At the beginning of the epidemic in early 2020, the official account of Tsinghua University once offered a tweet titled "Tsinghua Teachers Upgrade the "Top Ten Artifacts" and Attend Classes With Full Force", telling the story of how a teacher in the Software College worked from home and explored a set of cloud devices that prevented students from being lazy and helped students attend classes remotely.
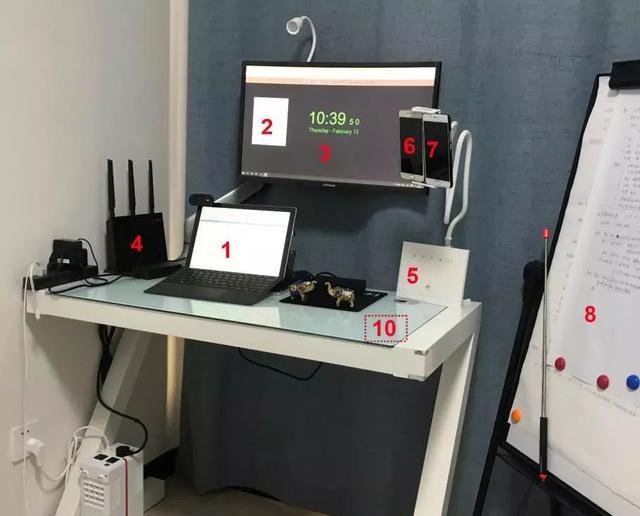
According to the official report at the time, the teacher swore an oath: "It can't be said that it is foolproof, but there are 10 major 'artifacts' protectors, whether it is power outage, network failure, hardware crash, software crash, can not stop my heart in class!" 」 True students are sad to hear, and tears are shed by hearers.
This teacher, Yang Zheng, the youngest Chinese scholar to be selected as a IEEE Fellow in 2022, is only 38 years old. He is also one of the few university scholars in China so far whose title was only associate professor when he was selected as an IEEE Fellow.
Leaving aside the funny BGM style, Yang Zheng's seriousness and rigor in scientific research discussion are equally impressive.
Yang Zheng studied under Liu Yunhao, a pioneer of the Domestic Internet of Things, which seems to have nothing to do with artificial intelligence, but coincidentally, the direction of his research is the intersection of the Internet of Things and artificial intelligence - intelligent wireless perception. The reason why he was selected as Fellow by the IEEE in 2021 is also his contribution to intelligent wireless perception.
Around the "intelligent wireless perception", AI Technology Review conducted in-depth discussions with Professor Yang Zheng and found many interesting points in this direction.
For example, the same is "recognition" and "detection", the most widely used in artificial intelligence is computer vision, but this direction is mainly through visual capture of environmental information and then analysis, while Yang Zheng's research on intelligent wireless perception technology can only rely on radio frequency signals (such as WiFi, 5G) to grasp the information in the environment. The latter removes the dependence on vision, which can also well eliminate the current computer vision in the landing applications face privacy, occlusion, low light and other issues.
In other words, wireless perception brings new "senses" beyond vision to AI.
In addition, many studies of wireless perception are also the technical basis for the current hot "metacosm". For example, The work recently proposed by Yang Zheng's team, SoftUpAR, deeply integrates wireless perception technology and mixed reality technology to help AR applications render continuous and smooth three-dimensional special effects on mobile targets, which is a typical case of intelligent wireless perception empowering the metaverse.
What is the "sacredness" of wireless perception based on RF signals? Here's how.
1. "Knowing" and "Wisdom"
From ancient times to the present, human beings' pursuit of "truth" can be summarized from "knowing" the physical world to "wisdom" that grasps the laws of all things.
However, due to limited cognitive conditions, people often encounter challenges in the process of realizing "wisdom". In the book "Republic", Plato once used the "cave fable" to describe the limitations of human understanding of the world: a beam of light enters the cave and projects external things on the cave wall, and the people in the cave can only observe the outside world through projection.
Note: Plato's "Cave Fable" experiment
Later, with the advancement of modern civilization, although human beings have the assistance of science and technology in the way of perceiving the physical world, they have been able to break the limitations of subjective cognition and experience the evolution of multiple stages such as sensors, wireless sensors and sensor networks, but there is still a long way to achieve an era of "ubiquitous intelligence" in which everything is intelligently connected. And this is the goal of intelligent wireless perception.
