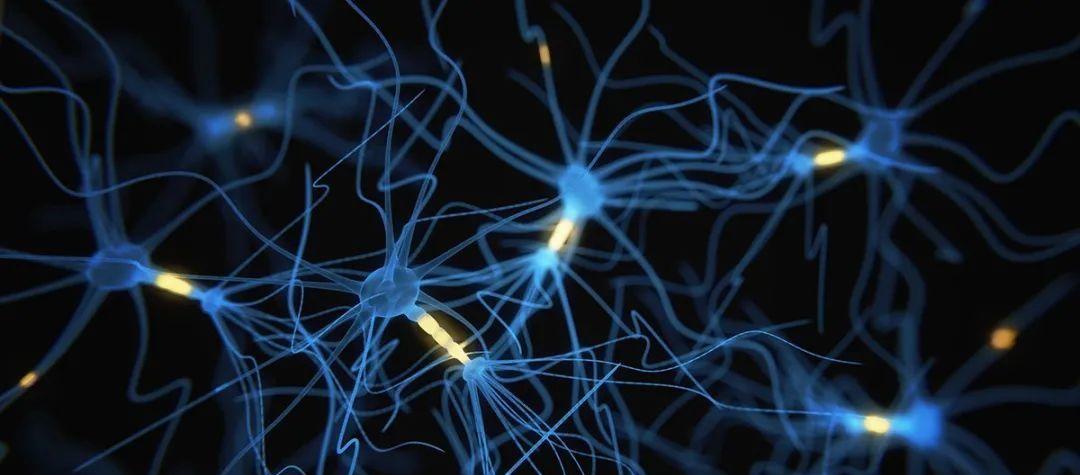
Author | Zhang Tielin, Xu Bo
Paper title: A Mesoscale Plasticity for Efficient AI Learning
In the field of artificial intelligence, the backpropagation algorithm (BP), which is widely used in artificial neural networks, adopts a global optimization strategy, which is an end-to-end learning method with excellent performance, but the learning process is energy-intensive and lacks flexibility. The joint research team of Xu Bo and Pu Muming of the Center for Brain Intelligence Excellence of the Chinese Academy of Sciences has recently made important progress in more efficient and flexible brain-like local learning methods with the help of the mesoscopic scale self-organizing backpropagation mechanism (SBP) found in biological networks.
The discovery of SBP dates back to 1997. Writing in the journal Nature, Pu's team found that neurons in the hippocampus can self-organize the plasticity of long-term depression (LTD) to three directions, namely Presynaptic lateral spread, postsynaptic lateral spread, and postsynaptic lateral spread. Backpropagation[1], the discovery of the self-propagation neural plasticity mechanism (SBP). Subsequent studies have confirmed that the SBP phenomenon is pervasive, covering not only more neural regions such as the retina-capping system[2], but also more types of plasticity[3], such as long-term potentiation (LTP). This mechanism is due to the natural reverse transmission of molecular modulation signals within biological neurons, which is thought to be key to leading to efficient feedback learning in biological neural networks [4]. Inspired by this mechanism, the research team constructed a separate mathematical model of the backpropagation direction (third direction) of SBP (Figure 1A), focusing on the fact that the plasticity of neuronal output synapses can be backpropaged into input synapses (Figure 1B), and the occurrence of plasticity can be achieved by timing-dependent synaptic plasticity (STDP) or by artificial local gradient adjustment. In the learning process of the standard three-layered pulse neural network (SNN), the SBP mechanism can self-organize the learning of the weights of the previous layer of the network, and can combine short-term plasticity (STP), membrane potential (Homeo-static membrane potential), etc., to form a more powerful SNN combination learning method (Figure 1C). In the learning of a class of artificial neural networks (ANNs) such as the Restricted Boltzmann machine (RBM) (Figure 2A), the SBP mechanism can also replace some of the BP mechanisms during the iteration process, enabling alternating collaborative optimization (Figures 2B-E). In view of the difference between SNN and RBM, the team set two different energy function constraints to ensure the smoothness of network parameter learning during training. In addition, the research team specifically proposed a new method for counting energy expenditure during training (Figure 3). On multiple standard datasets such as image classification (MNIST), speech recognition (NETtalk), and dynamic gesture recognition (DvsGesture), the SBP mechanism achieves lower energy consumption and higher precision SNN local learning by combining other plasticity mechanisms (Figure 4). In ANN-RBM learning, the SBP mechanism can also replace the BP mechanism extensively for global and local cross-learning, reducing computational energy consumption without losing accuracy (Figure 5). The researchers believe that SBP is a special bioplasticity mechanism of a mesoscopic scale, which has gained a