【Global Vision】
Written by John McQuaid Translated by Shi Yi
1. Monitor the society everywhere
In Liverpool, England, a somewhat tedious meeting on government procurement is underway. It was February 2020, and the exhibition hall of the conference was lined with various exhibits. Attendees walk around these exhibits, sometimes stopping in front of some of the exhibits and other times bypassing them. At the same time, they are also closely "monitored". Across the floor are placed 24 inconspicuous cameras for tracking everyone's activities. The change in human expression is produced by the movement of facial muscles, so when participants face different exhibits, the facial muscles will contract to varying degrees. Although these changes are subtle, the 24 cameras will shoot at a rate of 5 to 10 frames per second. The captured photos are then transmitted to a computer network, where AI algorithms are used to assess each person's gender and age, and their facial expressions are analyzed. Eventually, the system finds signals for "happiness" and "commitment."
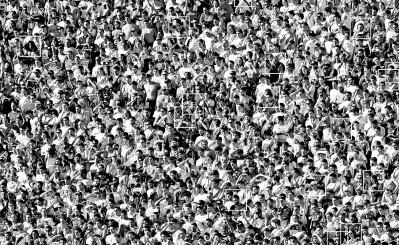
Courtesy of Global Science magazine
Although some time has passed since the Liverpool meeting, Panos Mutafis is still excited about the results of the "surveillance". Mutafis is the CEO of a company called Zenus. The company, based in Austin, Texas, provided AI technology for facial expression analysis at the conference. "Few commercial AI systems I've seen have achieved this level of accuracy." He said this to me on a video call. He also showed me a picture of the crowd, with some faces in the crowd framed out in boxes. In order for the AI system to learn to recognize human emotions, Zenus engineers "trained" the system. They selected a huge data set of facial expressions, each of which is also marked with corresponding inner feelings, and used this data set to train the AI system's ability to recognize emotions. To validate the training AI system's ability to recognize emotions, Zenus engineers tried several approaches. These include field testing, which involves photographing a person's face with a video camera while they are saying how they feel in the moment. "This AI system can recognize people's emotions in a variety of environments, such as indoors, when people are wearing masks, or when there is no light, or outdoors, when people are wearing hats and sunglasses," Mutafis said. ”
2, can recognize emotions of the machine
Recently, an emerging technology called emotion AI or affectivecomputing combines cameras with other devices based on AI programs to capture clues such as facial expressions, body language, intonation, and more. Among them, the AI system developed by Zenus is an example of this technology. It is worth mentioning that the purpose of emotional AI is not only to identify and identify facial expressions, but more importantly, to reveal information that could not be detected by previous technologies, such as the inner feelings, motivations and attitudes of people in photos. Jay Stanley, who wrote a 2019 report titled "Dawn of Robotic Surveillance," said: "Cameras are getting smarter these days. They are waking up – no longer just silently recording human activities, but now they can analyze the information they record. ”
It is conceivable that emotional AI has become a popular market research tool, but in addition, emotional AI has also been applied to riskier areas. For example, there are AI systems that read out clues related to feelings, personality, and intentions, and people are planning or have used these systems to detect threats at border checkpoints, assess job seekers' abilities, monitor for behavior that disrupts classes or dozing off, and identify signs of aggressive driving behavior. Major automakers plan to apply this technology to the cars of the future. Technology companies combine facial recognition technology to provide cloud-based emotional AI services such as Amazon, Microsoft and Google. In addition, dozens of startups have launched apps that can help businesses recruit. In South Korea, the practice of using AI to recruit has become so common that career trainers often let their clients practice how to pass ai interviews.
To identify emotions and behaviors, AI systems need to use multiple types of data. In addition to facial expressions, intonation, and body language, they can also obtain the emotions and attitudes contained therein by analyzing the content of the spoken or written language. There are also apps that collect data, not to explore emotions, but to get information about emotions. For example, what kind of personality the person has, whether they care about the content of the application, and whether they pose a potential threat to society.
But critics warn that the potential dangers of emotional AI may not be within the control of AI itself. That's because engineers may be training AI with datasets of racial, ethnic, and gender biases that in turn affect the results of the algorithm.
The scientific principles behind emotional AI are also controversial. This goes back half a century, when psychologists Paul Eckerman and Wallace Friesen, based on research, mapped a set of facial expressions to basic emotions that they considered to be the universal language of emotion. Six of the basic emotions include anger, disgust, fear, happiness, sadness, and surprise, and subsequently, Ekman found that contempt was most likely the seventh basic emotion. But now, Ekman and Frieson's views are highly controversial. That's because scientists have found that facial expressions can have significant cultural and individual differences. Many researchers say that at least for now, when analyzing the facial expressions of different individuals, the algorithm cannot correctly identify subtle differences in expressions with a set of rules, because sometimes the expressions of different individuals do not correspond to typical inner feelings. Ekman made an important contribution to the development of early emotion recognition technology, and it is worth mentioning that he now believes that this technology poses a serious threat to privacy and should be strictly regulated.
