want to use the GPU is very hard, I remember, I heard a while ago that domestic scientific research experts, in order to get better computing resources, they collected a lot of game cards, connected with the existing x86 server, is to explore how to better use the GPU computing power. To this end, large O&M companies are also seeing such a trend, so in 2006 we released such an architecture as Kuda. After the first time with this architecture, the average researcher can use ordinary Java plus language can use the gpu computing power, that is, from 06, NVIDIA officially entered the high-level budget capability, that is, from this time on, most of the world's scientific research and high-performance computer centers deploy NVIDIA equipment for computing and high-performance budget.
But there are a few big breakthroughs here, and I won't mention them again and again. One of them is to report to you, the 2012 competition to give everyone a deeper understanding of the use of GPU. By 2016, alpha dogs were also a practical case, and the application of GPUs went to another level.
As a worker who has been in high-performance computing since 26 years ago, GPUs have become the best choice. There are already more than 410 applications in high-performance computing, and basically 100% of everyone in artificial intelligence deep learning is accelerated on the GPU.
Here, I would like to take the opportunity to introduce to you what kind of solutions we provide in addition to the report on Nvidia's journey in high-performance budgeting and artificial intelligence. We have also made a lot of investment in deep learning, especially in the enterprise data center to provide solutions, no matter from offline learning, we have a very strong technical support, we also provide offline deployment, inference such a solution.
For example, our p40 is currently the best platform for deep learning used by most data centers, and I think it is the best platform for deep learning.
In addition, we also released products for online deployment or inference in data centers last year, very suitable when the offline training is completed, you have to do the application, how can you quickly combine your virtual results with your actual business, including the boundaries of a large number of videos, as well as real-time analysis, this is what we just released last year, very suitable as an online reasoning and application product.
Of course, you will propose, we don't have such a big data center, I am a research unit, I am just a startup, I want to develop artificial intelligence and deep learning, do I also have the opportunity to use NVIDIA's solutions? The answer is yes. We are also for university research, as well as startups, research institutes, we released a molded supercomputer called DGX-1 last year, which integrates the most advanced GPU, and integrates the familiar deep learning platform and optimizes it, DGX-1 is not only very common in foreign countries. It's like putting 250 servers in a single chassis, you don't need a big data center, you can have a device equivalent to 250 server computing power to engage in AI and deep learning research.
Of course, you will propose, we don't have such a big data center, I am a research unit, I am just a startup, I want to develop artificial intelligence and deep learning, do I also have the opportunity to use NVIDIA's solutions? The answer is yes. We are also for university research, as well as startups, research institutes, we released a molded supercomputer called DGX-1 last year, which integrates the most advanced GPU, and integrates the familiar deep learning platform and optimizes it, DGX-1 is not only very common in foreign countries. Its entire computing power, although it is only a small machine, but its entire computing power is like a single chassis put 250 server computing power, you do not need a large data center, you can have a device equivalent to 250 server computing power to engage in AI and deep learning research.
All of this began at the end of 2012, NVIDIA noticed this wave of deep learning, we made a lot of investment, in just 3 years, we have invested in GPU hardware has developed three generations, the overall performance has been 65 times improved, this is NVIDIA's commitment to deep learning and ai industry.
Also when it comes to deep learning and AI, NVIDIA's contribution is not just hardware in the GPU, but more importantly, how to make deep learning to AI practitioners, we even have a better development environment. So NVIDIA has made a very big investment in doing SDK, that is, in the software part of the investment.
For example, in the part of learning, NVIDIA has made a cudnn accelerator, for the development that everyone is familiar with, we have done a lot of optimizations, so that these AI and deep learning developers, it is easy to use these acceleration libraries, can better performance improvement. There are already more than 200,000 downloads worldwide in this section.
Also in terms of online deployment and inference, Nvidia has also made a lot of investment, when you deploy the results after deep learning training to a new generation of NVIDIA technology, you can do deployment and inference faster and more efficiently, including many deployed applications related to video and images, SDK is especially for deep learning in the deployment and application of this aspect of development, we can deal with video boundary code and updates at the same time.
Next, let's talk about some cases for NVIDIA, our solution, Google this part will not be discussed, may currently have more than 5,000 applications, this is not much to talk about.
In this part of the country, Baidu is a very typical customer of ours, and we are very grateful to Baidu's chief scientist for our support, we have made a lot of investment, whether it is in the recognition of faces, objects, all the way to automatic driving, we use a lot of GPU applications.
In addition, there is a big customer in China is Alibaba, which is also deep learning. There is a very good application, there is an application, I take out my mobile phone and take another picture, I can enter the Ali environment, this tie in which business. Not to mention that everyone in the double eleven, everyone in so many massive customer demand, how to deal with its customer service, there are many applications in this regard, are reflected through deep learning artificial intelligence methods.
The last application to share with you, but also recently everyone pays more attention to the smart city. You can note that in 2020, there are more than 1 billion cameras in the world, after the deployment of cameras, so much massive data, especially how to deal with image data, with the development of artificial intelligence and deep learning, just reported to the experts, whether it is through the learning of the NVIDIA platform, and for the online real-time coding processing and analysis, I believe that NVIDIA in the application of smart cities, as well as the application of deep learning, we can provide better solutions.
Because of the time relationship, I am here to introduce NVIDIA's solution, NVIDIA's entire transformation, and the application scenario, I believe this is just our company and artificial intelligence and deep learning just started the scene. I believe that with the joint efforts of all experts today, AI and artificial intelligence will be better, we at NVIDIA are very happy to provide such a platform to promote the development of artificial intelligence with you, thank you!