為什麼要寫這篇文章?
Flink出來已經好幾年了,現在release版本已經釋出到1.10.0(截止2020-05-05),統一了批處理和流處理,很多大公司也都用到生實際務中,跑得也很high。這些大家都知道,但是當我開始考慮怎麼在工作中落地flink的時候,我不知道怎麼入手。公司比較小,目前沒有實時計算,但是etl任務跑得比較慢,效率上有些跟不上。我的思路是想先試着用Flink來處理一些離線任務,看看能不能提升效率,同時為落地實時計算做準備。全網找了半天資料,文章倒是很多,包括一些付費資源,大部分的執行個體代碼都跑不通,真的是跑不通。當然有部分原因是因為我對flink了解太少,但是完整的跑通除了word count之外的代碼不應該是一件比較麻煩的事。
功能說明
1.生成json格式資料寫入kafka topic1
2.消費topic1中的消息,寫入topic2
目的很簡單,如果要落地到具體業務免不了需要做多次的資料處理,Flink雖說是可以做批處理,但是支援得最好的還是流資料,确切的說是kafka的資料,跑通了這個流程,實際上Flink的落地就隻差業務邏輯了,現在有Flink SQL,實作業務邏輯也是分分鐘的事。
代碼
其實隻有4個檔案
├── flink-learn-kafka-sink.iml
├── pom.xml
└── src
├── main
│ ├── java
│ │ └── org
│ │ └── apache
│ │ └── flink
│ │ └── learn
│ │ ├── Sink2Kafka.java
│ │ ├── model
│ │ │ └── FamilyMemberTemperatureRecord.java
│ │ └── utils
│ │ ├── GsonUtil.java
│ │ └── KafkaGenDataUtil.java
│ └── resources
└── test
└── java
複制
pom依賴
<properties>
<project.build.sourceEncoding>UTF-8</project.build.sourceEncoding>
<flink.version>1.10.0</flink.version>
<java.version>1.8</java.version>
<scala.binary.version>2.11</scala.binary.version>
<maven.compiler.source>${java.version}</maven.compiler.source>
<maven.compiler.target>${java.version}</maven.compiler.target>
</properties>
<dependencies>
<!-- json 處理 -->
<dependency>
<groupId>com.google.code.gson</groupId>
<artifactId>gson</artifactId>
<version>2.8.5</version>
</dependency>
<!-- kafka連接配接器 -->
<dependency>
<groupId>org.apache.flink</groupId>
<artifactId>flink-connector-kafka-0.11_${scala.binary.version}</artifactId>
<version>${flink.version}</version>
</dependency>
<!-- kafka 用戶端 -->
<dependency>
<groupId>org.apache.kafka</groupId>
<artifactId>kafka-clients</artifactId>
<version>0.11.0.2</version>
</dependency>
</dependencies>
複制
model
新冠肺炎影響身邊每一個人,舉了一個測體溫記錄測例子
package org.apache.flink.learn.model;
public class FamilyMemberTemperatureRecord {
private int id; // 測量次數
private String name; // 姓名
private String temperature; // 體溫
private String measureTime; // 測量時間
public FamilyMemberTemperatureRecord(int id, String name, String temperature, String measureTime) {
this.id = id;
this.name = name;
this.temperature = temperature;
this.measureTime = measureTime;
}
public int getId() {
return id;
}
public void setId(int id) {
this.id = id;
}
public String getName() {
return name;
}
public void setName(String name) {
this.name = name;
}
public String getTemperature() {
return temperature;
}
public void setTemperature(String temperature) {
this.temperature = temperature;
}
