As we all know, the advancement of autonomous driving technology is inseparable from large-scale road test data. There are three dimensions for evaluating the quality of road test data, one is the scale of the data, one is the accuracy of the data, and the other is the breadth of the data.
For "when will autonomous driving actually be realized", the industry has been quoting such a set of data. According to research by the RAND Corporation, self-driving cars need to be tested for at least 11 billion miles (17.7 billion kilometers) in a real or virtual environment to prove that self-driving systems are more reliable than human drivers.
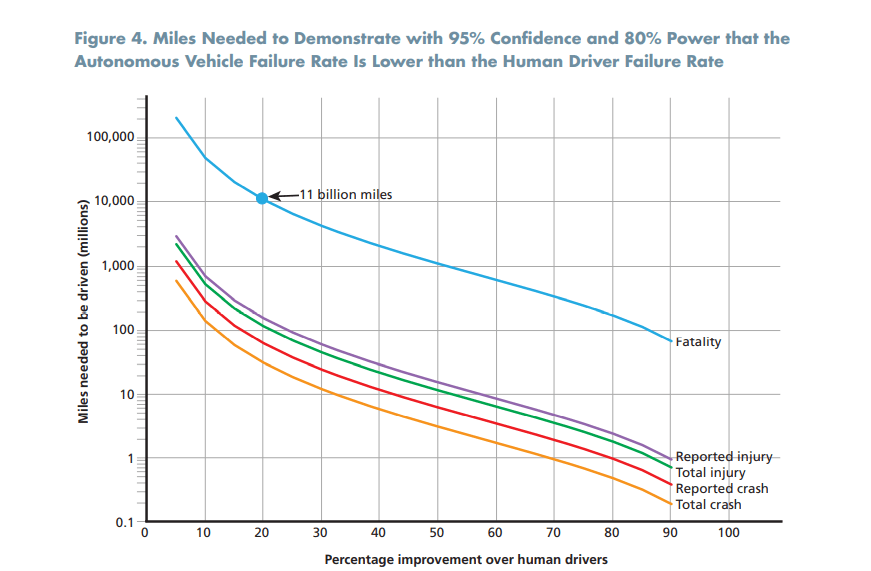
By RAND's own estimates, it would take about 500 years for a fleet of 100 vehicles to be tested 24 hours a day, 365 days a year, 24 hours a day, at an average speed of 28 miles per hour.
Don't say that ordinary people can't wait that long, and investors who believe in long-termism can't survive. So can autonomous driving still be done?
The answer is yes. The solution is also found in three dimensions of road test data.
For the scale of data, since tens or even tens of billions of kilometers are needed, don't just count on dozens of hundreds of test vehicles. Tesla apparently found a way to understand, that is, to mine the gold mine of data from the passenger cars that were mass-produced on the road.
According to Tesla, at the beginning of 2020, the cumulative mileage of Tesla's assisted driving has exceeded 4.8 billion kilometers, and Tesla's self-driving capabilities have also improved significantly. The results of taking the lead big brother have made domestic players who take the progressive mass production assisted driving route see a bright future.
Looking at the accuracy and breadth of the data, this brings us to labeling and training. Automatic driving based on deep learning algorithms inevitably cannot bypass the labeling and training of data, the accuracy of data labeling determines the accuracy of the algorithm, and the breadth of training determines the adaptability and robustness of the algorithm. But at the same time, data labeling and training are the two most expensive "gold-devouring beasts" in the research and development of autonomous driving technology.
That is to say, the maturity of autonomous driving is not only a quantitative problem, but also a quality problem and a cost problem. The solution of the cost problem of labeling and training determines whether the automatic driving technology can enter the daily life of the public as soon as possible.
How expensive is the cost of data labeling? The answer is manual. Otherwise, the industry is ridiculing that "artificial intelligence is how much intelligence there is, how many artificial" is. So, is there a solution? Yes. That is to replace "labor" with "intelligence" and automate labeling. This also confirms the saying that only magic can defeat magic, and it is still AI that relies on AI to solve the problem.
What is the cost of data training? The answer is actual road testing. Cars have to drink oil, people have to rest, and the cost of road testing has almost become a straw that overwhelms Waymo's camel. So, is there a solution? Yes. That's simulation.
On closer examination, the RAND report also mentioned that 17.7 billion kilometers of data can also be obtained through simulation training.
At this point, we know that if we want to achieve automatic driving as soon as possible, we must obtain large-scale, accurate, and wide-ranging good data. To really achieve that, we have to reduce the cost of data labeling and training.
Here's the problem. How to "reduce costs and increase efficiency"?
