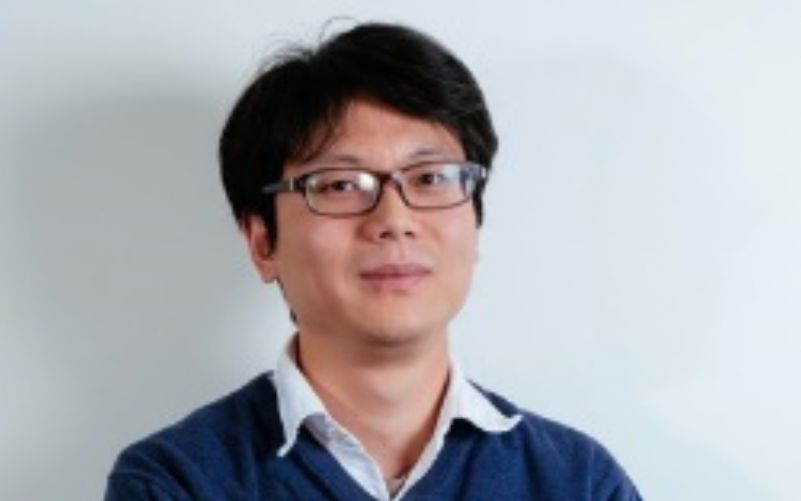
Introduction: Chao Wu, researcher and doctoral supervisor of Zhejiang University, director of the Center for Computational Social Sciences, member of the Collaborative Innovation Center for Artificial Intelligence of the Ministry of Education, whose main research direction is distributed machine learning
Why pay attention to the health code? When the health code first came out, CCTV Bai Yansong talked about the health code technology and its application when interviewing the official in charge of the health code in Hangzhou. When I began to pay attention to the mechanism and problems of health codes, I thought that there might be three problems, and so far I could prove that there would indeed be these problems, but now there is not enough empirical data, and health codes are still in the process of application, which is just my idea. I'm going to share these thoughts with you today.
First, I'll make a simple description of the health code, y=f(x). x is personal data, now or based on personal trajectory data, f is a kind of data modeling, this modeling now does not seem to be a machine learning model, basically or some rule system, may be similar to the model like a tree model, judge the trajectory, where to go or contact with whom to belong to a certain category, the result is a red, yellow and green code. This is a very typical classification problem, converting x data into y classification. This is a simple formal description of the health code problem.
I think there will be three aspects of the health code, one is interoperability, one is false negative, and the other is privacy protection.
The first is interoperability. Many places in the country have begun to implement health codes, and various enterprises and local governments have set up procedures similar to health codes. However, there will be differences in the situation in different places, and it is difficult for various enterprises to form a completely unified platform. If these health codes are to be put together, the easiest or most realistic way is mutual recognition.
So far, in the actual process, I have found that it is also based on the idea of mutual recognition. For example, in Shanghai, it is a green code, and it can also be recognized in Hangzhou. However, mutual recognition will have great problems, mutual recognition is only to believe in each other's classification results y, but not necessarily believe in each other's classification rules, the f of each subject, that is, the rules and standards are not the same. For example, the rules for generating green codes in Zhejiang may be different from the rules for generating green codes in Heilongjiang.
And it is very important that the basis of informatization in various places is different. For example, in Zhejiang, people use Alipay more, the frequency of background Alipay collection is higher, and the accuracy of location trajectory data is higher. However, other places may have less data collection in this regard, and can only use the data of the mobile phone base station, and the spatial distribution rate is low. Therefore, the basis of informatization is different and the rules are different, which will cause the standards for generating red, green and yellow codes to be different. If the standards in some places are relatively low, it will become a gap and a short board in the whole country.
Why is there no problem with health codes in our country? Because the whole epidemic in our country is good, in the case that most people have no possibility of disease, if it is judged that 100% of the people are green codes, there will be no problem. But if the form of health code is applied to the United States and Europe, the problem of interconnection will be more obvious.
