The full text is 4423 words in total, and the estimated learning time is 12 minutes
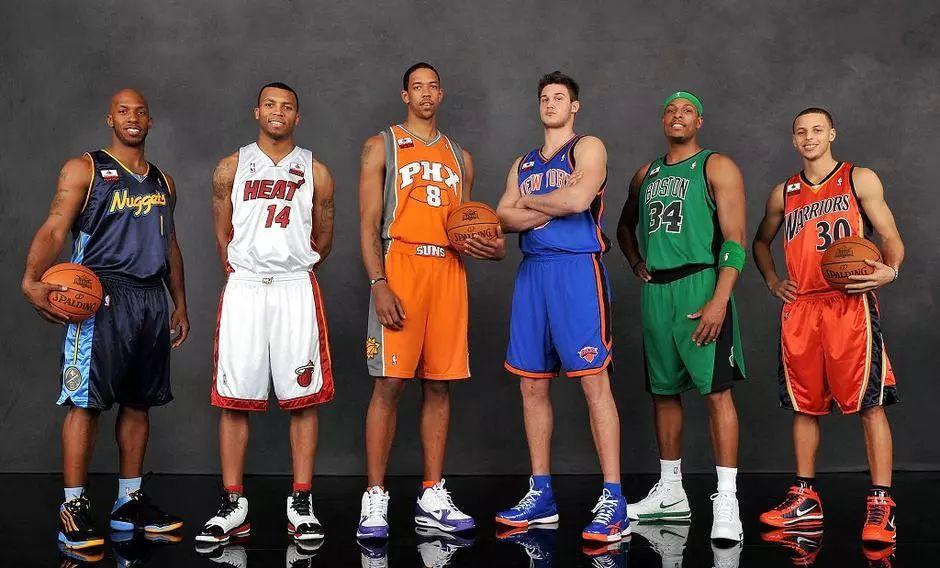
Source: Tuxi
I love basketball. I like to play basketball, watch basketball, talk about basketball. Sometimes I talk to my friends about topics like "If Kobe and LeBron single out who would win?" I needed to use this machine learning project to organically combine my two passions, basketball and data science.
Last summer, the Golden State Warriors transferred Kevin Durant, who won two consecutive NBA Finals MVP (Most Valuable Player Award), to bring in D'Angelo Russell. Sports analysts began speculating about Russell's fit on the Warriors, as follows:
Source: clutchpoints
It also got me thinking: How will D'Angelo Russell adapt to the Warriors' rhythm? Can you use machine learning to classify NBA players and predict how compatible a player will be with a given team?
The research objective of this project is to identify the types of players and their role on the pitch based on historical activity or their use of space.
Data such as points, rebounds, assists, steals, blocks, etc. are not used as features because they rely on data such as time played or number of goals scored (this data also does not appear in the feature). Featuring data such as points, rebounds, assists, steals, blocks, etc. may make the final result closely related to these characteristics, which deviates from the original intention of this project. I will list all the characteristics in detail in the Research Methodology section below.
data
Let's take a look at the data section.
The data is extracted and processed from stats.nba.com by Python and Selenium packets. Most of the features chosen are based on the playing frequency. Many styles of play involve both offensive and defensive positioning. For example, "Offensive Back Singles Rate" refers to the frequency of the player's back-to-back singles in the offensive position; "Defensive Back-to-Body Singles Rate" refers to the frequency of the player's back-to-back singles in the defensive position. For an epitome of these features, see this link: https://stats.nba.com/help/glossary/.
Sample stats: 272 players
The initial dataset contains 531 players. Players who played less than half a season and 1,000 minutes were then removed from the sample. The principle of this is to remove all players who appear precariously. Here is the full sample list of players:
List of players
Select features: 41
The total number of features before screening exceeds 600. Finally, the characteristics describing the landing position and dribbling are selected.
A list of features
Research methods and model selection
Since this project is unsupervised learning, the results it produces require further analysis. I have two goals for model and number of clusters selection:
1. Highlight significant differences between clusters. The number of clusters is too small, and there are too many samples in each cluster to draw stylistic differences between individual players.
2. Avoid too many clusters. If each player is a cluster, the results can only show that each individual is an independent individual, which is of little help to the study.
Model selection: DBSCAN, K-means and Mean Shift
Of the three models, K-Means most effectively achieved the research goals. Both DBSCAN and Mean Shift produce results that contain multiple clusters with only one player.
Number of clusters: 10
I decided to set the number of clusters in multiples of 5 because there are 5 positions on the basketball court. The 10 clusters fit the research approach I envisioned.
Research results
I calculated the average of all the features in each group with the results and ranked each group based on the highest and second highest features. The terms are defined as follows:
Primary features: The average of the listed features is the highest in a group.
Secondary features: The average of the listed features is the second highest in a group.
In addition, the primary characteristics of each group are shown through a bar chart, which is used to compare with other players.