Here we have to mention the head autopilot companies represented by Tesla and Zhixing. Through these two public solutions, we see the key to the two "gold-devouring beasts" of autonomous driving?
Labeling and Training: The Key to Accelerating Autonomous Driving Technology
Labeling and training, for the importance of automatic driving, we must say a little more.
Based on the first principle of automatic driving, it can be seen that automatic driving is essentially the learning and imitation of human driving, and labeling and training for automatic driving is equivalent to the gradual familiarity and understanding of the road environment from novices to old drivers.
Novices on the road, in addition to excluding the assistance of various electronic products, first need to learn to recognize the road, including all kinds of traffic signs, lane lines; On this basis, it is also necessary to learn to recognize people, including all kinds of people and vehicles involved in transportation.
The labeling work is to let automatic driving learn to recognize the road and recognize people, and under the comprehensive influence of various environments, it can recognize and recognize all the traffic participants in the surrounding area, and provide accurate information reference for algorithm decision-making.
Compared with the labeling, the work of training is very simple and easy to understand. Novices need to experience the baptism of various environments and road conditions, thus summarizing experience and gradually transforming into veterans; The training work will allow the automatic driving to replicate this process to improve generalization.
The only problem is that in the process of human driving, the work of marking and training is very slow, and the driver may be able to grasp the essentials within a year, or it may take several years to figure out the tricks; If autonomous driving is to replicate this process, it will require a lot of cost, and the shorter the time required, the higher the input cost.
Obviously, it is impossible to achieve this goal by relying on human stacking alone, so if automatic driving wants to mature quickly and quickly, it must reduce costs and increase efficiency for labeling and training.
Labeling is the highest cost source for autonomous driving. In the past, a large number of labeling work of autonomous driving enterprises was completed by manpower, and the labeling efficiency was low and the cost was high, and it was difficult to efficiently label massive data. To reduce the cost of labeling, the introduction of automatic labeling capabilities is inevitable.
The other is training. In the past, the training of autonomous driving was carried out in real scenes, but just as humans cannot control nature, the variables of the driving environment are also unattainable by autonomous driving companies.
As a simple example, what if an autonomous driving company wants to train and validate a set of autonomous driving algorithms in winter snow and ice environments suitable for mainland road conditions in the spring?
This unique weather environment is difficult to encounter in the real scene, and even if it is encountered, it is difficult to ensure whether its variables will affect the training effect, and if you can build a simulation system for the algorithm model for training, it will greatly improve the training efficiency.
In a word, the labeling and training cost determines the iteration speed of automatic driving technology, who can effectively reduce the cost of labeling and training, who can achieve the iteration of automatic driving technology faster.
Tesla: Use AI to learn human logic and then replace humans
Like all self-driving companies, Tesla's labeling work was done by human labor at the beginning, but after the rapid expansion of the scale of vehicle driving data, the inability of manual labeling in the face of massive data was also infinitely magnified.
As a result, Tesla has introduced automatic labeling capabilities into autonomous driving technology, and assigned tasks with manual labeling according to their respective advantages, which greatly reduces the cost of manual labeling and improves the overall labeling efficiency.
For static annotates, Tesla will give a three-dimensional point prediction estimate of this scene based on the image information fed back by the camera, first map it to the camera, and then optimize and reconstruct it across time and space dimensions after comparing the results with the real scene.
When other vehicles pass through this scene, the same data will also be fused and optimized by the labeling system, which not only improves the accuracy, but also improves the freshness. For dynamic annotated objects, Tesla will annotate the annotation units of the object with temporality, thereby calculating its motion trajectory and various parameters, and giving the response strategy of the vehicle.
It is not difficult to see that Tesla's auto-label logic is actually a reproduction of the human mind, and this logic is based on Musk's deep thinking on the first principles.
In the human world, human beings can quickly derive rough estimates of the location, size, and quality of things they see based on past experience, and then verify them through measurements and correct their ability to evaluate with the naked eye.
Tesla, on the other hand, quickly gives a three-dimensional point estimate of the image information obtained by visual perception according to the algorithm, and then feeds it back to the image for verification. As a result, tesla can efficiently run the annotation model with the terminal as a unit, and the cloud can quickly integrate and optimize massive data, which is greatly improved compared with manual annotation.
Like the labeling logic, Tesla also brought first principles to the field of training, but unlike "practice to make true knowledge", Tesla hopes to reduce training costs through "meditation".
As mentioned earlier, the real scene contains many variables, some of which are not only unattainable, but also need to repeat the training many times to give the best training results in the mode of relying on the training of the real team.
