Machine Hearts released
Machine Heart Editorial Department
BeVDet, the next-generation pure visual autonomous driving 3D object detection framework of Jianzhi Robot, provides more possibilities for solving key problems such as visual radar, 4D perception, and real-time local maps in vision-based autonomous driving solutions.
Recently, on the authoritative evaluation set of autonomous driving nuScenes, Jianzhi Robot won the world's first result in pure visual 3D object detection with an absolute advantage by virtue of the proposed new paradigm of pure visual autonomous driving 3D perception BEVDet. BEVDet is the first public because of the high performance, scalability and practicality of the BEV space 3D perception paradigm, BEVDet as the core of the series of technologies will hopefully solve the vision of the main automatic driving solution in the vision radar, 4D perception, real-time local map and other key problems, the future will be applied to the intelligent robot with visual radar as the core of the high-level automatic driving and other products and solutions, for the large-scale mass production of automatic driving to play a key role.
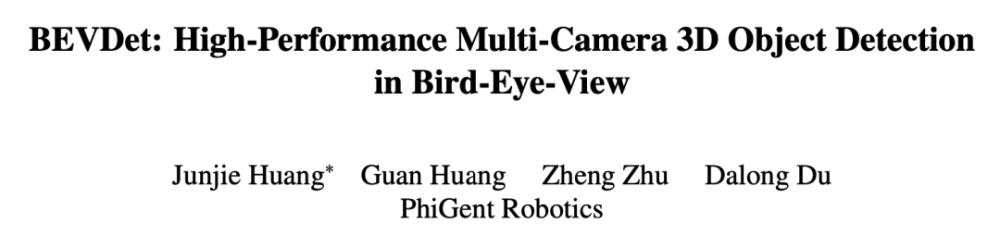
BEVDet Technical Report Link: https://arxiv.org/abs/2112.11790
The nuScenes dataset is one of the most widely used public datasets in the field of autonomous driving, and it is also the most authoritative set of pure visual 3D object detection and evaluation for autonomous driving. In terms of sensors, nuScenes is configured with a total of 6 cameras, 1 LiDAR, 5 RADAR, it is worth noting that unlike kitti and Waymo, which only provide a partial viewing angle, nuScenes provides a 360-degree camera field of view, which can fully perceive the surrounding environment. In terms of data, nuScenes provides rich labeling information including 2D and 3D object annotation, point cloud segmentation, high-precision maps, etc., including 1000 scenes, 1.4 million frames of images, 390,000 frames of lidar point cloud data, 23 object categories, and 1.4 million 3D label boxes, and the data scale and difficulty far exceed the automatic driving dataset KITTI. Previous manufacturers participating in nuScenes pure vision 3D object detection and evaluation include Toyota Research Institute (TRI), Huawei, Ideal Automobile, SenseTime, MIT, Tsinghua University, Hong Kong Chinese University, CMU, University of California, Berkeley and other well-known enterprises and research institutions at home and abroad.
Figure 1: BEVDet proposed by JianZhi Robot topped the list with absolute advantage in the pure visual 3D object detection track (including two evaluation modes without additional data and with additional data)
High-level autonomous driving requires constant perception of the surrounding environment for decision planning, and object detection in 3D space based on pure visual input is one of the most challenging tasks. The goal of 3D space perception by 2D images is to use low-dimensional inputs to predict high-dimensional information, and the lack of dimensions makes the task much more difficult than 2D object detection, and a reasonable paradigm needs to be designed to make full use of input image information to model and reason about high-dimensional information. At present, the industry is based on a 3D perception framework based on pure vision, mainly in the image space for object detection. Such paradigms not only rely on extremely high computing power resources, but also cannot be reasoned in parallel with tasks such as semantic segmentation, and their scalability is poor.
In response to this problem, Jianzhi Robot proposed the next generation of pure visual autonomous driving 3D object detection framework BEVDet. BEVDet follows the concept of modular design and contains the following four modules with a clear division of labor: the image coding module is used to extract high-latitude features in the two-dimensional image space; the perspective transformation module is used to convert the features of the image space to the characteristics of the bird-eye-view (BEV) space; the coding module of the bird's-eye perspective is used to further extract the features under the bird's-eye perspective; and a three-dimensional target prediction module (Head) is used to locate and scale the three-dimensional target in the bird's-eye perspective space , predictions for orientation, speed, and category. BEVDet concisely solves the problem of 3D object detection for pure visual autonomous driving through the above four modules.
Figure 2: BEVDet overall framework
The final performance also fully proves the effectiveness of the algorithm, and on the authoritative dataset nuScenes for autonomous driving, BEVDet has absolute advantages in indicators such as calculation volume and accuracy. Compared with the previous algorithm, BEVDet can achieve similar accuracy indicators through a smaller 1/8 input resolution and a lower 1/4 amount of computation. With inputs of similar resolution, BEVDet has a clear accuracy advantage. In addition, BEVDet has shown performance beyond the existing paradigm in terms of positioning, scale, and direction of the predicted target.
Figure 3: The BEVDet proposed by Jianzhi Robot combines high performance and low computing power requirements on the public test set of pure visual 3D object detection
From the perspective of the development of autonomous driving technology, BEVDet has the following advantages:
The BEVDet framework has strong extensibility, and the intelligent robot is expanding based on BEVDet to achieve key modules for autonomous driving such as visual radar, 4D perception, and real-time local map;
BEVDet builds a view-transformer based on the camera model, which can effectively reduce the learning difficulty of the visual transformation module, compared with the attention-based-view-transformer used by Tesla without prior, this solution can greatly reduce the data demand of the model, so that the model has stronger generalization performance in the case of limited data volume;
BEVDet uses lower computing power to achieve the same or better algorithm effect, which will help improve the computing power utilization efficiency of the automatic driving system.
Figure 4: The Forensic Robot is based on the BEVDet real-car vision 3D perception effect
The current development of autonomous driving technology has entered the second half, on the one hand, it is necessary to solve key problems (imaging problems, 3D problems) to promote the improvement of autonomous driving level, on the other hand, it is necessary to build a better paradigm to make full use of large-scale data and continuously upgrade and iterate.
With the goal of "building robot sensor computing and intelligent brain based on soft and hard collaborative optimization", JianZhi Robot focuses on the research and development of autonomous driving sensor computing and next-generation autonomous driving solutions. At present, a full-stack autonomous driving R&D team of more than 100 people covering algorithms, computing power, software and hardware has been established, and the core members are from domestic first-class AI algorithms, computing power design and automatic driving companies. Based on the sensor input based on vision, through the sensor calculation mode of camera + algorithm + computing power, we will create a standard product of visual radar, and build a high-level automatic driving solution with visual radar as the core.