And the ability of provinces to deal with the outbreak varies greatly. We recently did a study, but also the problem of y = f(x), this y is the severity of the epidemic in each province, not entirely measured by the number of cases, we standardized the number of cases with the floating population, imported cases, and calculated the results of epidemic control.
x is a model made with the characteristics of meetings, surveys, policy tools, access to public services, trust in the government, social capital, etc., and found that we can use these indicators to predict the ability of different provinces to control the epidemic. We have a lot of candidate indicators, but these are the most relevant, and the accuracy of the features will be higher, but there will be a problem of overfitting.
To put it simply, except for a few provinces such as Heilongjiang and Jiangxi, where our predictions are not very accurate, our predictions are more accurate in other provinces. However, the study found that policy tools and final results for dealing with outbreaks varied widely from province to province. If the policy of national unified standards such as health codes is implemented, problems caused by basic differences will arise.
If you want to really play a unified role in the more severe public health crisis, you should first achieve data interoperability or data standards and rules can be interoperable, data interoperability is more difficult, but the standard should be unified.
The second is false negative. This is a question about the y classification error. Our current f is basically a rules-based algorithm, so the accuracy is limited and can only be considered in very common cases, but it is difficult to deal with some fine cases, especially to deal with ambiguity. Rules are made by people and discovered by observation, but there are many boundary conditions, abnormal situations, and situations that require semantic understanding, which are difficult to calculate in y.
We think that there are many cases in which green codes should not be green codes, but they are identified as green codes. For example, opening the health code before the patient is diagnosed is a green code, these are very typical false negatives, in addition to these, is there more false negatives? If a health code had appeared in the early days of the pandemic, would the false negative ratio have been higher?
We made a model to test the above idea, which is based on the traditional SIR model. SIR is a classic model of epidemic transmission, assuming that the process of infection is a Markov process. The traditional SIR model of the infection factor is a pre-set value, but we felt that this value should change with different stages of the epidemic, so we used machine learning to fit this factor.
Take Italy as an example, because Italy is now relatively complete in data and has experienced a process from the outbreak to the peak to the present, which is conducive to model prediction. The red line is the actual number of infected patients who are diagnosed each day. The blue line is predicted when there is no false negative, based on available data and assuming that nucleic acid testing is accurate.
It can be found that the actual confirmed case is very different from the prediction of no false negative situation. When we change the false negative ratio, when the false negative ratio is 0.4%, it will fit very well. That is to say, the number of false negatives in the population does not need to be particularly high, and 4 out of 1000 people are false negatives, there will be a relatively large gap. If the probability of false negatives increases to 0.8%, the gap will be even greater, the extension of the epidemic will be longer, and the total number of infections on the right will increase by a lot.
This experiment tells us that false negatives do not need to have too many people, but they can have a great impact during transmission. False negatives are caused by the methods we detect. In the detection method, the health code is one way, and the nucleic acid test is also a way. In addition to nucleic acid testing in China, the health code will become an important source of false negatives, although the epidemic situation is now good, the problem is not too obvious, if we apply the concept of health codes to other countries, the impact of false negatives will be very obvious.
The third is privacy protection. Recently, in Zhejiang, especially in Hangzhou, there is a big controversy over the health code. Hangzhou is now upgrading the health code, hoping to become a normalized management tool. In addition to health codes, there are now various new codes such as enterprise codes. The range of health codes is also expanding. I have heard Hangzhou imagine that after the epidemic, the health code can be used to see a doctor, buy drugs and other normalized applications, will put people's exercise, drinking, smoking these conditions are added to the health code, causing everyone's disgust, this is a very direct violation of privacy protection.
Health codes involve two privacy protection issues. First of all, the data collected by the health code is very sensitive, and our personal health data and trajectory data are very sensitive data. In privacy protection, trajectory data is the core protected data. And this data is now processed in a centralized way, such as collecting to the big data bureau, Ali, and telecommunications departments for centralized processing.
If there are other data sources, such as Alipay's consumption records and personally identifiable information, these databases, if combined, can mine more private data. Another issue is the retention period. Both of these aspects can create privacy leakage problems.