public String getMeasureTime() {
return measureTime;
}
public void setMeasureTime(String measureTime) {
this.measureTime = measureTime;
}
}
複制
json工具類
将對象解析為json格式的資料發給kafka
package org.apache.flink.learn.utils;
import com.google.gson.Gson;
import java.nio.charset.Charset;
/**
* Desc: json工具類
* Created by suddenly on 2020-05-05
*/
public class GsonUtil {
private final static Gson gson = new Gson();
public static <T> T fromJson(String value, Class<T> type) {
return gson.fromJson(value, type);
}
public static String toJson(Object value) {
return gson.toJson(value);
}
public static byte[] toJSONBytes(Object value) {
return gson.toJson(value).getBytes(Charset.forName("UTF-8"));
}
}
複制
資料生成工具類
package org.apache.flink.learn.utils;
import org.apache.flink.learn.model.FamilyMemberTemperatureRecord;
import org.apache.kafka.clients.producer.Producer;
import org.apache.kafka.clients.producer.ProducerRecord;
import org.apache.kafka.clients.producer.KafkaProducer;
import org.apache.commons.lang3.RandomUtils;
import java.text.SimpleDateFormat;
import java.util.Date;
import java.util.Properties;
/**
* Desc: 生成資料,寫到kafka中
* Created by suddenly on 2020-05-05
*/
public class KafkaGenDataUtil {
private static final String broker_list = "localhost:9092";
private static final String topic = "tempeature-source"; // 資料源topic
public static void genDataToKafka() throws InterruptedException {
Properties props = new Properties();
props.put("bootstrap.servers", broker_list);
props.put("key.serializer", "org.apache.kafka.common.serialization.StringSerializer");
props.put("value.serializer", "org.apache.kafka.common.serialization.StringSerializer");
Producer<String, String> producer = new KafkaProducer<>(props);
try {
for (int i = 1; i <= 100; i++) {
Date currentTime = new Date();
SimpleDateFormat formatter = new SimpleDateFormat("yyyy-MM-dd HH:mm:ss");
String dateString = formatter.format(currentTime); // 測量時間
Double body_tempeature = (int)(RandomUtils.nextDouble(36.0,38.5)*10)/10.0; // 體溫
FamilyMemberTemperatureRecord patient = new FamilyMemberTemperatureRecord(i, "suddenly", String.valueOf(body_tempeature), dateString);
ProducerRecord record = new ProducerRecord<String, String>(topic, null, null, GsonUtil.toJson(patient));
producer.send(record);
System.out.println("記錄體溫: " + GsonUtil.toJson(patient));
Thread.sleep(3 * 1000);
}
}catch (Exception e){
}
producer.flush();
}
public static void main(String[] args) throws InterruptedException {
genDataToKafka();