wide range of combinatorial optimization advantages in both SNNs and ANNs, and has great repercussion for further in-depth exploration of brain-like local computing. The essence of biological intelligence computing is likely to be self-organizing local learning that flexibly integrates multi-class microscopic, mesoscopic and other plasticity mechanisms, combined with the remote projection network structure given by genetic evolution, to achieve efficient global optimization learning effects. This work can further guide the deep integration of biological and artificial networks, and finally achieve a new generation of artificial intelligence models with high energy efficiency ratio, strong interpretability and high flexibility. The Self-backpropagation of synaptic modifications elevates the efficiency of spiking and artificial neural networks was published online on October 20, 2021 (ET) in Science Advances, a sub-journal of Science. Zhang Tielin, associate researcher of the Research Center for Brain-like Intelligence of the Institute of Automation, Chinese Academy of Sciences, is the first author, Researcher Xu Bo is the corresponding author, and Cheng Xiang (doctoral student), Jia Shuncheng (doctoral student), Researcher Pu Muming and Researcher Zeng Yi are co-authors. The relevant research work has been funded by the National Natural Science Foundation of China and Pilot B projects.
The address of the relevant paper can be found in: https://www.science.org/doi/10.1126/sciadv.abh0146
Figure 1: Application of SBP in SNN.
(A), SBP plasticity mechanism. (B), the local backpropagation of SBP in SNN. (C), combinatorial optimization of SBP and other plasticity mechanisms in SNN.
Figure 2: Application of SBP in RBM.
(A), combinatorial optimization of SBP and BP in RBM. (B), the alternating collaboration process of SBP and BP. (C), the standard Sleep Phase in RBM. (D), a Wake Phase containing SBP. (E), a Wake Phase containing BP.
Figure 3: Calculation method of training energy consumption.
(A), the average number of iterations. (B) the complexity of the algorithm in each iteration.
Figure 4: Performance comparison on three datasets: MNIST, NETtalk, dvsGesture.
(A, C, E), SBP achieved the optimal performance of SNN based on gradient and plasticity methods, respectively. (B, D, F), SBP achieved the lowest energy consumption of SNN based on gradient and plasticity-based methods, respectively.
Figure 5: SBP helps RBMs improve accuracy and reduce energy consumption.
(A-C), in the MNIST dataset, SBP can reduce the training error of RBM by a small amount (A), can balance accuracy and energy consumption at the same time to obtain the optimal number of Wake Phases (B), and can significantly reduce training energy consumption (C). (D-I), in the NETtalk and DvsGesture datasets, SBP came to similar conclusions as in MNIST.
bibliography:
[1] Fitzsimonds, R. M., Song, H. J. & Poo, M. M. Propagation of activity-dependent synaptic depression in simple neural networks. Nature 388, 439-448, (1997).
[2] Du, J. L. & Poo, M. M. Rapid BDNF-induced retrograde synaptic modification in a developing retinotectal system. Nature 429, 878-883, (2004).
[3] Du, J. L., Wei, H. P., Wang, Z. R., Wong, S. T. & Poo, M. M. Long-range retrograde spread of LTP and LTD from optic tectum to retina. Proceedings of the National Academy of Sciences of the United States of America 106, 18890-18896, (2009).
[4] Bi, G. & Poo, M. Synaptic modification by correlated activity: Hebb's postulate revisited. Annual Review of Neuroscience, 24, 139-166, (2001).
Lei Feng network