Emotional AI isn't inherently bad. Experts say that if machines can learn to reliably interpret emotions and behaviors, emotional AI will show great potential in areas such as robotics, health care and cars. But now, the field is almost a "scuffle", and perhaps eventually an unproven technology will dominate and become ubiquitous. However, unproven technology can be harmful to society, and we may be caught off guard by then.
3. Use AI to recruit
In 2018, Mark Gray, then vice president of human and commercial operations at Airtame, who developed devices with screen sharing capabilities, wanted to find ways to improve the company's hiring process, including improving the efficiency of hiring. Partly, because despite its modest size, which employs about 100 people, sometimes the company receives hundreds of resumes applying for marketing or design positions. On the other hand, it is because of the subjectivity of hiring decisions. "There have been many times when I've felt like someone subconsciously says, 'Oh, I like this guy a lot,' instead of 'this guy is very capable.'" In fact, the world of hiring is full of intangibles, so I wanted to figure out how I could incorporate tangible considerations into hiring. Gray explained.
The United States Aitamer has a contract with Theoreio company in Munich, Germany, in which Retiro has developed an AI system that can be used in video interviews. The video interview process is fast, and candidates only need to record a 60-second video to answer 2 or 3 questions. Subsequently, algorithms are used to analyze the candidate's facial expressions and voices, as well as the content of their answers. Then, based on the "Big Five" personality model (OCEAN, a personality structure model commonly used in psychology), a profile based on 5 personality traits was generated for each candidate. These 5 personality traits are openness, conscientiousness, extraversion, agreeableness, and neuroticism. By comparing the candidate's profile and job description, the system will sort the candidates by degree of matching, and the recruiter will finally get a ranking list of candidates.
In fact, similar software has begun to change the way business decisions are made and how organizations interact with people. It reshaped Aitamer's hiring process, allowing them to quickly select more suitable candidates. Gray says that's because the generated archives are useful. He shared a chart showing the relationship between job performance and 5 personality trait scores among several recently recruited salespeople, with employees with higher scores in terms of conscientiousness, agreeability and openness performing best.
Machines that can understand human emotions have long been the subject of science fiction. But in computer science and engineering, for a long time, human emotions were an unfamiliar concept. In the 1990s, "it was a taboo topic and not popular. Rosalind Picard of the Massachusetts Institute of Technology (MIT) said.
Picard and other researchers have developed tools that automatically read and respond to biometric information. Among them, biometric information covers a range from facial expressions to blood flow and can be used to indicate emotional states. However, the surge in emotional AI applications today dates back to the beginning of 2010, when deep learning began to be widely used. Deep learning is a powerful form of machine learning based on artificial neural networks, where the prototype of an artificial neural network is a biological neural network. Deep learning has improved the power and accuracy of AI algorithms, automating tasks that were previously reliable only to humans, such as driving, facial recognition, and medical image analysis.
4. Ai's algorithmic bias
However, such AI systems are far from perfect, and emotional AI handles an extremely difficult task. Algorithms are supposed to reflect the truth about the world, such as they should recognize apples as apples, not peaches. "Learning" in machine learning is the process of iteratively comparing raw and training data. Among them, the raw data is usually an image, but also includes video, audio and other data, but these raw data do not have unique features, while the training data is labeled with features related to intelligent tasks. This is how AI systems learn to extract potential commonalities, such as extracting "apple sense" from images of apples, so that apples can be identified from arbitrary images.
But if the task of an AI system is to identify hard-to-define traits like personality or emotion, it's harder to get the truth. For example, what exactly does "happy" or "neurotic" look like? Emotional AI algorithms don't intuitively know emotions, personalities, or intentions; instead, they learn to mimic human judgments about other people through training. Among them, engineers collect data through crowdsourcing to build a dataset for training AI. Critics argue that the process of training AI introduces too many subjective variables. Kate Crawford of the University of Southern California in the United States said: "There is a huge gap between the judgments made by these algorithms and a person's real thoughts or emotional state. Therefore, 'making machines perceive emotions like people' is both a huge leap forward in AI-related technologies and a risky step. ”
The process by which AI systems identify traits such as emotions is complex, and there are potential flaws at every step. The need for large amounts of data in deep learning is notorious, so emotional AI also needs huge data sets. But these datasets are often accompanied by judgments by thousands, if not billions, of individuals. This could cause algorithms to inadvertently "learn" the systemic biases of all data collectors. Algorithms integrate these systemic biases to form "algorithmic biases," which can come from demographic biases in training datasets and unconscious attitudes of data labelers.