"In fact, as early as two thousand years ago, the mainland thinker Xun Zi had already discussed the relationship between human perception and intelligence in the "Correct Name Chapter"—'those who know in people are called knowledge, and those who know something are called wisdom'. But Xun Zi and his old man certainly did not expect that one day "knowledge" and "wisdom" would be combined and extended from "people" to "things." Yang Zheng preached.
In simple terms, wireless sensing is a technology that utilizes ubiquitous wireless signals to achieve scene awareness.
These "ubiquitous wireless signals" come from a variety of wireless devices deployed around us, including Wi-Fi hotspots, Bluetooth, RFID, etc., which can not only transmit data and complete their own tasks (such as communication), but also "additionally" used to perceive the environment, because the radio waves generated by the signal transmitter propagate through multiple paths such as direct illumination, reflection, and scattering, and the multipath superimposed signal formed at the signal receiver carries information reflecting the characteristics of the environment.
Although these radio frequency signals cannot be directly felt by the eyes, they can be captured by wireless communication transceivers, becoming a "new organ" for perceiving the IoT world outside of vision.
Take, for example, fall detection based on RF signals. When people fall, it will cause changes in the wireless signal propagation path, which will affect the received wireless signal. Wireless perception technology analyzes the changes of wireless signals in the propagation process by extracting the corresponding characteristics, and summarizes the difference between the wireless signal changes caused by falls and other activities with the help of classification algorithms, and determines whether there is a fall action in the environment, so as to achieve fall detection.
Note: Wi-Fi signal visualization
In other words, wireless perception is the secret weapon of modern scientists to "shoot cattle in the air".
"So when you conspire against bad things in the future, in addition to closing the door, pulling the curtains, checking whether there is a bugging device under the table, don't forget to turn off the Wi-Fi haha." 」 Yang Zheng laughed.
By analyzing the characteristics of the received signal and obtaining the characteristics of the signal propagation space, wireless sensing technology can realize the perception of people and the environment without deploying special sensors, with the advantages of wide range of perception, easy maintenance, and strong universality, which has become a research hotspot in the field of Internet of Things in the past few years, and a large number of research results have emerged.
But in fact, the use of dedicated radio frequency signals for environmental detection and exploration is not a particularly new concept. Long before World War II, after the advent of radar, people have begun to use sonar and radio frequency signals in the military field to perceive targets in the area under test. In the decades after the war, radar has gradually moved from military to civilian, and there have been many different functions of radar, such as weather radar, speed radar, terrain tracking radar and so on.
So, why can wireless sensing technology still attract a large number of scientists at home and abroad to participate in it today? In recent years, researchers around the world have successively proposed a series of remarkable new achievements in the field of wireless perception, such as UW's WiSee, MIIT's WiTrack, UCL's Phaser and so on. Many developed countries, including the United States and the United Kingdom, have also listed wireless perception as the key support direction.
Yang Zheng's view is that although the concept of using wireless signals to achieve perception is not new, from the perspective of "ubiquitousity" and "universality", the use of commercial communication technology to achieve ubiquitous intelligent perception is still in its infancy, providing many researchers with more possibilities.
The year 2000 was a watershed. At that time, a wide variety of wireless communication technologies were booming. In the case of Wi-Fi, since the beginning of the 21st century, the number of Wi-Fi access points can be called "explosive growth", and wi-Fi access points have been deployed in almost every household and all kinds of indoor places.
"I think this is the biggest benefit to 'ubiquitous wireless perception' and the most solid research foundation." "Especially with the evolution of the 802.11n/ac/ax standard, the number of antennas in commercial equipment is increasing, and the spectrum width is getting larger, which also gives us greater confidence in the prospect of ubiquitous wireless perception and communication perception integration.""
In a sense, the Internet of Things is to solve the problem of "ubiquitous perception", and artificial intelligence is to solve the problem of "universal intelligence", and the concept "AIoT", which is all the rage in 2019, is a combination of the two.
