A byproduct of equipping ADAS or self-driving cars with multiple sensors is that automakers will almost certainly add more subsystems to keep those sensors clean and unobstructed.
The auto industry is aware of this, but few have figured out how to solve the problem. While a blocked sensor has the potential to paralyze a self-driving car (AV), humans are likely to encounter similar problems while driving, but it will only have an impact on ADAS functionality.
In addition, in the case of ADAS, it is possible that simply having multiple types of sensors will solve this problem in the short term. Willard Tu, senior director of Xilinx's automotive business unit, mentioned this issue in an interview.
At the same time, while there may be few self-driving passenger cars in the near future, there will also be some applications (plus a growing number of delivery and cargo vehicles, drones and other self-driving systems), so this problem needs to be addressed early, not delayed.
Whatever the solution, it will add more cost to the entire vehicle. It could be more equipment, it could be redesigning the vehicle to make room for additional subsystems, it could be processing power, but it's definitely going to increase costs.
An extreme view is that the sensor must remain spotless. This view is very prominent among automotive component suppliers who have developed sensor cleaning devices. The other extreme is held by some lidar and radar engineers who see the problem of partially covered sensors as one that AI will eventually handle with ease, as long as there is enough training.
...... Given the need for adequate training. Keep in mind that most companies that test ADAS and AV technologies do most of the actual road test mileage in cities/suburbs like Arizona and California. They know it's just an ideal reality where it's almost every day when the sky is clear and they rarely encounter anything that might obscure the sensors.
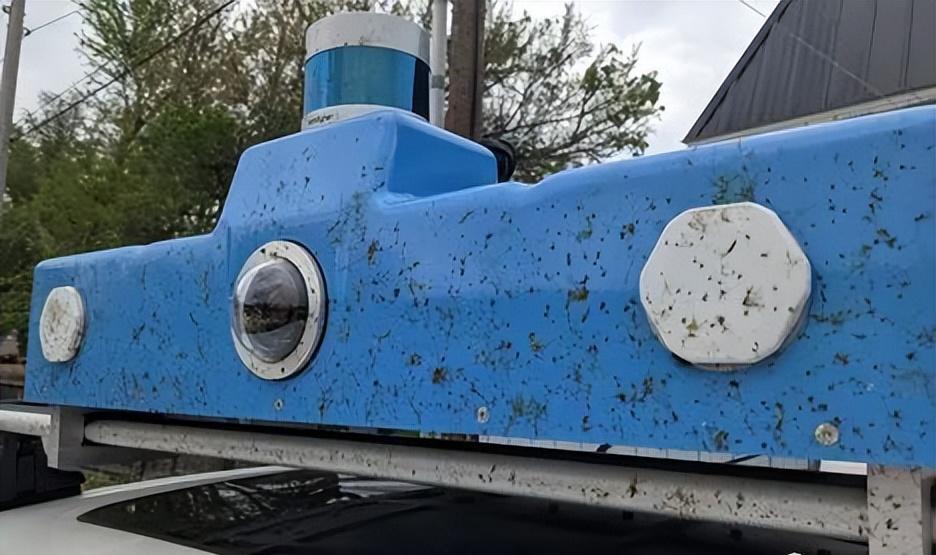
In long-distance tests conducted by VSI Labs in various U.S. states, it was found that the surface of the sensor would be attached to the debris of many flying insects and would be very difficult to clean.
The obvious cleaning mechanism is the one that automakers have used for decades to keep the windshield clear: the cleaning fluid is dispensed through the sprinkler, and then the cleaning fluid and dust are removed with a wiper. For example, Waymo uses this approach for its sensors.
But as the number of sensors (or groups of sensors) that must be cleaned increases, it becomes increasingly unrealistic to add wipers.
However, resorting to a simple rinse mechanism without a wiper is problematic, as the beads of the cleaning solution can be as obstructive as the dirt being washed off, especially for optical cameras. Some auto parts suppliers, including dlhBowles and Kendrion, have argued that the cleaning mechanism will have to be combined with liquid sprayers and air jets to dry parts.
One suggestion is to design the body in such a way that the airflow naturally removes dust and dirt from the sensor kit most of the time. Of course, "most of the time" means that there will be times when the sensor will inevitably get dirty. And then what? However, another option being explored is the use of ultrasonic cleaning.
Automakers are still evaluating all of these possible solutions, even as they add more sensors to their vehicles every year.
The typical reason for equipping a vehicle with different sensor combinations is that multiple different sensor data streams complement each other to provide better ADAS and AV performance than any single sensor mode.
But there is also a reason that it is important to add different kinds of sensors. When the performance of one sensor is degraded or even completely stopped by rain, mud, splashing bugs, or other environmental waste, the key is to have a backup input data stream from a different sensor that may be less impaired or even unhindered.
That's part of the reason Tesla said in May that it would abandon radar and rely entirely on cameras to get so much attention. Anyone who thinks for a few minutes knows that it is inevitable that a Tesla vehicle will be splashed with mud and fail. That's why some auto experts believe Tesla's decision may be a temporary situation.
Xilinx's Willard Tu describes how different sensors perform under different types of obstacles (Xilinx's FPGAs are being used by some companies to process sensor data).
For example, he explained, the advantage of radar over vision systems is that radar can pass through rain and fog, even though these conditions may limit the range of a radar system.
At the same time, the mud is unique. Radar can easily penetrate dry mud. But what about wet mud? Water is electrically conductive, and it can distort signals.
The graph below shows how multiple cameras, radar, and lidar can complement each other. Some automakers are evaluating all three of these sensors, and many are trying to determine if they can use two of them (and which combination). With the exception of Tesla, few companies consider using a single type of sensor.
Willard believes that two types should be used. Both lidar and radar are good at ranging. Cameras are good at color, and lidar and radar can't do that. Lidar, on the other hand, can sometimes read signs if there are enough edges on the text. There are always trade-offs in terms of cost and performance.
Willard went on to say that most automakers only want one camera because it's cheap and easy. Then maybe it's lidar or radar.
But deciding which sensor or combination to use is much more complicated, Willard explains.
The more sensor data a vehicle collects, the more processing power it needs. But automakers have a lot of leeway in balancing the amount of data they collect, the processing power they need, and the complexity of their AI.
Willard says good AI is key. He shared a picture of a point cloud read from an actual sensor, and one of Xilinx's customers assured Willard that it was used to correctly identify everything in the field of view. It's so sparse that we can't even guess what might be in the scene. The point is that sophisticated AI can do more than expected with seemingly minimal data.
That's what they did when lidar and radar engineers said AI could be used to compensate for partially occluded sensors. Similar to the way AI can function with relatively little data, AI can be used to solve the problem of getting less data than normal, as long as there is enough training.
Search public number: Zhiche Tribe
Get more industry profiles