Even identifying a smile is far from a simple task. In 2020, in a study by the GESIS-Leibniz Institute for Social Sciences in Germany, Carsten Schweimer and colleagues analyzed photos of members of Congress using Cloud-based emotion recognition apps from Amazon, Microsoft, and Google. Looking at it with the naked eye, the researchers determined that 86 percent of the men and 91 percent of the women in the photo were smiling, however, the app's results were more likely to think that women were smiling. Google Cloud Vision, for example, labels more than 90 percent of women's photos with a "smile," compared to less than 25 percent of men's photos. The researchers speculate that the training dataset may be gender biased. Moreover, "blur" is common when researchers make judgments about these images, but this is often ignored by machines. "The meaning of many facial expressions is not so clear. Is that really a smile? Does a smirk count as a smile? What if the person in the photo shows their teeth but doesn't look happy? They added.
In fact, most deep learning-based facial recognition systems have been widely criticized for their bias.
Now, many companies are emphasizing that they are aware of and are trying to address the problem of "bias." Christopher Hornberger, co-founder of The German company Retorio, says they are already taking steps to eliminate biases that bias personality judgments, such as demographic and cultural biases. But there is currently a lack of regulatory mechanisms in the industry. So, for the most part, we have to believe the company's one-sided words, although it is difficult for us to verify the robustness and fairness of the company's proprietary data sets. HireVue, a company dedicated to video interviews, uses algorithms to analyze candidates' speech content and tone to assist in hiring decisions. At the same time, the company also asks external auditors to check the algorithm for bias, but companies that do so are rare.
5. Controversy over scientific principles
Iphioma Arjunwa of the University of North Carolina in the United States said that emotional AI has not only raised concerns about algorithmic bias, but the scientific principles behind it have also begun to be strongly opposed by scientists. Emotional AI follows the scientific view that each person's outward performance can match the interpretable inner emotions. Moreover, this view dates back more than 50 years. At the time, Exman and Frieson were doing fieldwork in Papua New Guinea. They found the indigenous Fore people in the southeastern highlands here and studied the way the Fore people recognized and understood facial expressions. The researchers selected several sets of emojis that could express 6 basic emotions and showed the images to the volunteers. The results found that the response of the Fore people was almost exactly the same as that of experimental volunteers in other countries, such as Japan, Brazil and the United States. As a result, the researchers believe they successfully demonstrated that facial expressions are a universal human language of emotion.
Ekman and Frieson also painted a "map" of thousands of facial muscle movements, which were analyzed to determine the correspondence between facial muscle movements and expressions, resulting in a facial behavior coding system (FACS). It is worth mentioning that "maps" and FACS together form the theoretical cornerstone of emotional AI, which has now been integrated into many AI applications.
Scientists have disputed Ekman's theories, arguing that they are flawed. For example, in 2012, a study published in the Proceedings of the National Academy of Sciences (PNAS) showed that facial expressions vary greatly across cultures. In 2019, lisa Feldman Barrett, a psychologist at Northeastern University, and colleagues analyzed more than 1,000 scientific papers on facial expressions and found that while the idea that the appearance reflects inner feelings has expanded to multiple fields, from technology to law, there is little conclusive evidence to justify this view.
Barrett says basic emotions are a broad and stereotypical way of categorizing. Because every moment, facial expressions reflect complex inner states—a smile may be masking pain or conveying sympathy. She believes that the current AI system can not consistently and reliably distinguish the inner state of the person, this is because the training data of the AI system is essentially a dataset composed of labeled stereotypes. "It's about measuring certain characteristics first and then speculating about their psychological significance, but these are two very different things. The current much-hyped emotion recognition technology often confuses these two things. Barrett said.
One of the reasons for the problem, Crawford says, is that tech startups don't understand the scientific debate in other areas, and that these companies are drawn to the beauty of FACS-like simplicity. "Why is Ekman's theory favored by the field of machine learning?" Crawford asked. "This is because Ekman's theory fits well with the characteristics of machine learning. If the number of expressions is limited in a theory, and the number of emotions that an expression may correspond to is strictly controlled, this theory can be used to build machine learning models. In fact, in addition to Ekman's findings and ocean's personality trait model, companies developing emotional AI have adopted other theoretical systems. One of them is the "wheel of emotions" proposed by the late psychologist Robert Pluchke. All of these theories translate the complexity of human emotions into simple and straightforward formulas.
Still, the researchers believe that after understanding the limitations of emotional apps, we can make improvements based on them to make them work. Ayana Howard is dean of the Ohio State University School of Engineering and a roboticist. She used an improved version of Microsoft's facial expression recognition software to have robots teach children with autism to learn social behaviors. For example, if a bot detects an "angry" expression on the interlocutor, it adjusts its movements to calm the situation. Typical facial expressions may not always mean exactly the same emotions, Howard says, but they're still useful. "Indeed, we are all unique. But in fact, the difference between people is not so big. Therefore, for emotions in a broad sense, the judgment of these emotional AI may not always be correct, but it is not just a coincidence. They are more likely to be correct than random. She said.