It is widely believed that the Internet of Things and artificial intelligence technology will jointly promote human society from "Internet of Everything" to "Intelligent Connection of All Things". For example, Huawei proposed to "build a smart world with interconnection of all things", Xiaomi took "AIoT" as the core strategy, and Internet companies such as BAT also listed similar visions.
As the intersection of the Internet of Things and artificial intelligence, wireless perception reflects this trend and has become a hot spot pursued by academic research and industry.
2. The development of intelligent wireless perception
At present, the development of domestic wireless sensing presents two major characteristics and trends:
1) The perceptual granularity is gradually refined. For example, from the perspective of personnel perception applications, from the early stage of development of personnel intrusion detection, to the passive positioning tracking of personnel, and then to the current gesture recognition, the granularity of perception from the presence of personnel, to the position of personnel, to the activities of various body parts, the granularity is becoming more and more detailed.
2) The trend of landing is becoming more and more obvious, and wireless perception is moving from laboratory theoretical research to daily application of the family. According to Yang Zheng's observation, in recent times, the industry has paid more and more attention to non-sensor perception, and various application platforms based on non-sensor perception are gradually developing, showing the characteristics that various related applications are moving towards "thousands of households".
The application scenarios of intelligent wireless perception are very extensive, covering security, medical treatment, human-computer interaction and even meta-universes. Among them, medical monitoring is Yang Zheng's most optimistic application direction, including non-contact heartbeat, respiratory monitoring, and fall detection.
In particular, fall detection (such as Apple's Apple Watch 4 added this feature): "As the phenomenon of an aging population becomes more and more serious, many elderly people live alone. Routine surveys have found that falls are one of the main causes of injuries to the elderly, and in severe cases, falls can even be life-threatening. Therefore, it is very important for the elderly to study a reliable fall detection mechanism."
Intelligent perception scholars such as Yang Zheng are very optimistic about the application prospects of this direction. However, Yang Zheng also clearly pointed out that there are still three major challenges in the current development of intelligent wireless perception in China, namely the annihilation of effective features, the crude recognition model, and the lack of data sets.
Note: Three challenges for non-sensor perception
From the feature part, since the wireless signal contains information about the channel propagation space, most of the existing signal features extracted by the work depend on the specific environment of the system deployment. Therefore, different usage environments, different users, and even different locations and different orientations of the same user will reduce the accuracy of perception. For new scenarios, most of the work needs to re-collect data for training, which makes the universality of wireless perception poor, the cost of learning and training is high, and the generalization ability of the system is greatly affected.
From the model part, most of the existing models are based on the perfect assumption of wireless signal propagation and hardware conditions, but in the actual deployment scenario, the actual propagation of wireless signals includes diffraction, scattering, non-specular reflection and other situations, commercial network cards usually contain a lot of noise, and the characteristics between different network cards have greater differences. The lack of systematic modeling of wireless signal propagation mode and error elimination makes there still be a certain difference between the operation results of the wireless perception system and the theoretical expectations.
From the perspective of data sets, due to the time-consuming and laborious collection of data sets in wireless perception, there are differences in collection hardware, scenarios and other aspects of the data collected by different teams, which also hinders the disclosure and sharing of basic data sets in this field, and the amount of data studied is insufficient and the application scenarios are small, which affects the technical analysis and progress in this field.
There is still a long way to go to solve the above problems.
3. Yang Zheng's research journey
As one of the few young scholars in China who took the lead in studying the combination of wireless perception, Yang Zheng's research journey began in 2012. At that time, he had just obtained a ph.D. in computer science from the Hong Kong University of Science and Technology for less than two years, and returned to teach at Tsinghua University, where he was a "green pepper" with little seniority.
Since his Ph.D., Yang Zheng has been engaged in research on the Internet of Things, including intelligent perception, industrial Internet, edge computing and blockchain. At that time, his doctoral supervisor was Liu Yunhao, a pioneering scholar of the domestic Internet of Things. Liu Yunhao is the first Chinese winner of the ACM and IEEE Double Fellowew and ACM Chairman Awards, and in the summer of 2020, he replied to 2,000 questions about artificial intelligence of Tsinghua students in 10,000 words, which was "named" by Tsinghua officials and became popular on the Internet.