Many people say that the epidemic is an emergency, at this time utility is the most important, privacy can be sacrificed, even in normal circumstances, we often say that for convenience, we will sacrifice some privacy. Navigation software, for example, must obtain location data. We always think that utility and privacy are antithetical.
But I think that's an excuse, and a lot of times we don't focus on improving technology so that it can be useful without invading privacy. For example, the camera of the mobile phone, the convenience and quality of the mobile phone in the past were opposites. Now there is a great improvement in mobile phone lenses, especially algorithms can make up for the lack of lenses, at this time the convenience and quality of mobile phone photography are not opposed.
The same is true for privacy and utility issues. For example, the collection of location data of this epidemic, MITT proposed an algorithm, the local non-stop generation of random numbers, through Bluetooth and other mobile phones to exchange random numbers, other mobile phones have a database, save and it is close to it and a certain period of time these devices all generated random numbers, the exchange is Bluetooth exchange, do not need to go through the central server, this is the way of P2P.
When it is found that a person is diagnosed, the mobile phone database sends its own historical random numbers to the central server, and each user's local data is compared with the central server data. This is to get the data of the central server for comparison, there is no need to upload their own random numbers, if you find that the random numbers of these people who pass around match the random numbers infected by the central server, it is possible to be infected. In addition to the fact that the transmission of the final comparison data is centralized, the others are all P2P, do not need to collect location data, and are anonymized, which is a good idea.
Another idea is the research I'm doing now, distributed machine learning modeling algorithms. This algorithm is based on federated learning algorithms, and its core concept is to decentralize modeling to the user's localities. The original model is to collect data to the center, like the health code needs to collect everyone's personal data information to Alipay, and then it gives the corresponding health code. Can you put Alipay or the operator's algorithm on the mobile phone, and generate the health code locally by ourselves, this is the idea of federal learning, we will further optimize on the basis of federal learning to achieve privacy protection.
There are several aspects of work we do, the first of which is further decentralization. Federated learning has a central server, the role of this central server is to distribute the initial model, the local model updates, such as machine learning, the update is passed to the central server, do aggregation, at this time the central server still has the risk of privacy leakage. If the update frequency is frequent, we can estimate the data for each node in reverse.
We consider whether we can further decentralize, now the practice is to take the blockchain smart contract to do, use the smart contract to run the original central server model distribution and aggregation operations, use the encryption algorithm to generate some random numbers, let some users add random numbers, some users subtract random numbers, the total number of aggregations is unchanged, and each user gradient is protected.
The second is that we are also doing algorithms for model aggregation, and each node has an algorithm to determine its own health status or whether it is a green code, and has its own model. Aggregate the models together to get a better model. At this time, if you use the traditional algorithm, it is to do a weighted average of the parameters, which will cause some problems, such as model isomorphism. If there is a node with a very large amount of data, it will slow down the performance of the entire model. So we are now using the distillation method, and now the experiment has achieved better results.
The next job is to do data pricing, and after the model is aggregated, we have to judge how much data on each node, each user, each individual, and each mobile phone contributes to the overall modeling. The core idea is to use multi-party cooperative game computation, which is very complex, so we are now using a tree-like model, using a cross-cutting method to form the structure of several trees. All in all, our goal is to use these technologies to solve the privacy protection problem in the privacy protection gap such as the health code.
We established the Computational Social Science Research Center at Zhejiang University, which provides models, algorithms and data for computer social science problems such as traffic problems, epidemic transmission, and social governance problems. Another direction, and the direction we pay more attention to, is how the social sciences can raise new scientific questions to computational science and data science. Identifying problems that our original algorithms couldn't solve to drive new scientific research, such as distributed modeling is an example.
For example, when we do machine learning image problems, it is difficult to encounter data dispersion and protect the privacy of data nodes, but put into the social science scenario, put into the epidemic scenario, smart city scenario, this problem will soon be highlighted. At this time, we need to put forward new requirements to computing science, whether we can make a good model in the case of decentralized data, and this model can deal with governance issues in the case of privacy protection.
Editor: Li Biying
Contribute, cooperate, contact us: [email protected]