Overall, algorithms that scan and aggregate the facial reactions of many people will be more accurate, such as those used to interpret crowds. That's because statistically, Barrett says, as the size of the population increases, "impossible" becomes "possible," giving it a "greater probability than a random correctness." But assessing individuals is risky, because anything less than 100% accurate creates discrimination against certain individuals.
Many computer vision experts now prefer an agnostic attitude toward facial expressions, meaning that they cannot get exact results by analyzing facial expressions. And more and more companies say they don't directly use facial expressions to describe emotions or inner states. Jonathan Gracchi of the University of Southern California in the United States said: "As this field has evolved, there is a growing recognition that many expressions have nothing to do with emotion. Expressions are like words with meaning in a conversation, and neither expressions nor words can directly convey the feelings of the moment. ”
6. Potential privacy risks
As more and more technology tries to describe emotions, personality traits, and behaviors, and tries to bring related technologies to market, our lives are being more monitored. Twenty years after tech companies mined personal data from online behavior, a new, more private field is poised to do something similar: collect face and body information and the signals they convey. VSBLTY Canada sells smart cameras and software for scanning people, which analyze consumer demographics and reactions to products for retailers. In December 2020, VSBLTY announced a partnership with Mexican beer maker Modro Group to deploy in-store cameras to capture data in 50,000 Modro Rama convenience stores owned by the Modero Group, as well as community hotels in Mexico and other Latin American countries, by 2027.
This raises a fundamental legal and social question: Is the data from your face and body yours yours? If you separate individual identity from these data, in most parts of the world, the answer is no. Jennifer Budd, a professor at the University of Cincinnati Law School in the United States who has studied the issue, said: "If you want to know the information of some people in public places, scanning them to identify emotions doesn't seem to be restrictive. ”
Most emotional AI companies that collect data in public places say the information they collect is anonymous, so the public doesn't have to worry about it. Zenus' Mutafis noted that Zenus' app doesn't upload images of real faces captured by cameras, only metadata about emotion and location. During surveillance, they display signs on the screens of the venue to inform the people in the meeting. Mutafis said: "It is actually a very good practice to inform the person being collected when collecting information. Because as a company, we should put up signs in the areas where we are monitoring behavior, indicating that this place is being monitored. "But the diversity of applications means there is no uniform standard. And once such routine surveillance becomes a political and policy question, there is far from a definitive answer to whether the general public and politicians will accept it.
Exman has previously worked with Emotient and Apple on emotional AI, but now he warns that emotional AI poses a threat to privacy and says the company has a legal obligation to obtain the consent of everyone scanned. "Unfortunately, this is a technology that can be used without people's knowledge. Emotional AI is being used on people, but not to make them happier. The technology will also allow people to buy products that would not otherwise be purchased. However, this may be the most benign non-benign use of emotional AI. Ekman added.
In addition, emotional AI has also invaded private spaces, which store richer behavioral data. Amazon's Alexa (Amazon's emotional AI system) analyzes the user's tone of voice, looks for signs of frustration and improves the algorithm accordingly. By 2023, some automakers will roll out AI-based in-vehicle systems that will generate vast amounts of data about the behavior of drivers and passengers. Automakers will use this data, which may be anonymized, to improve system responsiveness and interior design. Modar Allavi, CEO of Emotional AI Company Eyeris, said users may have the option to activate different levels of functionality in the system, so if the user doesn't use certain features, the system won't collect data from those places.
Alex Martinez is a computer vision scientist at Ohio State University and Amazon. In 2019, he co-authored a paper with Barrett that criticized the connection between facial expressions and emotions. He would always show a photograph of a man's face twisted and it looked as if he were in an emotion mixed with anger and fear. He then showed the whole picture, which turned out to be a footballer who was ecstatic after scoring the goal. He points out that signals such as facial expressions and gestures are not only products of the body and brain, but also related to the context in which things are happening, as well as to what is happening in a person's surrounding environment. The biggest challenge facing emotional AI to date is how to interpret ambiguous situations. "Unless I know what football is, I will never be able to understand what's going on in the pictures. So that knowledge is the foundation, but no AI system can do a good job of interpreting contexts right now. Martinez explained.
Martinez said emotional AI will become more effective if the scope of the task is narrowed, the environment is simple, and diverse biometric information is collected. However, the future of emotional AI, which integrates diverse biometric information, may only be a more powerful and more invasive technology that society is not ready to meet.
(This edition is courtesy of Global Science)
Guangming Daily (2022.01.27. 14th edition)
Source: Guangming Network - Guangming Daily