Photo note: During his ph.D. in 2007, Yang Zheng (left) took a group photo with his doctoral supervisor Liu Yunhao (right).
"When I first started my PhD career (2006), one of the hot topics of research in the industry was actually wireless sensor networks." Yang Zheng recalled to AI Technology Reviews.
Wireless sensor networks can be understood as the prototype of today's Internet of Things, which use wireless communication technologies (such as Bluetooth, ZigBee, etc.) to achieve information interaction between sensor nodes, but the task of perception needs to be completed by various types of dedicated sensor devices. Obviously, this necessarily limits the flexibility of wireless sensor networks.
"Deploying a wireless sensor network is a particularly time-consuming and laborious process, not only to ensure the perception function of the sensor nodes themselves, but also to ensure the communication performance between them point-to-point. So during my phD studies, every time I did an experiment, I had to put a lot of effort into just deploying the experimental equipment."
At that time, he hoped to explore a more ubiquitous and universal way of perception to reduce the labor cost and equipment cost of system deployment.
Around 2010, he began to focus on some work that used wireless signals for positioning. This "non-sensor perception" mode does not rely on any dedicated sensing equipment, only uses transceiver equipment, and can locate the equipment indoors by analyzing the signal characteristics. Compared with the wireless sensor network, the deployment cost is lower, the universality is stronger, and it is easier to promote, which attracts Yang Zheng to continue his research.
Photo note: When Yang Zheng first arrived at Tsinghua to teach
Since 2012, Yang Zheng and his team have gradually realized a series of work based on wireless signal positioning, tracking, navigation, and then to personnel gesture recognition, heartbeat and breath detection, etc. through unremitting efforts.
In response to the above three challenges (features, algorithms and data), Yang Zheng's team proposed its own solutions in the Widar series (especially Widar 3.0).
According to Yang Zheng, Widar is the abbreviation of Wi-Fi Radar, and the Widar series of work gives commercial Wi-Fi devices a strong sense of the environment on the basis of ensuring the original communication function of Wi-Fi. From the first generation of multi-device personnel positioning, to the second generation of single-device personnel tracking, and then to the third generation of gesture recognition, the sensitivity of the Widar series of work to the environment is also constantly refined, fully exploring the technical feasibility of communication perception integration.
For wireless signal characteristics, Yang Zheng's team's research ideas focus on two points: multi-dimensional feature fusion and extraction of environmentally independent signal features.
"Multidimensional feature fusion refers to the comprehensive use of signal attenuation, arrival angle (AoA), time-of-flight (ToF), Doppler frequency bias (DFS) and other signal characteristics to more accurately complete the task of wireless positioning and perception."
The environment-independent signal features are to model a feature that does not depend on environmental characteristics, strong generalization, and robustness, such as the speed spectrum (BVP) under the human coordinate system we proposed in WiDar3.0, which can use human body coordinates as a benchmark to more comprehensively and accurately describe the action characteristics of different environments and downward human bodies, so as to achieve high-precision gesture recognition tasks suitable for various scenarios."
Widar3.0 Address: http://tns.thss.tsinghua.edu.cn/~yangzheng/papers/Zheng-Widar3-MobiSys2019.pdf
In terms of models, Yang Zheng mainly borrowed the method of deep neural network (DNN) model in Widar3.0.
"The rise of the wave of deep learning has also brought great inspiration to the field of wireless perception. In recent years, many efforts have been made to input wireless signals into some existing classification models in the form of matrices or tensors to try to obtain better results, but there is no more reasonable design of the model for the unique characteristics of wireless signals."
Therefore, Yang Zheng and his team proposed a convolutional and circular structure in Widar 3.0 that has been designed to fully explore the spatial and temporal dimension characteristics of wireless.
At present, their team is also working on combining time-frequency transformation and classical signal sampling principles to design a feature extraction and discriminant network suitable for wireless perception, which can more effectively excavate the implicit information in wireless signals, thereby simplifying the training and learning process and breaking through the bottleneck of recognition accuracy. This work is currently being submitted.
As the third generation of the Widar series, The main contribution of Widar 3.0 is to achieve environment-independent gesture recognition.