As a result, Tesla has built a set of simulated worlds that can imitate the perspective of the perception system, which is somewhat similar to human racing games, but it can accurately imitate a variety of complex scenes and variables, and repeated multiple trainings until the algorithm model is polished and matured.
In the simulation system, Tesla can not only repeatedly train the algorithm model under a variety of scenarios and variables, but also restore some scenes of failed driving data and analyze the reasons for their failure through re-disk to further optimize the algorithm. As a result, Tesla's autopilot technology has been rapidly iterated and has become the most successful autopilot company in the current commercialization.
Data-driven, labeling and simulation training is fast and cost-effective
Gu Weihao, CEO of Mo mo zhi xing, said in 2021 HAOMO AI DAY that data intelligence is the core of autopilot, and this core also brings a strong driving effect to the labeling ability of mo mo zhi xing.
Like Tesla, Millima Zhixing also introduces automatic annotation capabilities, but unlike Tesla's annotation logic, Millima Zhixing obtains 4D point cloud annotation information by detecting the tracking and motion attributes of the perceived object and adding timing information.
Based on this automatic annotation capability, Millima Zhixing can first distinguish dynamic annotations from static annotations, but if you want to make more accurate judgments on the motion logic of dynamic annotations, you need denser point cloud information.
In this regard, the practice of Zhixing is that it can compress the multi-dimensional and multi-angle point cloud information that has been collected so far, and at the same time increase the density of the existing point cloud, it can also carry out object detection to obtain more accurate point cloud labeling information.
If Tesla's annotation logic is to "guess first and then test" like a person, then the end of the zhixing is to do mathematical problems of "drawing and solving", dense 4D point cloud information can greatly improve the accuracy of the entire autonomous driving world, which is in line with its data is the core idea of automatic driving.
Most importantly, this can reduce the labeling cost of Millima Zhixing by 80% and greatly improve the labeling efficiency.
After the introduction of automatic labeling to achieve the "cost reduction and efficiency increase" of labeling capabilities, The Wisdom Of the End also needs to be optimized for training capabilities, which is called verification capabilities in the data intelligence system MANA.
In the real scene, affected by the performance parameters and installation position of various types of perception hardware, the automatic driving perception system will be affected by various factors in the scene in actual work, such as the appearance of light spots in the camera, lidar is blocked by sediment, etc.
On the other hand, the threat of various extreme scenarios and variables to the generalization of the automatic driving algorithm model still exists, and in order to ensure the maturity of automatic driving technology, Zhixing also needs to verify such scenarios and variables.
In this regard, Zhixing has also built a set of simulation systems that can imitate the perspective of the perception system, and train and verify the algorithm model by reproducing various scenes, so that many verification work that requires at least 1 year in the real scene can be completed in less than 1 second.
Although simulation systems can improve verification efficiency, the problem that comes with it is the requirement for higher computing power performance.
According to the information of the 2022 HAOMO AI DAY, Zhixing chose to cooperate with the Alibaba Cloud PAI-EFLOPS team to conduct distributed training of the Swin Transformer model based on the 128 card A100 cluster to improve the training efficiency.
On the other hand, through cooperation with Alibaba, Zhixing has also achieved hybrid precision optimization, operator optimization, and compilation optimization, which reduces the cost of model training by more than 60% and accelerates by more than 96%, so that "fast" and "provincial" can be realized at the same time.
In order to accelerate the evolution of the automatic driving system, in the future, Zhixing will use the super high computing power advantages of cloud computing and supercomputing center to optimize the virtual test more smoothly and realistically to ensure that the verification and training effect is fast and effective.
Defeat magic with magic and deal with AI with AI
In general, Tesla and Zhixing's idea of "reducing costs and increasing efficiency" is slightly the same as that of heroes, that is, "learning to let go", by giving automatic driving more "intelligence", so that it can learn to run on its own, thereby liberating manpower and achieving cost reduction and efficiency.
As the saying goes, only magic can be defeated by magic, so the only way to solve the AI problem of automatic driving is to use AI technology itself.
Specifically, first of all, the introduction of automatic labeling capabilities to achieve the improvement of labeling speed and labor cost reduction under massive data, and through the way of manual + automatic labeling collaboration, give play to their respective advantages to further ensure the efficiency and quality of labeling.
In terms of training, both have created a set of simulation systems for training and verifying algorithm models for themselves, and further improve the training efficiency and ensure the efficient operation of the simulation system through the computing power and algorithm optimization of the corresponding systems.
Through the upgrading and improvement of labeling and training, Tesla and Zhixing have become the fastest autopilot companies at home and abroad, but in the face of the current urban scenario, automatic driving cannot achieve the premise of complete safety, so when autopilot breaks through L2 to L3, it will take a while.