}
}
複制
處理代碼
package org.apache.flink.learn;
import org.apache.flink.api.common.serialization.SimpleStringSchema;
import org.apache.flink.streaming.api.datastream.DataStreamSource;
import org.apache.flink.streaming.api.environment.StreamExecutionEnvironment;
import org.apache.flink.streaming.connectors.kafka.FlinkKafkaConsumer011;
import org.apache.flink.streaming.connectors.kafka.FlinkKafkaProducer011;
import java.util.Properties;
/**
* Desc: 從kafka中讀資料,寫到另一個kafka topic中
* Created by suddenly on 2020-05-05
*/
public class Sink2Kafka {
private static final String SOURCE_TOPIC = "tempeature-source"; // 資料源topic,從這裡讀資料
private static final String SINK_TOPIC = "tempeature-sink"; // 什麼都不做,資料讀出來之後直接寫到這個目标topic
public static void main(String[] args) throws Exception {
final StreamExecutionEnvironment env = StreamExecutionEnvironment.getExecutionEnvironment();
Properties props = new Properties();
props.put("bootstrap.servers", "localhost:9092");
props.put("zookeeper.connect", "localhost:2181");
props.put("group.id", "tempeature-measure-group"); // 這個随便起個名,沒具體研究有什麼用,我也是初學,先不用太在意這些細節
props.put("key.deserializer", "org.apache.kafka.common.serialization.StringDeserializer");
props.put("value.deserializer", "org.apache.kafka.common.serialization.StringDeserializer");
props.put("auto.offset.reset", "latest");
// 從source讀資料
DataStreamSource<String> student = env.addSource(new FlinkKafkaConsumer011<>(
SOURCE_TOPIC,
new SimpleStringSchema(),
props)).setParallelism(1);
student.print();
Properties properties = new Properties();
properties.setProperty("bootstrap.servers", "localhost:9092");
properties.setProperty("zookeeper.connect", "localhost:2181");
properties.setProperty("group.id", "tempeature-measure-group");
// 寫到sink裡
student.addSink(new FlinkKafkaProducer011<>(
"localhost:9092",
SINK_TOPIC,
new SimpleStringSchema()
)).name("flink-connectors-kafka")
.setParallelism(5);
env.execute("flink learning connectors kafka");
}
}
複制
運作效果
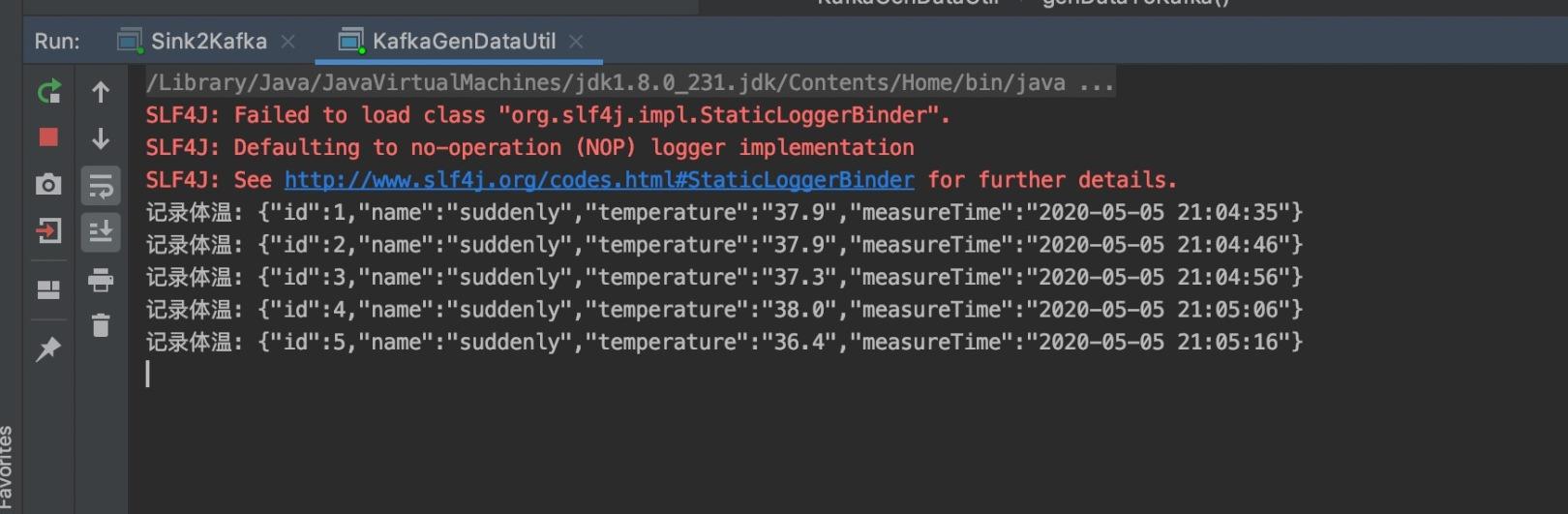
生成資料
消費資料
檢視kafka source和sink topic中的資料
到此,我們實作了生成資料寫到kafka,再把kafka的資料消費後,發到另一個kafka中。
擴充
思考一下,上面的處理過程怎麼用到離線業務中
1.把資料生成部分換成離線業務的資料源
2.把轉發部分的邏輯改成資料清洗邏輯,離線任務就變成準實時任務了(比如原來按天排程的任務,可以先改成按小時讀資料,資料延時就從24小時變成1小時了,進步還是不小的)
3.如果未來離線要改為實時,實時資料肯定也是走消息隊列,假設就是kafka,那生成的源資料直接打到data source中就可以了,處理邏輯基本不需要作修改
怎麼運作
1.kafka肯定是要安裝的
2.上面的例子直接在idea中運作的,代碼copy下就可以,如果報錯的話,需要把flink-dist的包添加到idea的依賴裡,如果你也是mac,/usr目錄被隐藏了,添加目錄的時候選擇Macintosh HD,再按commond + shift + .就能顯示隐藏目錄了
idea添加flink基礎依賴