Specifically, the previous identification systems based on wireless signals can only maintain a high accuracy rate in specific environments where training data is collected, and lack the possibility of use in other scenarios, which is due to the low quality of features and poor model design of the existing schemes, which will lead to certain generalization problems. Combined with the observation of the directionality of human movements, Yang Zheng's team proposed the velocity spectrum (BVP) under the human coordinate system, which solved the domain adaptation problem of the system at the level of features.
In addition, in Widar3.0, the network structure designed based on the principle of signal time correlation and time-frequency transformation also helps to improve the training speed and accuracy of the model. In short, Widar3.0 effectively improves the design of intelligent wireless perception system at the two levels of features and models.
Note: Widar dataset (gesture recognition)
Finally, there is the dataset. Yang Zheng believes that the vigorous development of computer vision and deep learning in recent years is inseparable from many publicly available massive data sets such as ImageNet. Therefore, they also want to build a dataset specifically for intelligent wireless perception. Since the introduction of Widar 1.0 in 2017, all the original CSI data and signal characteristic data in the subsequent 2.0 and 3.0 have been open sourced. The data covered 260,000 sets of actions collected in 75 scenarios, with a total collection time of 144 hours and a total data scale of about 325 GB. At present, the dataset expands new data such as fall detection on the basis of gesture recognition.
Widar 3.0 dataset address: http://tns.thss.tsinghua.edu.cn/widar3.0
"We hope that through this way, we can support researchers to make more innovative work in the field of wireless perception and contribute a little to the development of the field of wireless perception." Yang Zheng said.
Building a dataset is a big project. Yang Zheng soberly realized that in the era of big data, it is impossible to support a huge perception application by manually collecting data by relying on his own team. Therefore, researchers still need to mobilize more forces and use more technical means to expand the perceptual data set.
In this regard, Yang Zheng has "three axes":
First, based on the statistical electromagnetic field model and the ray tracing model, they developed a wireless signal simulator at the physical layer level, using the simulation data as a supplement to the real data, in order to solve the problem of insufficient training data and difficult data acquisition in specific environments.
On this basis, they are also trying to use the architecture of generative adversarial network (GAN), using the collected real data to train a discriminator, and develop a wireless signal generator based on the above simulator, through adversarial learning, generate "false and real" perception data to effectively expand the amount of data.
Finally, they are also preparing to refer to the collection methods in areas such as data mining, and adopt strategies such as "crowdsourcing" to encourage users to use network devices in their homes to actually collect a large amount of wireless signal data for research. In the process, users can obtain a certain amount of funds as an incentive.
But the power of the individual is always limited. Yang Zheng called on everyone to actively participate in the open source work of the perception data set and promote the scale expansion of the perception data set through open sharing.
4. The "marriage" between artificial intelligence and perception
In the Internet of Things, Yang Zheng's two major research directions are intelligent perception and industrial interconnection. He explains:
"Intelligent perception is to achieve ubiquitous perception and ubiquitous intelligence by analyzing the characteristics of signals such as vision, radio frequency, sound and light, and applying artificial intelligence technology to explore the state or events of the physical world." For industrial production scenarios, Industrial Interconnection proposes an industrial network architecture of control as a service, develops a time-sensitive network with determinism and low latency, and combines the information (IT) network with the production (OT) network to realize the servitization of control tasks and support future intelligent flexible production."
So, in intelligent wireless perception, what role can algorithms based on machine learning, deep learning, and adversarial learning play? How do researchers combine AI algorithms with wireless sensing?
"It's a particularly good question, and it's something our team has been thinking about in recent years. First of all, wireless perception expands the "field of vision" of artificial intelligence, adding one more dimension of perception information." Yang Zheng said.
Over the past decade, Yang Zheng and his team have tried many machine learning models to help build smarter, robust wireless perception systems.
For example, in wiDance, which won the CHI 2017 Best Paper Honorable Mention award, Yang Zheng tried to use the classic hidden Markov model (HMM); in WiDar3.0 released in 2019, they designed a high-precision motion recognition network model based on convolutional neural networks (CNNs) and long-short time memory networks (LSTM); in addition, they also tried to introduce domains in the work of wireless fingerprint positioning adversarial) and a series of other mechanisms to ensure the robustness of the system in different environments, to achieve environment-independent positioning and perception.