The first group
Steven Curry
Bradley Bill, Buddy Hilde, Stephen Curry, Evan, Trevor Ariza, Kyle Lowry, Joe Ingles, Otto Porter Jr., Bogdan BogdanOvich, Avery Bradley, Tim Hardaway Jr., Jason Tatum, Justis Winslow, Jeremy Lamb, Itovan Moore, Kevin Knox, Kevin Hürth, Bogdan Bognovic, Gary Harris, Brin Forbes, Eric Gordon, Taylor Johnson, Damian Dotson, Torrian Prince, Garrett Temple
Primary feature: Defensive singles shooting percentage
Secondary features: Hand-to-hand defense rate, Defensive shot rate around cover, Defensive around cover rate, Defensive back-to-body singles rate, Fast attack rate, Hand-to-hand offense rate, Offensive shot rate around cover
Defensive long-range shooting frequency
The second group
Carl Anthony Downs, LaMarcus Aldridge, Joel Embiid, Thadde, Thaddeus Young, Blake Griffin, Anthony Davis, Nikola Jokic, Julius Randall, Nikola Vucevic, DeAndre Ayton, Miles Turner, Al Horford, Mark Gasol, Malvin Bagle III, Jalen Jackson Jr., Serge ibaka, Bobby Portis, Ines Kanter, Jonas Vallanciyunas, Robin Lopez, Markieff Morris, Gorgy Jean
Primary features: Offensive back-to-body singles rate, back-to-body singles touch rate
Secondary feature: Offensive rebounding rate adjustment
Offensive back-to-back singles rate
The third group
PJ Tucker, Draymond Green, Malvin Williams, Jay Crowder, Brooke Lopez, Dario Saric, Dwayne Dedmond, Jeff Green, Kelly Orique, Davis Bertans, Mike Muscara, Maxi Krebel, Jared Dudley, Mike Scott, Jonas Jerebke, Anthony Tolliver, Vince Carter
Primary features: Catch shot rate, Offensive set shot rate, No defensive shot rate, Defensive singles rate, Defensive back-to-body singles rate
Secondary characteristics: Defensive fixed-point shooting rate, the number of passes is greater than the number of catches
Catch shot rate
Group IV
Josh Richardson, CJ McCollum, Mike Conley, Jamal Murray, Daron Fox, Trey Young, Sadie Osman, Averid Payton, Chris Dunn, Danny Schroeder, Eric Bledsoe, Malcolm Brogden, Thomas Satoransky, Patrick Beverly, Danny Smith Jr., Emmanuel Mudiel, Fred VanVleet, Ricky Rubio, Shay Gilgis Alexander, Darren Collison, Reggie Collison Jackson, D.J. Augustine, Corey Joseph, Drake White, Ryan Ashtiacno
Primary features: Defensive rebounding distance, Offensive blocking execution rate, Average dribbling with the ball, Uniform offense
Secondary characteristics: Average number of seconds to hold the ball, offensive blocking execution rate, offensive rebounding distance, long dribble shooting rate
Frequency of defensive ball processing
Group V
LeBron James
Judhir Heldi, Paul George, Zaco Lavin, Tobias Harris, Brandon Ingram, Jimmy Butler, Devon Booker, Cowy Leonard, Demar DeRozan, Kemba Walker, Russell Westbrook, Damian Lillard, Andrew Wiggins, Donovan Mitchell, Kyle Irving, Kevin Durant, LeBron James, James Harden, Chris Middleton, Luka Doncic, Colin Sexton, De Angelo Russell, Chris Paul, Rajan Rondo, Jordan Caraxon
Primary features: Long dribble shooting rate, offensive singles rate, offensive blocking execution rate, average number of seconds of touch
Secondary characteristics: average number of dribbles touched balls, frequency of defensive blocking execution. Defensive rebounding probability adjustment, no defensive shooting rate
Average number of dribbles with the ball
Group VI
Nicholas Batum, Lonzo Bauer, Mikaar Bridges, Danny Green, Kelly Uprell Jr., Jonathan Isaac, Terrence Ferguson, Jaylen Brown, Dorian Finney Smith, Kenridge Williams, Josh O'Kerkiki, Demare Carroll, Deandre Bembry, Maurice Harkris, Andre Iguodala, Rodions Kuruz, James Ennis III, Shaquil Harrison, Pat Connaughton, Royce O'Neill, OG Anna Nobi, Tori Craig, Justin Jackson, Bruce Brown, Frank Jackson
Primary features: Fast attack rate, defensive back-to-back singles rate, defensive shot rate
Secondary characteristics: defensive singles shooting rate, offensive fixed-point shooting rate, no defensive shooting rate
Fast attack rate
Group SEVEN
DeAndre Jordan, Monterez Harrell, Bam Adebayo, Jermichael Green, Mason Plumlee, Mitchell Robinson, Zach Collins
Primary characteristics: other offensive tactical probabilities, other offensive probabilities, close-in shooting rates, defensive blocking execution rates, defensive fixed-point shooting rates
Secondary features: Confrontation pitch, Defensive shot rate, Elbow zone touch rate, Offensive air cut rate, Offensive back-to-body singles rate, Paint Zone/Three-Second Zone Touch Rate, Back-to-Body Singles Touch Rate
Close-range confrontation shooting percentage
Group VIII
Giannis Antetokounmpo
Kyle Kuzma, Aaron Gordon, Ben Simmons, Harrison Barnes, Geramy Grant, Pascal Siakam, Giannis Antetokounm, Laurie Markkanen, T.J Warren, Kyle Anderson, Danilo Gallinali, Al Farouk Aminu, Jabari Parker, Noah Vonle, Nemania Belitsa, Wilson Chandler, Miles Bridges, Ronda Hollys Jefferson, Mario Hezonia, James Johnson, Derek Jones Jr