WiDance paper link: http://tns.thss.tsinghua.edu.cn/~yangzheng/papers/Qian-WiDance-CHI2017.pdf
"So what I've always felt is that perception and intelligence should be one." Various intelligent algorithms, including machine learning, are responsible for analyzing data and giving results, while various types of sensing devices in the Internet of Things (including both visual perception and wireless perception) provide basic data support for intelligent algorithms, which obtain data in real time from the physical world and react back to the physical world after processing." Yang Zheng commented on AI technology reviews.
On the one hand, wireless perception "learns" from the application of artificial intelligence in vision; on the other hand, wireless perception also plays a certain role in inspiring the design of neural networks. For example, Yang Zheng mentioned that in wireless perception, some experience for signal processing can also guide us to design more efficient and reasonable neural networks:
"Radio frequency signals have unique characteristics that differ from visual signals, and these characteristics are very beneficial for specific perceptual recognition tasks. How to dig deeper into the unique characteristics of these RF signals is also a special consideration when designing artificial intelligence models."
Due to the close relationship between perception and intelligence, combining artificial intelligence (especially deep learning) with research in the field of the Internet of Things has become a mainstream research approach. In recent years, wireless perception papers published at top conferences have taken up "half of the world" of work combining deep learning techniques.
Despite being in the IoT circle, Yang Zheng has always paid attention to the recent progress of artificial intelligence (especially deep learning), looking for commonalities between them and his work, and has repeatedly found new scientific research opportunities.
Yang Zheng said frankly: "Of course, there are challenges. Most models were first proposed for use in the field of computer vision or natural language processing, so the flexible migration of these solutions to our own research fields requires not only a deep understanding of our own research areas, but also a thorough understanding of various neural network design philosophies to avoid "rigidity"."
"The bigger opportunity is that wireless perception, as a way of perception outside of vision, has not yet received attention from the direction of artificial intelligence."
In the same class of applications, wireless perception sometimes performs better than vision.
Take fall detection, for example. Common fall detection technologies include computer vision, wearable sensors, and dedicated radar hardware. Yang Zheng pointed out that these technologies have certain flaws: computer vision is easy to involve user privacy, and usually can only work in good lighting conditions; wearable sensors need to wear specific devices, which is very inconvenient for the elderly to use; and large-scale deployment of specific hardware requires higher costs.
In such scenarios, non-sensor-based perceptions exhibit their unique advantages over technologies such as computer vision: Wi-Fi perception, for example, is not limited by the above conditions, providing a new idea for fall detection. At present, almost every household has deployed Wi-Fi devices, without the need to deploy new hardware devices; Wi-Fi-based perception does not require users to wear specific devices, and can work normally in lightless conditions; the wireless signal information it collects will not interfere with the privacy of users.
Asked whether the future development of intelligent wireless sensing technology depends more on artificial intelligence or the advancement of communication technologies such as 4G/5G, Yang Zheng's answer is that "both types of technologies are very important, and they will affect the development of intelligent wireless sensing technology in the future in two different ways."
Specifically, the support of a new generation of wireless communication technology for new technologies such as MIMO and Beamforming objectively provides us with more antennas and more fine-grained angle detection methods, while new communication technologies generally support higher communication rates through a wider frequency band, which also provides a most basic support for the improvement of distance resolution.
The artificial intelligence technology represented by deep learning fully excavates more hidden features in wireless signals, and also has a great improvement in the perception ability of the system.
Therefore, the evolution of communication technologies such as 4G/5G provides more effective information, while the evolution of artificial intelligence technology provides more and more powerful means for the mining and processing of information, and the progress of the two has jointly promoted the prosperity of the field of intelligent wireless perception.
5, the future of wireless perception lies in "generalization"
In 2021, based on his contributions to wireless perception and positioning, Yang Zheng was elected as the 2022 IEEE Fellow, the youngest Chinese scholar selected in the same year, at the age of 38. Leifeng Network
In terms of intelligent perception, Yang Zheng's scientific research plan focuses on two major blocks, one is the design of neural network algorithms based on wireless signals, and the other is to promote the commercialization of wireless perception. Leifeng Network
Although AI has played a significant role in the study of wireless perception, it is also extremely difficult to migrate AI models geared toward machine vision to wireless perception.
Most of the current deep learning models are models customized based on image data and language data, such as CNNs and RNNs. However, the RF signal in wireless perception has its own characteristics compared with images and language: it contains multi-dimensional information such as time, space, frequency, etc., and the data is mostly plural types.
It is precisely because of the difference between RF data and existing network models that the current deep learning model still does not play its greatest role in the field of wireless perception. Therefore, Yang Zheng's idea is to design a deep network dedicated to wireless signals for the characteristics of wireless signals, so that wireless signals and depth models can achieve deeper coupling, so as to make full use of artificial intelligence tools.
In addition, most of the current wireless sensing technology is still in the experimental stage, and there are not many technologies that have truly realized commercialization. At present, the industry's attention to medical health is rising, and the potential of wireless sensing to be applied to fall detection has also entered the eyes of the three industries of industry, academia and research. Yang Zheng and his team also hope to contribute to this, designing a reliable fall detection system to improve the robustness and generalization ability of the system.
"I personally believe that the key to the further development of intelligent wireless perception lies in its scene generalization ability. More generally speaking, can the wireless perception system we designed really be directly applied to thousands of households, as envisioned, support all kinds of wireless devices, and adapt to different complex environments? At present, we seem to face some challenges."
Yang Zheng analyzed that in order to improve the generalization of wireless perception technology, researchers still need to solve the following problems:
First, it is not yet known whether the existing CSI model assumptions fit perfectly into the real-world scenario. For many years, most researchers have based on ray tracing models to see walls, obstacles, etc. as an ideal reflective surface, and electromagnetic wave signals will be perfectly reflected by the wall. Although this kind of hypothesis has some truth, in the actual complex indoor space, wireless signals tend to scatter rather than specular reflection, in addition, the transmission and diffraction effects of wireless signals are ignored to varying degrees. So, in a sense, the limit of the accuracy of wireless perception comes from the assumption of being too perfect. A more realistic, general-purpose model of signal propagation needs to be built.
On the other hand, some researchers are accustomed to researching and experimenting with highly flexible software-defined radio (SDR) devices, however, when the perception system is landed, users can only use commercial network cards. If the practical factors such as nonlinearity error, carrier frequency deviation, and packet detection delay of commercial network cards are not solved, even the best perception system design will become a "castle in the air". In addition, the error mode and error coefficient of different network card models are also very different, so for different types of wireless devices, we need to develop intelligent and automated error correction and elimination strategies to ensure that the operating results of the intelligent perception system are consistent with our expectations.
Most importantly, researchers often have to collect data for training and testing in a limited number of indoor environments when conducting experiments. However, the complexity and diversity of the actual use environment often greatly exceeds that of the training environment. This is also why Yang Zheng emphasizes that research should enhance the generalization of the system from multiple levels such as features, models, and data: at the feature level, it is possible to design robust features that the environment has no dependencies on; at the model level, we can try to use domain adaptation strategies and learn from the idea of domain adversarial networks to complete the rapid migration of multi-class scenarios; at the data level, we must find ways to expand the diversity of data sets.
"However, there are already many researchers who are aware of these problems and have proposed many feasible solutions, so I still have full confidence in the development of our intelligent wireless perception field, and believe that in the near future, we will be able to witness the landing and application of intelligent wireless sensing systems." Yang Zheng laughed. Leifeng Network
Finally, Mr. Yang Zheng is recruiting postdoctoral and doctoral students, and students who are interested in the Internet of Things can send an email to Mr. Yang Zheng: [email protected].
Reference Links:
1.http://tns.thss.tsinghua.edu.cn/~yangzheng/
2.https://scholar.google.com/citations?user=ExRe-64AAAAJ
3.https://www.nwnu.edu.cn/2020/0708/c3842a145368/page.htm
4.https://cs.bit.edu.cn/xyxw/fc530c4af4c84ef8abb128c59966b6f1.htm
5.https://baijiahao.baidu.com/s?id=1658509271522706294&wfr=spider&for=pc