Primary features: Change in defensive rebounding rate, defensive set-point rate, defensive shot rate around cover
Secondary characteristics: defensive singles rate, defensive blocking execution shooting rate, defensive fixed point shooting rate, offensive singles rate
Defensive rebounding probabilities change
Group IX
Klay Thompson, JJ Redick, Justin Holliday, Joe Harris, Reggie Baroque, Wesley Matthews, Terrence Rose, Aaron Crabbe, Kentavios Caldwell Pope, Landry Shamet, Vin Ellington, Marco Belinelli, Dalius Miller, Lanston Galloway, Kyle Korver, Doug McDermott, Tony Snell
Primary features: offensive hand pass rate, offensive shot rate around cover, no defensive shot rate, offensive rebounding distance, defensive hand pass rate, defensive around cover rate
Secondary characteristics: uniform attack, catch rate, defensive rebounding distance
No defensive shooting percentage
Group 10
Steve Adams, Clint Capela, Rudy Gobert, Andre Drummond, John Collins, Willie Cowley Stan, Tristan Thompson, Yusuf Nurkiz, Cody Zeller, Jarrett Allen, Larry Nance II, Wendel Carter II, Demantas Sabotis, Ty Gibson, Drake Favors, Dwight Powell, Javier McKee, Hassan Whiteside, Thomas Bryant, Alex Lane, Kevin Rooney, Ed Davis, Ivica Zubac, Jacob Peltel, Ante Žižić
Primary features: Offensive blocking execution rate, offensive air cut rate, shooting rate, offensive rebounding probability adjustment, number of passes greater than the number of catches, elbow zone touch rate, three-second zone/paint zone touch rate
Secondary characteristics: Near-on shooting percentage, defensive back-to-back singles rate, offensive other probabilities, offensive tactics other probabilities
Three-second zone/paint zone touch rate
The results surprised me. Usually, we think that the top league-wide point guard like Steven Curry will be tied with other star players, but the model used this time puts him in the first group, where most of the players have average ability values. In contrast, the fifth group contains a lot of star players. As ball-handling players, their primary characteristics are: long dribbling shooting rate, offensive singles rate, offensive blocking execution rate, average number of touch seconds.
I'd love to discuss the characteristics of each set of data in detail, but since this is a data science project, I'll turn to data visualization issues below.
Visualization of results
Due to the difficulty of visualizing all 41 dimensions, I used principal component analysis (PCA) to reduce 41 dimensions to 3 dimensions. Readers unfamiliar with principal component analysis can refer to the following definitions:
"Principal component analysis is responsible for finding new series of dimensions (or a set of basic points of view) so that all dimensions appear orthogonal (i.e., linearly independent of each other) and arranged according to the difference in data between them. This means that principal component analysis preserves the more important principles. ”
After integrating the K-means output and the results of principal component analysis dimensionality reduction, three three-dimensional clusters of Plotly are generated, as shown in the following screenshot:
3D charts
Three-dimensional space is more likely to show the differences between the individual clusters, and the chart can also visually show how K-means divides 41 dimensions into 4 clusters.
Conclusions and reflections
Back to the original question: Can D'Angelo Russell work well with Steven Curry? Let's go back to the fifth group.
The Warriors moved out of Kevin Durant and into D'Angelo Russell. Both belong to the fifth group, the ball-handling players group.
So my advice to Warriors coach Steve Kerr is to have Curry and Russell play at the same time. Of course, he must have anticipated this, and there was no need for the model to give him advice. Russell's possession is expected to improve, while Curry will play more of a no-ball player role.
In the future, I hope to analyse the players in each group one by one and look at how well each player performs on the primary and secondary characteristics of the group. Adding analytical content, thinking about how to improve unsatisfactory points, or how to reposition a player's role in the team, will help improve player performance.
I hope that all readers enjoy this article, and I also look forward to your suggestions and comments (manual comparison).
Leave a message like attention
Together, we share the dry goods of AI learning and development
If reprinted, please leave a message in the background and abide by the reprint specifications